上期链接
引言
- attention 总结的初版主要介绍了注意力机制的理论概念,本文主要作为其补充,补充个人理解的一个加深以及相关变体的一些代码实现。
- 以下为个人的一些比较疑惑的地方以及个人理解,仅供参考
实际问题中 key,value 到底是什么?
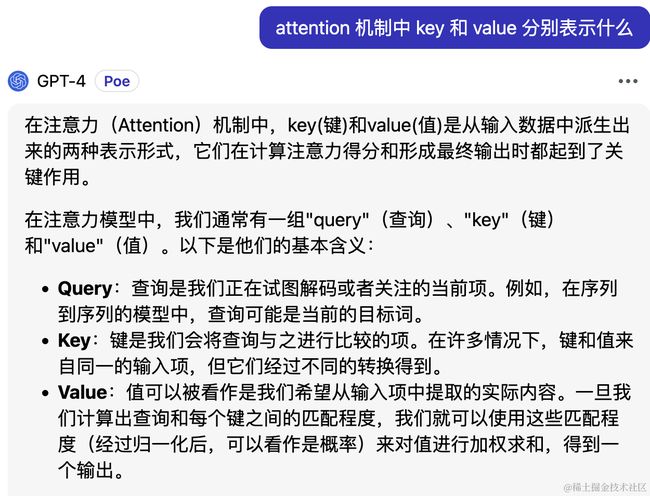
- 参考1
- 参考2
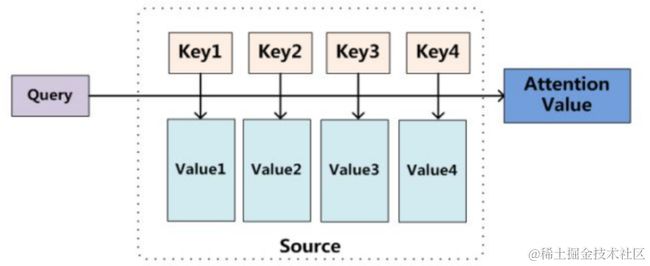
- 个人理解:key 和 value 的值其实来自于同一输入,某种程度上说是等价的,只是不同转换方法得到的一个输出
- 计算 attention 值的过程:首先要计算 q 和 k 的相关性,相关性的值要经过 softmax 函数类似的归一化操作,来表示不同的 key 对 query 的重要程度,再使用归一化后的值乘以对应的 value,个人认为是表示对实际的输入内容进行加权,加权求和后的输出代替 query 参与后面的运算
- 点积计算,结果越大其实就是向量乘积越大表示这俩向量越相似
self-attention
- 自注意力机制个人简介理解:相对于上面介绍的传统的 attention,q,k,v 三个角色是指向不同的词语,自注意力机制关注的就是输入序列之间的关系,即每个词语都有自己的 k,q,v,这样不仅包含了词语本身的信息,又考虑了上下文的信息
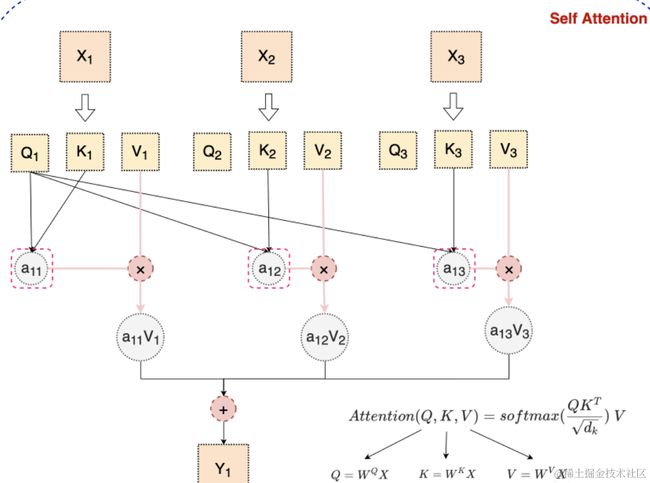
- 上图中每个输入的 Xi 都有各自的 q,k,v,这些是通过各自的输入乘以对应的权重矩阵 Wq,Wk,Wv得到,这三个权重矩阵对所有的输入元素是共享的,而且是可学习的;(缩放点积公式就是上图中Attentiion(Q,K,V))
- 这个权重矩阵可学习,意味着其实初始矩阵可以自行设置(个人理解,感觉有点问题的,此处作为一个 flag)
- 如上图所示每个 k 和 q 都会计算相似度,最终家加权求和
- 注意,此时考虑上下文信息的时候,x1和x2的关系与 x2 和 x1 的关系不一样,也就是顺序不同关系也不同,比如小明喜欢小红,但是小红不喜欢小明,那么计算q小明k小红的分数要远大于q小红k小明的分数
- 以下为上面参考链接中的例子
- dk 是数据embedding 的维度,除以更号它是为了缩小点积的范围,确保 softmax 的梯度稳定性,具体详情可见参考资料
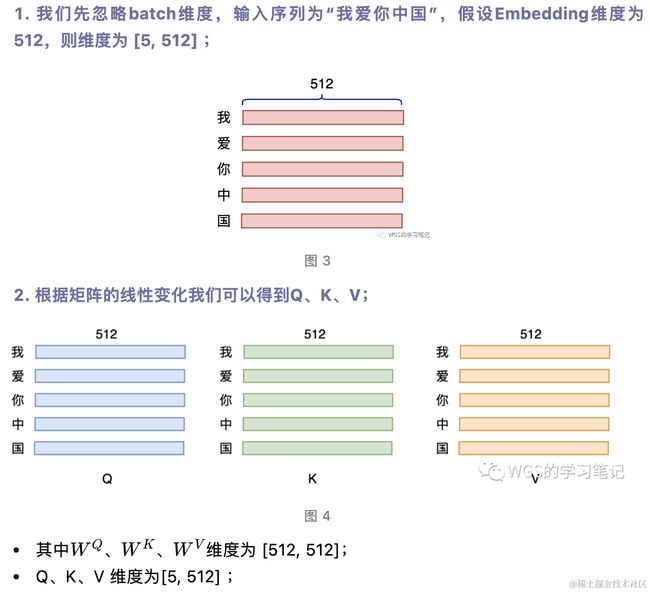
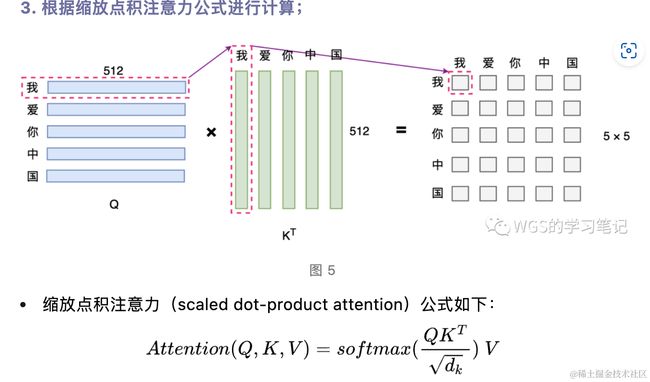
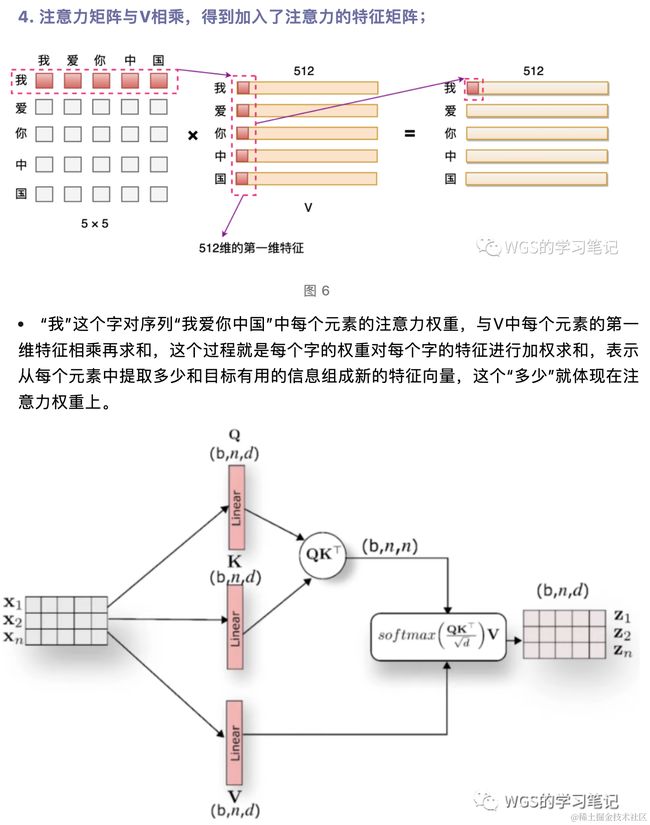
- 此处补充一个自注意力中 k,q,v 的设计由来(仅供参考)
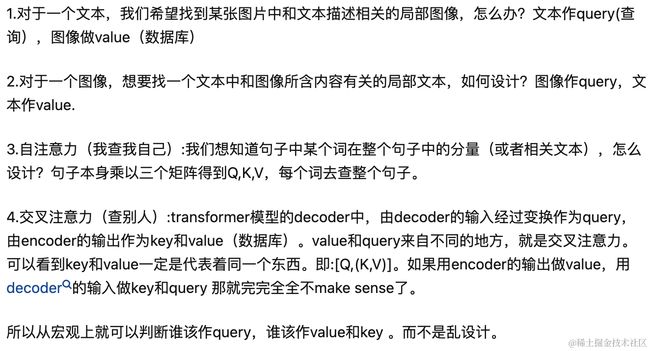
多头注意力机制
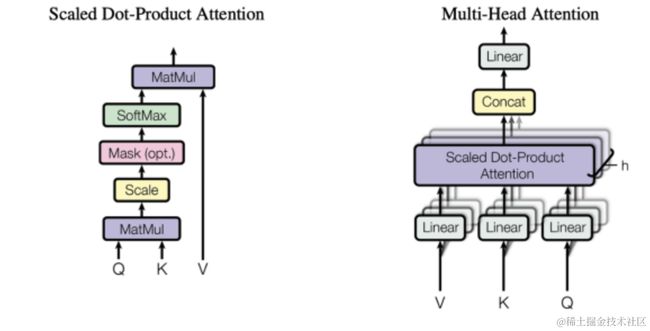
- 个人理解,多头注意力机制就是多个自注意力机制的堆叠,就像每个自注意力头都取输入数据的不同信息;
- 对每个词语的 q,k,v根据分头的个数进行拆分,每个头部都是一个子空间(注意 embedding 的维度要是分头数的倍数,不然没法平均拆分)
- 对每个头部按照自注意力的计算缩放点积,最后将所有的头部输出拼接在一起得到最终的输出矩阵
- 以下为参考资料中的例子图解
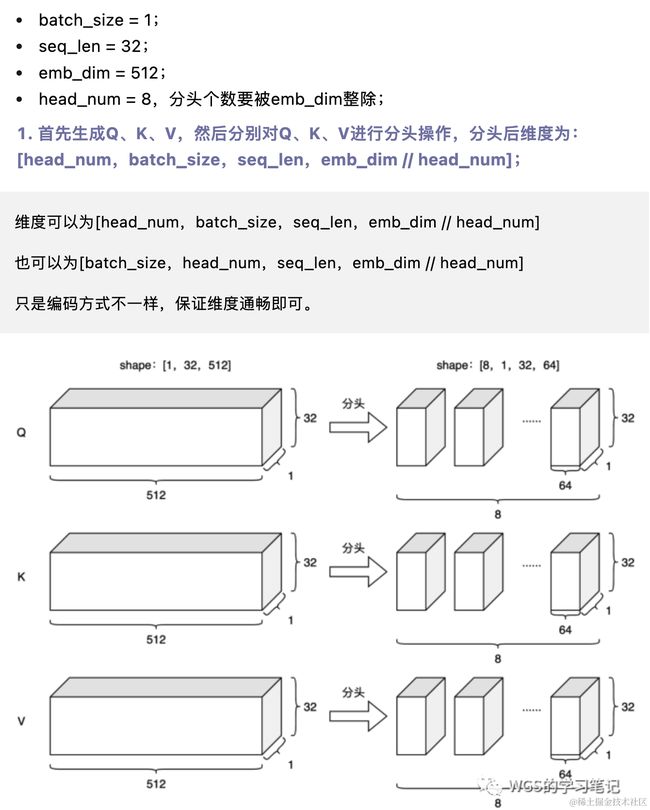
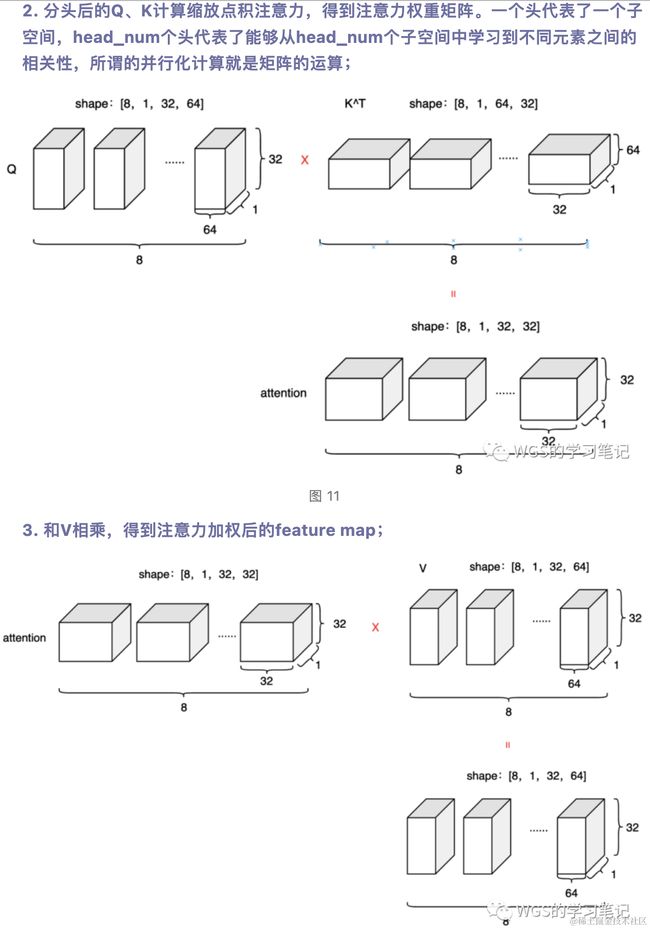
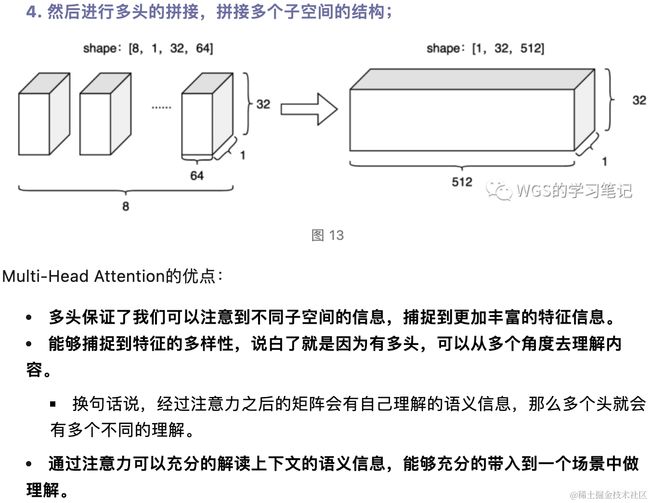
padding mask
- 参考文章中padding mask 部分个人理解是,在处理数据长度的时候,为了保持长度一致会使用 0 来进行填充,但是在计算 attention 值的时候这个位置的值是无用的需要 mask 掉,具体方法应该就是下图所说的内容(个人理解,仅供参考,后续理解加深会修改)
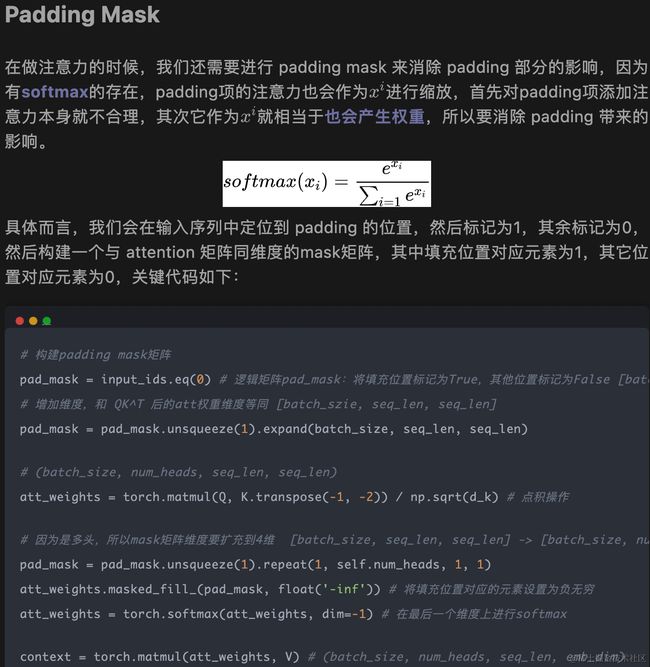
关于多模态 cross-attention 部分这里不做详解,上面的自注意力和多头主要是为了后面学习 transform 铺垫的
- 多模态说的是处理两个不同模态序列之间的关联,比如文本和图片数据进行交互处理,具体应用例如 stable diffusion 的实现(更详细的可以看上面参考链接)。
代码部分()
import torch
import torch.nn as nn
import torch.nn.functional as F
import math
from einops import rearrange, repeat
from torch.nn import MultiheadAttention
class SelfAttention(nn.Module):
def __init__(self, emb_dim):
super(SelfAttention, self).__init__()
self.emb_dim = emb_dim
self.Wq = nn.Linear(emb_dim, emb_dim, bias=False)
self.Wk = nn.Linear(emb_dim, emb_dim, bias=False)
self.Wv = nn.Linear(emb_dim, emb_dim, bias=False)
self.fc = nn.Linear(emb_dim, emb_dim)
def forward(self, x, pad_mask=None):
Q = self.Wq(x)
K = self.Wk(x)
V = self.Wv(x)
print(Q.shape, K.shape, V.shape, K.transpose(1, 2).shape)
att_weights = torch.bmm(Q, K.transpose(1, 2))
print("att_weights1", att_weights)
att_weights = att_weights / math.sqrt(self.emb_dim)
print("att_weights2", att_weights,att_weights.shape)
att_weights = F.softmax(att_weights, dim=-1)
print("att_weights3", att_weights,att_weights.shape)
output = torch.bmm(att_weights, V)
print("output, att_weights的 shape",output.shape, att_weights.shape)
output = self.fc(output)
print("output, att_weights的 shape",output.shape, att_weights.shape)
return output, att_weights
class MultiHeadAttention(nn.Module):
def __init__(self, emb_dim, num_heads, att_dropout=0.0):
super(MultiHeadAttention, self).__init__()
self.emb_dim = emb_dim
self.num_heads = num_heads
self.att_dropout = att_dropout
assert emb_dim % num_heads == 0, "emb_dim must be divisible by num_heads"
self.depth = emb_dim // num_heads
self.Wq = nn.Linear(emb_dim, emb_dim, bias=False)
self.Wk = nn.Linear(emb_dim, emb_dim, bias=False)
self.Wv = nn.Linear(emb_dim, emb_dim, bias=False)
self.fc = nn.Linear(emb_dim, emb_dim)
def forward(self, x, pad_mask=None):
batch_size = x.size(0)
Q = self.Wq(x)
K = self.Wk(x)
V = self.Wv(x)
print(Q.shape, K.shape, V.shape, K.transpose(1, 2).shape)
Q = Q.view(batch_size, -1, self.num_heads, self.depth).transpose(1, 2)
K = K.view(batch_size, -1, self.num_heads, self.depth).transpose(1, 2)
V = V.view(batch_size, -1, self.num_heads, self.depth).transpose(1, 2)
print(Q.shape, K.shape, V.shape, K.transpose(1, 2).shape)
att_weights = torch.matmul(Q, K.transpose(-2, -1))
print("att_weights",att_weights.shape)
att_weights = att_weights / math.sqrt(self.depth)
att_weights = F.softmax(att_weights, dim=-1)
print("att_weights2",att_weights.shape)
output = torch.matmul(att_weights, V)
print("output1",output.shape)
output = output.transpose(1, 2).contiguous().view(batch_size, -1, self.emb_dim)
print("output2",output.shape)
output = self.fc(output)
return output, att_weights
import torch
import torch.nn as nn
import torch.nn.functional as F
import math
from einops import rearrange, repeat
from torch.nn import MultiheadAttention
if __name__ == '__main__':
batch_size = 3
seq_len = 5
emb_dim = 512
vocab_size = 301
input_ids = torch.tensor([[100, 200, 300, 300, 0],
[22, 33, 44, 0, 0],
[66, 55, 66, 30, 0]], dtype=torch.long)
inputs = nn.Embedding(vocab_size, embedding_dim=emb_dim)(input_ids)
self_att = SelfAttention(emb_dim=emb_dim)
self_att(inputs, pad_mask=pad_mask)
multi_att = MultiHeadAttention(emb_dim=emb_dim, num_heads=8)
multi_att(inputs, pad_mask=pad_mask)
- 可见维度变化以及结果,可自行测试上述代码查看具体结果
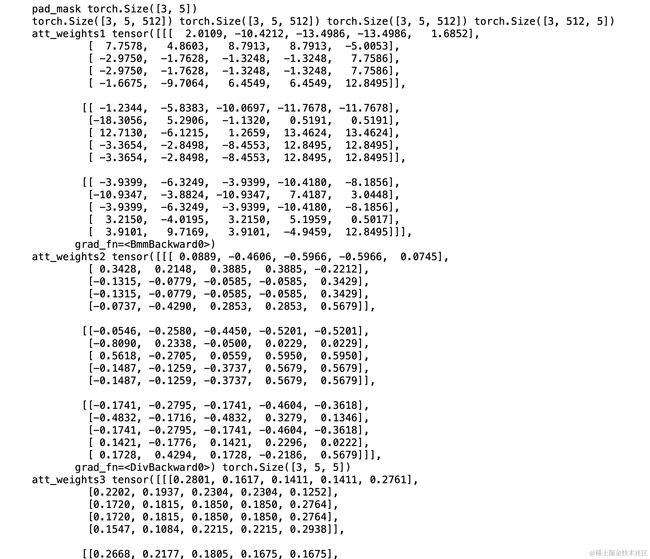
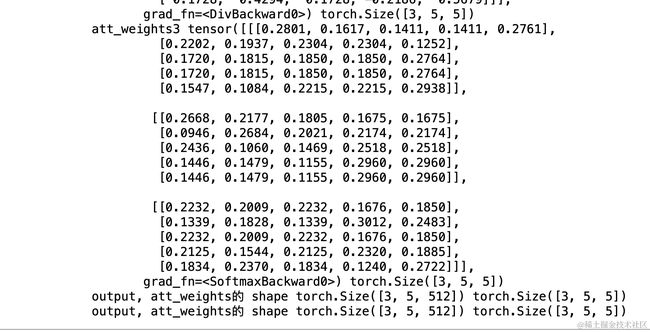
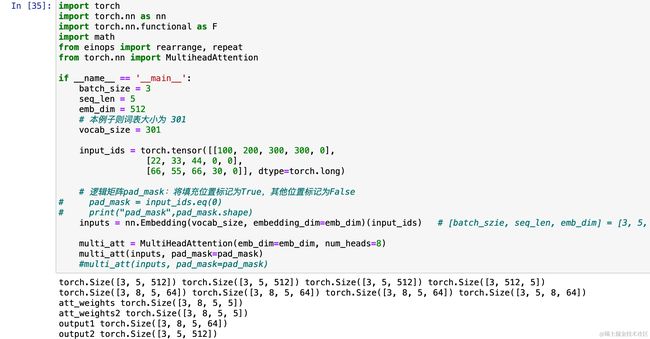
结语
- 本文总结更像是对参考文章的部分一笔带过却不太易于理解的部分做个人理解,通过这样的方式加深个人学习 attention 的印象,想要更准确更深刻的理解可以参考文中参考链接。
- 希望自己能早日工作!