线性回归的从零开始实现
- 一、线性回归算法设计
-
- 1.导入相关包
- 2.生成人造数据
- 3.使用小批量梯度下降
- 4.初始化参数
- 5.进行预测
- 6.计算损失
- 7.更新参数
- 8.建立模型
- 二、进行试验
-
一、线性回归算法设计
1.导入相关包
import torch
import matplotlib.pyplot as plt
2.生成人造数据
def synthetic_data(w, b, num_examples):
x = torch.normal(0, 1, (num_examples, len(w)))
y = torch.matmul(x, w) + b
y += torch.normal(0, 0.01, y.shape)
return x, y.reshape((-1, 1))
3.使用小批量梯度下降
def random_mini_batch(x, y, batch_size):
m = x.shape[0]
sort = list(np.arange(m))
np.random.shuffle(sort)
random_x = x[sort]
random_y = y[sort]
batchs = []
for i in range(x.shape[0] // batch_size):
batchs.append((random_x[i * batch_size:(i + 1) * batch_size], random_y[i * batch_size:(i + 1) * batch_size]))
if x.shape[0] % batch_size != 0:
batchs.append(
(random_x[(x.shape[0] // batch_size) * batch_size:], random_y[(x.shape[0] // batch_size) * batch_size:]))
return batchs
4.初始化参数
def init_parameters(feature_num):
parameters = {}
parameters['w'] = torch.normal(0, 0.01, size=(feature_num, 1), requires_grad=True)
parameters['b'] = torch.zeros(1, requires_grad=True)
return parameters
5.进行预测
def linreg(x, parameters):
w = parameters['w']
b = parameters['b']
return torch.matmul(x, w) + b
6.计算损失
def compute_cost(y_hat, y):
m = y.shape[0]
return (1 / (2 * m)) * torch.sum(torch.square(y_hat - y))
7.更新参数
def update_parameters(parameters, learning_rate):
with torch.no_grad():
for key in parameters.keys():
parameters[key] -= learning_rate * parameters[key].grad
parameters[key].grad.zero_()
8.建立模型
def model(x, y, num_epoches=10, batch_size=3, learning_rate=0.001):
m = x.shape[0]
n = x.shape[1]
parameters = init_parameters(n)
batchs = random_mini_batch(x, y, batch_size)
costs = []
for i in range(num_epoches):
for batch in batchs:
mini_batch_x, mini_batch_y = batch
y_hat = linreg(mini_batch_x, parameters)
cost = compute_cost(y_hat, mini_batch_y)
costs.append(cost)
cost.backward()
update_parameters(parameters, learning_rate)
return parameters,costs
二、进行试验
1.生成人造数据
true_w = torch.tensor([2, -3.])
true_b = 4.0
x, y = synthetic_data(true_w, true_b, 1000)
2.建立模型
parameters,costs = model(x, y, num_epoches=3, batch_size=10, learning_rate=0.03)
3.显示结果
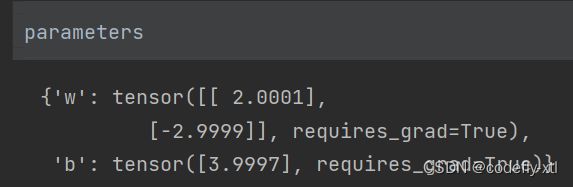