这部分都是一些介绍,不需要主观加工,直接收集起来分享之。
1. OpenCV中HOG特征参数图解
http://blog.csdn.net/raocong2010/article/details/6239431
最近要做图像特征提取,可能要用下HOG特征,所以研究了下OpenCV的HOG描述子。OpenCV中的HOG特征提取功能使用了HOGDescriptor这个类来进行封装,其中也有现成的行人检测的接口。然而,无论是OpenCV官方说明文档还是各个中英文网站目前都没有这个类的使用说明,所以在这里把研究的部分心得分享一下。
首先我们进入HOGDescriptor所在的头文件,看看它的构造函数需要哪些参数。
[cpp] view plain copy print ?
- CV_WRAP HOGDescriptor() : winSize(64,128), blockSize(16,16), blockStride(8,8),
- cellSize(8,8), nbins(9), derivAperture(1), winSigma(-1),
- histogramNormType(HOGDescriptor::L2Hys), L2HysThreshold(0.2), gammaCorrection(true),
- nlevels(HOGDescriptor::DEFAULT_NLEVELS)
- {}
CV_WRAP HOGDescriptor() : winSize(64,128), blockSize(16,16), blockStride(8,8),
cellSize(8,8), nbins(9), derivAperture(1), winSigma(-1),
histogramNormType(HOGDescriptor::L2Hys), L2HysThreshold(0.2), gammaCorrection(true),
nlevels(HOGDescriptor::DEFAULT_NLEVELS)
{}
[cpp] view plain copy print ?
- CV_WRAP HOGDescriptor(Size _winSize, Size _blockSize, Size _blockStride,
- Size _cellSize, int _nbins, int _derivAperture=1, double _winSigma=-1,
- int _histogramNormType=HOGDescriptor::L2Hys,
- double _L2HysThreshold=0.2, bool _gammaCorrection=false,
- int _nlevels=HOGDescriptor::DEFAULT_NLEVELS)
- : winSize(_winSize), blockSize(_blockSize), blockStride(_blockStride), cellSize(_cellSize),
- nbins(_nbins), derivAperture(_derivAperture), winSigma(_winSigma),
- histogramNormType(_histogramNormType), L2HysThreshold(_L2HysThreshold),
- gammaCorrection(_gammaCorrection), nlevels(_nlevels)
- {}
CV_WRAP HOGDescriptor(Size _winSize, Size _blockSize, Size _blockStride,
Size _cellSize, int _nbins, int _derivAperture=1, double _winSigma=-1,
int _histogramNormType=HOGDescriptor::L2Hys,
double _L2HysThreshold=0.2, bool _gammaCorrection=false,
int _nlevels=HOGDescriptor::DEFAULT_NLEVELS)
: winSize(_winSize), blockSize(_blockSize), blockStride(_blockStride), cellSize(_cellSize),
nbins(_nbins), derivAperture(_derivAperture), winSigma(_winSigma),
histogramNormType(_histogramNormType), L2HysThreshold(_L2HysThreshold),
gammaCorrection(_gammaCorrection), nlevels(_nlevels)
{}
[cpp] view plain copy print ?
- CV_WRAP HOGDescriptor(const String& filename)
- {
- load(filename);
- }
CV_WRAP HOGDescriptor(const String& filename)
{
load(filename);
}
[cpp] view plain copy print ?
- HOGDescriptor(const HOGDescriptor& d)
- {
- d.copyTo(*this);
- }
HOGDescriptor(const HOGDescriptor& d)
{
d.copyTo(*this);
}
我们看到HOGDescriptor一共有4个构造函数,前三个有CV_WRAP前缀,表示它们是从DLL里导出的函数,即我们在程序当中可以调用的函数;最后一个没有上述的前缀,所以我们暂时用不到,它其实就是一个拷贝构造函数。
下面我们就把注意力放在前面的构造函数的参数上面吧,这里有几个重要的参数要研究下:winSize(64,128), blockSize(16,16), blockStride(8,8), cellSize(8,8), nbins(9)。上面这些都是HOGDescriptor的成员变量,括号里的数值是它们的默认值,它们反应了HOG描述子的参数。这里做了几个示意图来表示它们的含义。
窗口大小 winSize
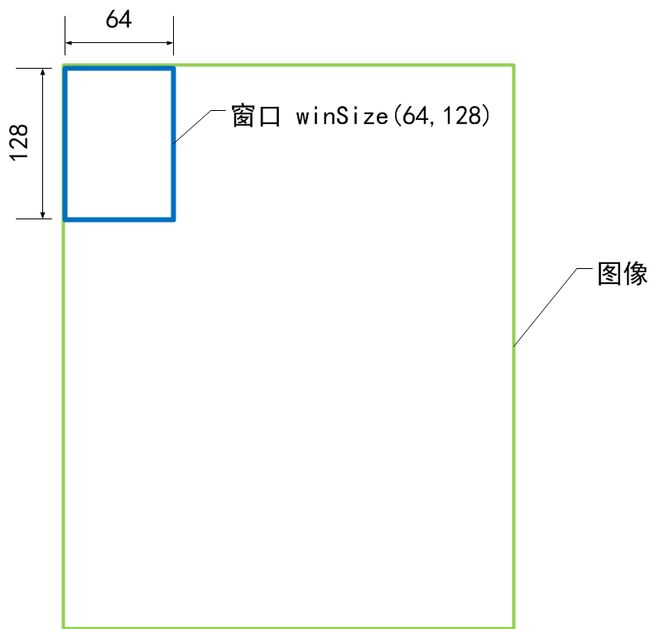
块大小 blockSize
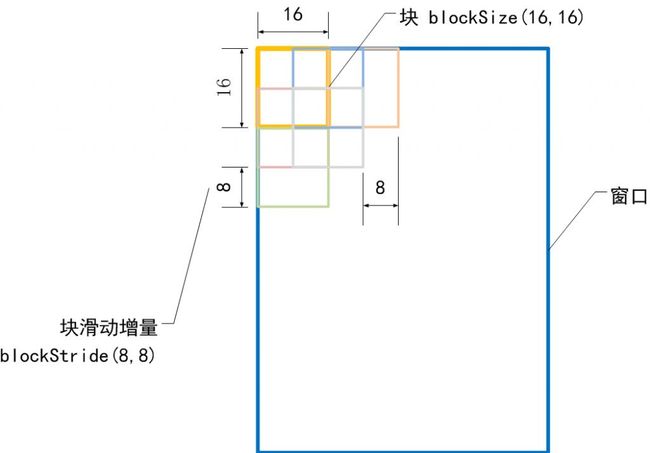
胞元大小 cellSize
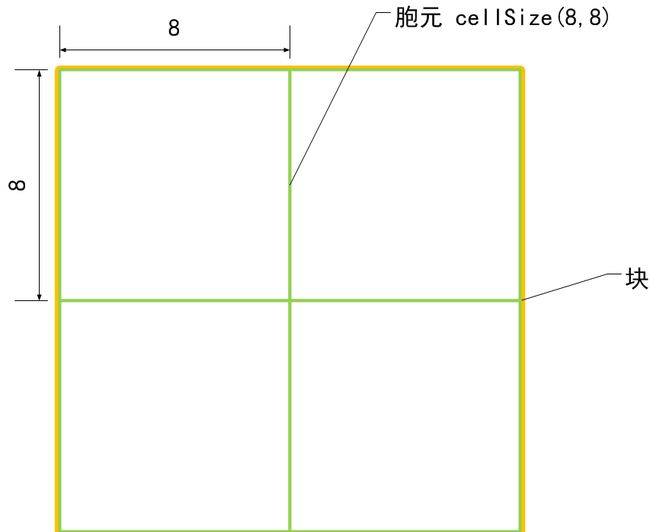
梯度方向数 nbins
nBins表示在一个胞元(cell)中统计梯度的方向数目,例如nBins=9时,在一个胞元内统计9个方向的梯度直方图,每个方向为180/9=20度。
HOG描述子维度
在确定了上述的参数后,我们就可以计算出一个HOG描述子的维度了。OpenCV中的HOG源代码是按照下面的式子计算出描述子的维度的。
[cpp] view plain copy print ?
- size_t HOGDescriptor::getDescriptorSize() const
- {
- CV_Assert(blockSize.width % cellSize.width == 0 &&
- blockSize.height % cellSize.height == 0);
- CV_Assert((winSize.width - blockSize.width) % blockStride.width == 0 &&
- (winSize.height - blockSize.height) % blockStride.height == 0 );
- return (size_t)nbins*
- (blockSize.width/cellSize.width)*
- (blockSize.height/cellSize.height)*
- ((winSize.width - blockSize.width)/blockStride.width + 1)*
- ((winSize.height - blockSize.height)/blockStride.height + 1);
- }
size_t HOGDescriptor::getDescriptorSize() const
{
CV_Assert(blockSize.width % cellSize.width == 0 &&
blockSize.height % cellSize.height == 0);
CV_Assert((winSize.width - blockSize.width) % blockStride.width == 0 &&
(winSize.height - blockSize.height) % blockStride.height == 0 );
return (size_t)nbins*
(blockSize.width/cellSize.width)*
(blockSize.height/cellSize.height)*
((winSize.width - blockSize.width)/blockStride.width + 1)*
((winSize.height - blockSize.height)/blockStride.height + 1);
}
参考文献
OpenCV中的HOG算法来源于Histograms of Oriented Gradients for Human Detection, CVPR 2005。详细的算法可以参考这个文章。
2.OpenCV中HOG特征参数个数
http://gz-ricky.blogbus.com/logs/85326280.html
在OpenCV实现的是R-HOG, 即对图像img->窗口window->块block->细胞单元cell进行向量统计
首先看描述器的构造函数, 我用
HOGDescriptor *desc=newHOGDescriptor(cvSize(40,80),cvSize(10,20),cvSize(5,10),cvSize(5,5),9);
进行测试..
这里的window为(40, 80), block为(10, 20), block的步进stride是(5, 10), 细胞单元cell是5 * 5像素, 每个cell的直方图bin是9.
于是,
对每一个cell, 有9个向量
对每一个block, 有2*4个cell, 所以有72个向量
对于window而言, 计算block个数的方法是, 对两个方向计算 (window_size -block_size)/block_stride + 1, 算得共有7*7 个block, 共有72*49=3528个向量
在搜索img, 计算图片特征的时候, 调用desc->compute(img,w,cvSize(10,20),cvSize(0,0));
其中img是输入图像, w是保存向量的vector, 第三个是window的步进, 第四个是padding, 用于填充图片以适应大小的.
当设置padding为默认(0,0)时, 计算(img_size - window_size) / window_stride +1 不一定为整数
在compute函数中可以看到:
padding.width = (int)alignSize(std::max(padding.width, 0), cacheStride.width);
padding.height = (int)alignSize(std::max(padding.height, 0),cacheStride.height);
即padding的大小会自动适应stride的值.
gz_ricky输入的图片是96*160的, 对应了5.6 * 5, 经函数调整后变成6 * 5 =30
所以对这张96*160的图片, 共有105840个特征向量
PS. 如果设置了padding的值, 图片就会先伸展padding*2, 或许是和内部那个paddingTL和paddingBR两个有关, 即在Top-Left和Button-Right两个方向都扩展. 计算特征数方法同上.
hog特征值会生成以后, 可以转入svm训练的阶段了