Real-Time compressive Tracking, Kaihua Zhang, ECCV2012
这篇论文由香港理工大学张开华发表在2012年的ECCV之上,论文附有数据对比及代码。
cvchina: http://www.cvchina.info/2012/07/31/real-time-compressive-tracking/comment-page-1/#comments
个人主页及代码下载; http://www4.comp.polyu.edu.hk/~cslzhang/CT/CT.htm
优酷跟踪效果:http://v.youku.com/v_show/id_XNDMzODcxNjcy.html
下面分享一下个人对于(Compressive Tracking)CT的个人观点,文章中与当前很多前沿技术做了效果对比,包括PAMI2012上的著名的TLD。但理论研究与实际工程应用差距巨大。
下面我们根据代码,来分析一下CT的主要算法步骤,因本人水平有限,欢迎拍砖。
论文中的流程图
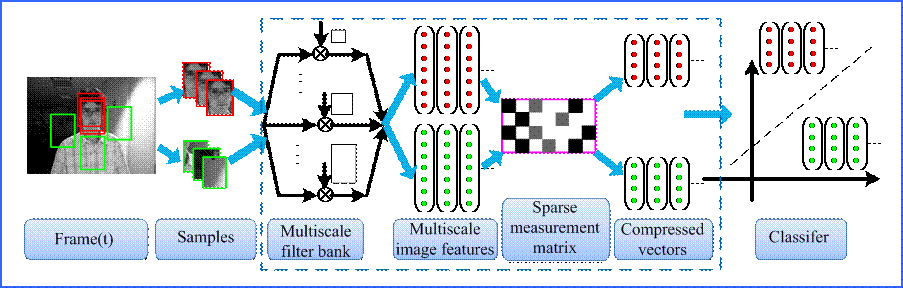
在 t 帧中首先在目标周围选择正样本,距离目标较远处选择负样本,对正负样本分别进行多尺度滤波而进行压缩获得正负特征以此来训练分类器。
在 t+1 帧中,在上一帧的目标位置周围选取可能的目标框,而后提取特征利用分类器选取得分最高者进行输出作为当前帧的框图。
首先,在第一帧中圈出目标位置。进行初始化。
- void CompressiveTracker::init(Mat& _frame, Rect& _objectBox)
- {
- // compute feature template
- HaarFeature(_objectBox, featureNum);
-
- // compute sample templates
- sampleRect(_frame, _objectBox, rOuterPositive, 0, 1000000, samplePositiveBox);
- sampleRect(_frame, _objectBox, rSearchWindow*1.5, rOuterPositive+4.0, 100, sampleNegativeBox);
-
- integral(_frame, imageIntegral, CV_32F);
-
- getFeatureValue(imageIntegral, samplePositiveBox, samplePositiveFeatureValue);
- getFeatureValue(imageIntegral, sampleNegativeBox, sampleNegativeFeatureValue);
- classifierUpdate(samplePositiveFeatureValue, muPositive, sigmaPositive, learnRate);
- classifierUpdate(sampleNegativeFeatureValue, muNegative, sigmaNegative, learnRate);
- }
HaarFeature函数主要目的在与产生多尺度滤波器。此函数中会随机生成50次,每次 2 或 3 个选择框。 而论文当中多次强调的压缩矩阵R应该就是此矩阵。此矩阵在初始化时生成,跟踪过程当中不再变化。而其高斯性稀疏性是通过随机产生实现的。
而后 SampleRect 用于产生 正负样本,其中的各个参数及论文中所提更新过程中的α和β。
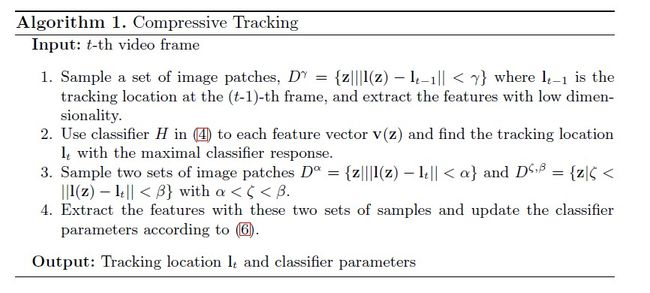
Intergral是对图像进行积分化,生成积分图,方便计算Haar特征。
getFeatureValue即分别获得所获取正样本 负样本的Haar特征。每维特征有50维。对应前面取到的压缩矩阵R。
而后进行分类器的初始化,分类器采用朴素贝叶斯分类器。更新过程采用论文当中的如下公式。至此,则已完成分类器的初始化。
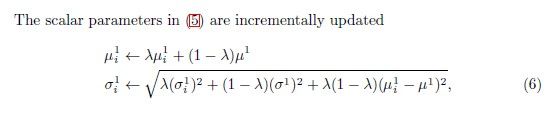
在 以后若干 帧中,
- void CompressiveTracker::processFrame(Mat& _frame, Rect& _objectBox)
- {
-
- sampleRect(_frame, _objectBox, rSearchWindow,detectBox);
- integral(_frame, imageIntegral, CV_32F);
- getFeatureValue(imageIntegral, detectBox, detectFeatureValue);
- int radioMaxIndex;
- float radioMax;
- radioClassifier(muPositive, sigmaPositive, muNegative, sigmaNegative, detectFeatureValue, radioMax, radioMaxIndex);
- _objectBox = detectBox[radioMaxIndex];
-
-
- sampleRect(_frame, _objectBox, rOuterPositive, 0.0, 1000000, samplePositiveBox);
- sampleRect(_frame, _objectBox, rSearchWindow*1.5, rOuterPositive+4.0, 100, sampleNegativeBox);
-
- getFeatureValue(imageIntegral, samplePositiveBox, samplePositiveFeatureValue);
- getFeatureValue(imageIntegral, sampleNegativeBox, sampleNegativeFeatureValue);
- classifierUpdate(samplePositiveFeatureValue, muPositive, sigmaPositive, learnRate);
- classifierUpdate(sampleNegativeFeatureValue, muNegative, sigmaNegative, learnRate);
- }
在前一帧目标周围选择若干候选位置,在当前帧的积分图中对每个候选位置提取 Haar 特征,选取分类器得分最高者作为输出。
而后在新的位置周围按照前述方式对分类器进行更新。
CT实时性确实是一个很大的诱惑性。因为他只在目标周围进行选择,而且特征及分类器设计都十分简单。 这也导致其出现相应的问题。
当然这是几乎所有跟踪算法都无法避免的的问题。
1.当目标大部分被遮挡时,跟踪结果会立即出错。比如在跟踪行人时,如果出现另外的人擦肩而过,则完全跟踪到另外的人身上。
2.当目标离开视野再度出现时,不可能跟踪到。
3.该方法没有解决尺度问题。当目标由远及近或者反之,尺度都是一样的。
而以上三个问题TLD中都已经涉及解决。
4. CT主要的优点在于其实时性,论文中经过与诸方法比较,时间仅次于TLD,满足实时性要求。