文章目录
- ShuffleNet V1
-
- Channel Shuffle:通道打散
- SuffleNet Unit
- Model Architecture
- 实验结果
- ShuffleNet V2
-
- Guideline 1
- Guideline 2
- Guideline 3
- Guideline 4
- 模型结构
- 代码
论文:ShuffleNet:
ShuffleNet: An Extremely Efficient Convolutional Neural Network for Mobile Devices
论文:ShuffleNet V2:
ShuffleNet V2: Practical Guidelines for Efficient CNN Architecture Design
ShuffleNet V1
Channel Shuffle:通道打散
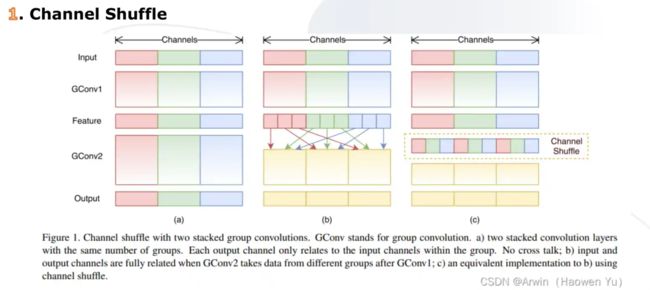
SuffleNet Unit
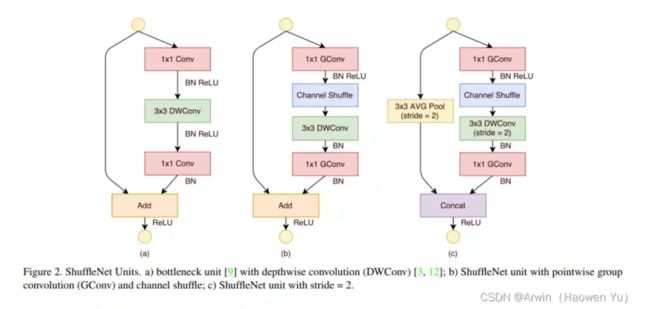
Model Architecture
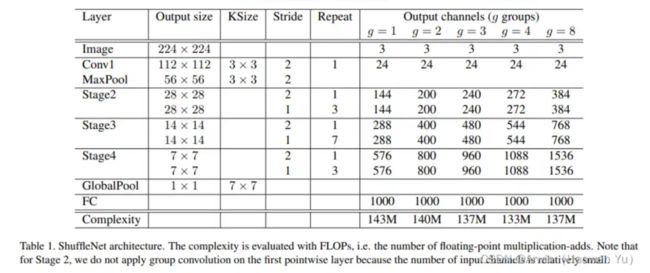
实验结果
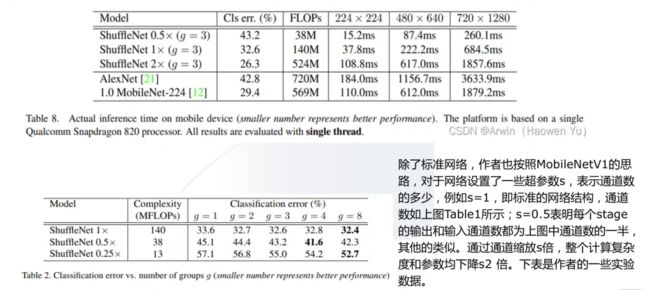
ShuffleNet V2
Guideline 1
- 卷积输入和输出是相同的通道数时,将最小化内存访问成本,每秒处理图片最多
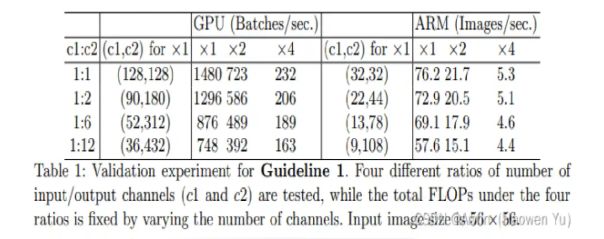
Guideline 2
- 过多的分组卷积会加大内存访问成本,导致训练速度下降,时间上升,但是参数量更低,准确率更高。
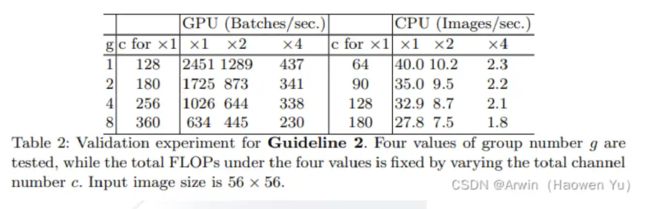
Guideline 3
- 碎片操作减少网络的并行度,碎片操作:将大的卷积分成小卷积操作进行。
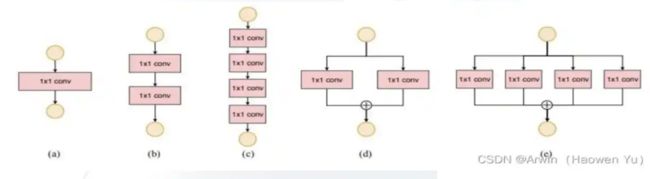
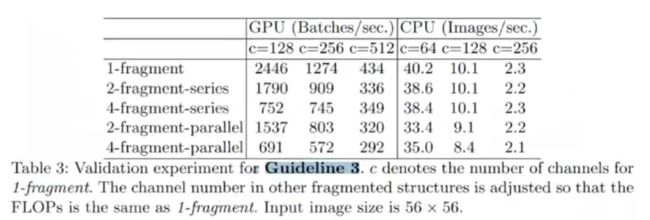
Guideline 4
- 不要忽略元素级操作,元素级指的是 Relu,TensorAdd,BiasAdd等等的矩阵元素操作,可以推测这些操作基本没有被算到 FLOPs 中,但是对内存访问成本(MAC)的影响比较大。
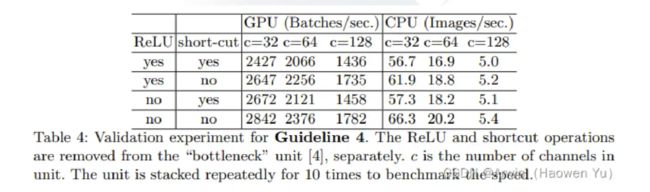
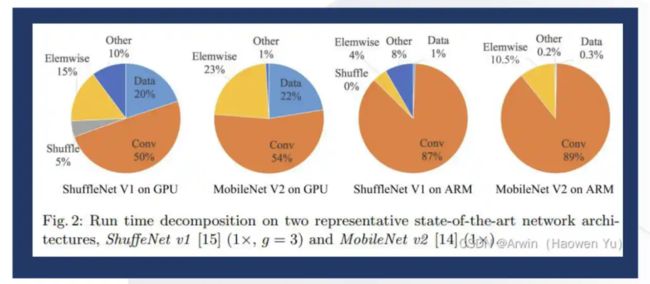
模型结构
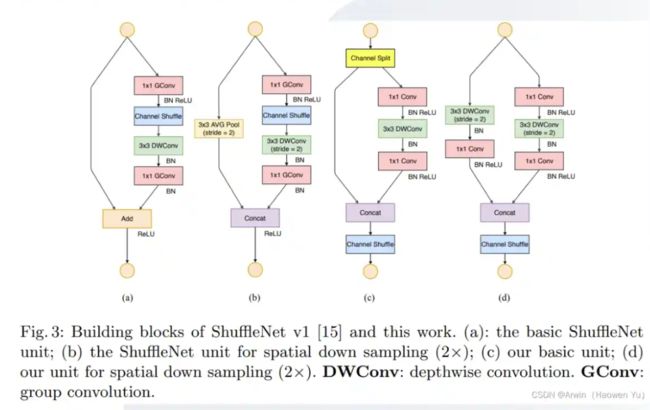
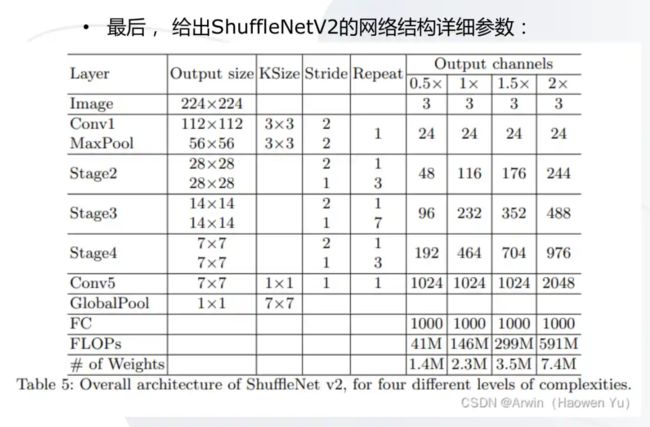
代码
from typing import List, Callable
import torch
from torch import Tensor
import torch.nn as nn
def channel_shuffle(x: Tensor, groups: int):
batch_size, num_channels, height, width = x.size()
channels_per_group = num_channels // groups
x = x.view(batch_size, groups, channels_per_group, height, width)
x = torch.transpose(x, 1, 2).contiguous()
x = x.view(batch_size, -1, height, width)
return x
class InvertedResidual(nn.Module):
def __init__(self, input_c: int, output_c: int, stride: int):
super(InvertedResidual, self).__init__()
if stride not in [1, 2]:
raise ValueError("illegal stride value.")
self.stride = stride
assert output_c % 2 == 0
branch_features = output_c // 2
assert (self.stride != 1) or (input_c == branch_features << 1)
if self.stride == 2:
self.branch1 = nn.Sequential(
self.depthwise_conv(input_c, input_c, kernel_s=3, stride=self.stride, padding=1),
nn.BatchNorm2d(input_c),
nn.Conv2d(input_c, branch_features, kernel_size=1, stride=1, padding=0, bias=False),
nn.BatchNorm2d(branch_features),
nn.ReLU(inplace=True)
)
else:
self.branch1 = nn.Sequential()
self.branch2 = nn.Sequential(
nn.Conv2d(input_c if self.stride > 1 else branch_features, branch_features, kernel_size=1,
stride=1, padding=0, bias=False),
nn.BatchNorm2d(branch_features),
nn.ReLU(inplace=True),
self.depthwise_conv(branch_features, branch_features, kernel_s=3, stride=self.stride, padding=1),
nn.BatchNorm2d(branch_features),
nn.Conv2d(branch_features, branch_features, kernel_size=1, stride=1, padding=0, bias=False),
nn.BatchNorm2d(branch_features),
nn.ReLU(inplace=True)
)
@staticmethod
def depthwise_conv(input_c: int,output_c: int,kernel_s: int,stride: int = 1, padding: int = 0,bias: bool = False):
return nn.Conv2d(in_channels=input_c, out_channels=output_c, kernel_size=kernel_s,
stride=stride, padding=padding, bias=bias, groups=input_c)
def forward(self, x: Tensor):
if self.stride == 1:
x1, x2 = x.chunk(2, dim=1)
out = torch.cat((x1, self.branch2(x2)), dim=1)
else:
out = torch.cat((self.branch1(x), self.branch2(x)), dim=1)
out = channel_shuffle(out, 2)
return out
class ShuffleNetV2(nn.Module):
def __init__(self, stages_repeats: List[int],stages_out_channels: List[int],num_classes: int = 1000,
inverted_residual: Callable[..., nn.Module] = InvertedResidual):
super(ShuffleNetV2, self).__init__()
if len(stages_repeats) != 3:
raise ValueError("expected stages_repeats as list of 3 positive ints")
if len(stages_out_channels) != 5:
raise ValueError("expected stages_out_channels as list of 5 positive ints")
self._stage_out_channels = stages_out_channels
input_channels = 3
output_channels = self._stage_out_channels[0]
self.conv1 = nn.Sequential(
nn.Conv2d(input_channels, output_channels, kernel_size=3, stride=2, padding=1, bias=False),
nn.BatchNorm2d(output_channels),
nn.ReLU(inplace=True)
)
input_channels = output_channels
self.maxpool = nn.MaxPool2d(kernel_size=3, stride=2, padding=1)
self.stage2: nn.Sequential
self.stage3: nn.Sequential
self.stage4: nn.Sequential
stage_names = ["stage{}".format(i) for i in [2, 3, 4]]
for name, repeats, output_channels in zip(stage_names, stages_repeats, self._stage_out_channels[1:]):
seq = [inverted_residual(input_channels, output_channels, 2)]
for i in range(repeats - 1):
seq.append(inverted_residual(output_channels, output_channels, 1))
setattr(self, name, nn.Sequential(*seq))
input_channels = output_channels
output_channels = self._stage_out_channels[-1]
self.conv5 = nn.Sequential(
nn.Conv2d(input_channels, output_channels, kernel_size=1, stride=1, padding=0, bias=False),
nn.BatchNorm2d(output_channels),
nn.ReLU(inplace=True)
)
self.fc = nn.Linear(output_channels, num_classes)
def _forward_impl(self, x: Tensor):
x = self.conv1(x)
x = self.maxpool(x)
x = self.stage2(x)
x = self.stage3(x)
x = self.stage4(x)
x = self.conv5(x)
x = x.mean([2, 3])
x = self.fc(x)
return x
def forward(self, x: Tensor):
return self._forward_impl(x)
"""
Constructs a ShuffleNetV2 with 1.0x output channels, as described in
`"ShuffleNet V2: Practical Guidelines for Efficient CNN Architecture Design"
`.
weight: https://download.pytorch.org/models/shufflenetv2_x1-5666bf0f80.pth
:param num_classes:
:return:
"""
def shufflenet_v2_x1_0(num_classes=1000):
model = ShuffleNetV2(stages_repeats=[4, 8, 4],
stages_out_channels=[24, 116, 232, 464, 1024],
num_classes=num_classes)
return model
"""
Constructs a ShuffleNetV2 with 0.5x output channels, as described in
`"ShuffleNet V2: Practical Guidelines for Efficient CNN Architecture Design"
`.
weight: https://download.pytorch.org/models/shufflenetv2_x0.5-f707e7126e.pth
:param num_classes:
:return:
"""
def shufflenet_v2_x0_5(num_classes=1000):
model = ShuffleNetV2(stages_repeats=[4, 8, 4],
stages_out_channels=[24, 48, 96, 192, 1024],
num_classes=num_classes)
return model