参考KDD会议时,对Linkedin的首席科学家Posse的讲座印象很深,不仅来自他那带法国口音且超快的语速,还来自与linkedin对数据的细致入微的分析。
title和abstract如下:
Key Lessons Learned Building Recommender Systems for Large-scale Social Networks
Christian Posse
Linkedin Inc.
Abstract
By helping members to connect, discover and share relevant content or find a new career
opportunity, recommender systems have become a critical component of user growth and
engagement for social networks. The multidimensional nature of engagement and diversity of
members on large-scale social networks have generated new infrastructure and modeling
challenges and opportunities in the development, deployment and operation of recommender
systems. This presentation will address some of these issues, focusing on the modeling side for
which new research is much needed while describing a recommendation platform that enables real-
time recommendation updates at scale as well as batch computations, and cross-leverage between
different product recommendations. Topics covered on the modeling side will include optimizing
for multiple competing objectives, solving contradicting business goals, modeling user intent and
interest to maximize placement and timeliness of the recommendations, utility metrics beyond CTR
that leverage both real-time tracking of explicit and implicit user feedback, gathering training data
for new product recommendations, virality preserving online testing and virtual profiling.
Categories & Subject Descriptors: H.2.8 [Database Management]: Data Mining.
Author Keywords: Recommender Systems, Real-time updates, Multi-Objective
Optimization, User Intent Modeling, Online Testing.
Bio
Dr. Christian Posse is Principal Scientist at LinkedIn Inc. where he leads the development of
recommendation solutions as well as the next generation online experimentation platform. Prior to
LinkedIn, Dr. Posse was a founding member and technology lead of Cisco Systems Inc. Network
Collaboration Business Unit where he designed the search and advanced social analytics of Pulse,
Cisco’s network-based search and collaboration platform for the enterprise. Prior to Cisco, Dr.
Posse worked in a wide range of environments, from holding faculty positions in US universities,
to leading the R&D at software companies and a US National Laboratory in the social networks,
biological networks and behavioral analytics fields. His interests are diverse and include predictive
analytics, search and recommendation engines, social networks analytics, computational social and
behavioral sciences, computational linguistics, and information fusion. He has written over 40
scientific peer-reviewed publications and holds several patents in those fields. Dr. Posse has a PhD
in Statistics from the Swiss Federal Institute of Technology, Switzerland.
linkedin的数据:
>50% of connections are from recommendations (PYMK)
>50% of job applications are from recommendations (JYMB)
>50% of group joins are from recommendations (GYML)
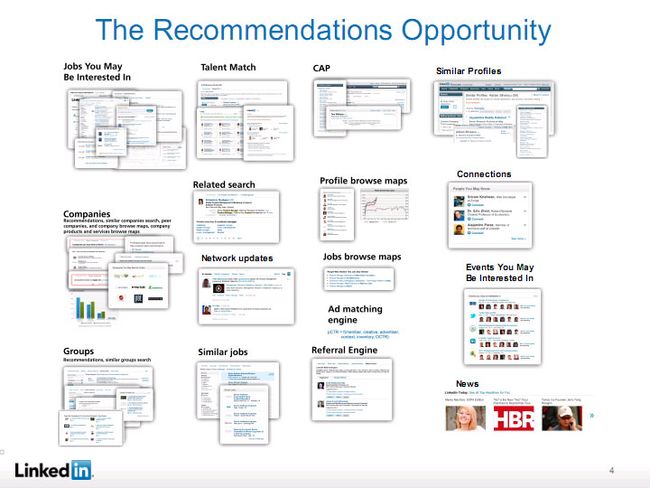
(Linkedin丰富的推荐类型)
what is a recommender system? --》 Posse的解释是 A Recommender selects a product that if acquired by the "buyer" maximizes value of both "buyer" and "seller" at a given point in time,从职位推荐的角度来看,就是让应聘者和招聘者直接的利益平衡并且收益最大化
User Experience Matters Most ---这是Posse提出的一个重要观点
不仅是用户的兴趣,求职意愿,还有用户在访问linkedin时的点击流程(user flow),都是会影响推荐效果的。另外推荐的描述方式(set right expectations),对结果的解释(explain recommendations)都是非常重要的。要从用户的角度来设计整个系统
具体的一些数据:
Job Recommendation use cases
user experience |
Optimization |
Impact on job application rate |
user intent/location |
Homepage personalization |
2.5X |
user flow/user intent |
Before vs. after having applied to a job |
7x |
user flow |
linkedin homepage vs. jobs homepage |
10x |
location |
center rail vs. right rail |
5x |
message |
followers vs. leaders |
2x |
另外interact with the user也很重要,这个考验产品设计的功力了
leverage social referral ,这点Posse在Recsys的论文里写了,现场我没做记录,但记得对推荐效果的提升非常有帮助。论文名称是:
M. Amin, B. Yan, S. Sriram, A. Bhasin and Christian Posse, Social Referral: Using Network Connections to Deliver Recommendations, Proceedings of the sixth ACM conference on Recommender Systems (Recsys' 2012)