最大稳定极值区域(MSER-Maximally Stable Extremal Regions)可以用于图像的斑点区域检测。该算法最早是由Matas等人于2002年提出,它是基于分水岭的概念。
MSER的基本原理是对一幅灰度图像(灰度值为0~255)取阈值进行二值化处理,阈值从0到255依次递增。阈值的递增类似于分水岭算法中的水面的上升,随着水面的上升,有一些较矮的丘陵会被淹没,如果从天空往下看,则大地分为陆地和水域两个部分,这类似于二值图像。在得到的所有二值图像中,图像中的某些连通区域变化很小,甚至没有变化,则该区域就被称为最大稳定极值区域。这类似于当水面持续上升的时候,有些被水淹没的地方的面积没有变化。它的数学定义为:
q(i)=|Qi+△-Qi-△|/|Qi| (1)
其中,Qi表示阈值为i时的某一连通区域,△为灰度阈值的微小变化量,q(i)为阈值是i时的区域Qi的变化率。当q(i)为局部极小值时,则Qi为最大稳定极值区域。
需要说明的是,上述做法只能检测出灰度图像的黑色区域,不能检测出白色区域,因此还需要对原图进行反转,然后再进行阈值从0~255的二值化处理过程。这两种操作又分别称为MSER+和MSER-。
MSER具有以下特点:
1、对图像灰度具有仿射变换的不变性;
2、稳定性:具有相同阈值范围内所支持的区域才会被选择;
3、无需任何平滑处理就可以实现多尺度检测,即小的和大的结构都可以被检测到。
MSER的原理比较简单,但要更快更好的实现它,是需要一定的算法、数据结构和编程技巧的。David Nister等人于2008年提出了Linear Time Maximally Stable Extremal Regions算法,该算法要比原著提出的算法快,opencv就是利用该算法实现MSER的。但这里要说明一点的是,opencv不是利用公式1计算MSER的,而是利用更易于实现的改进方法:
q(i)=|Qi-Qi-△|/|Qi-△| (2)
David Nister提出的算法是基于改进的分水岭算法,即当往一个固定的地方注水的时候,只有当该地方的沟壑被水填满以后,水才会向其四周溢出,随着注水量的不断增加,各个沟壑也会逐渐被水淹没,但各个沟壑的水面不是同时上升的,它是根据水漫过地方的先后顺序,一个沟壑一个沟壑地填满水,只有当相邻两个沟壑被水连通在一起以后,水面对于这两个沟壑来说才是同时上升的。该算法的具体步骤如下:
1、初始化栈和堆,栈用于存储组块(组块就是区域,就相当于水面,水漫过的地方就会出现水面,水面的高度就是图像的灰度值,因此用灰度值来表示组块的值),堆用于存储组块的边界像素,相当于水域的岸边,岸边要高于水面的,因此边界像素的灰度值一定不小于它所包围的区域(即组块)的灰度值。首先向栈内放入一个虚假的组块,当该组块被弹出时意味着程序的结束;
2、把图像中的任意一个像素(一般选取图像的左上角像素)作为源像素,标注该像素为已访问过,并且把该像素的灰度值作为当前值。这一步相当于往源像素这一地点注水;
3、向栈内放入一个空组块,该组块的值是当前值;
4、按照顺序搜索当前值的4-领域内剩余的边缘,对于每一个邻域,检查它是否已经被访问过,如果没有,则标注它为已访问过并检索它的灰度值,如果灰度值不小于当前值,则把它放入用于存放边界像素的堆中。另一方面,如果领域灰度值小于当前值,则把当前值放入堆中,而把领域值作为当前值,并回到步骤3;
5、累计栈顶组块的像素个数,即计算区域面积,这是通过循环累计得到的,这一步相当于水面的饱和;
6、弹出堆中的边界像素。如果堆是空的,则程序结束;如果弹出的边界像素的灰度值等于当前值,则回到步骤4;
7、从堆中得到的像素值会大于当前值,因此我们需要处理栈中所有的组块,直到栈中的组块的灰度值大于当前边界像素灰度值为止。然后回到步骤4。
至于如何处理组块,则需要进入处理栈子模块中,传入该子模块的值为步骤7中从堆中提取得到的边界像素灰度值。子模块的具体步骤为:
1)、处理栈顶的组块,即根据公式2计算最大稳定区域,判断其是否为极值区域;
2)、如果边界像素灰度值小于距栈顶第二个组块的灰度值,那么设栈顶组块的灰度值为边界像素灰度值,并退出该子模块。之所以会出现这种情况,是因为在栈顶组块和第二个组块之间还有组块没有被检测处理,因此我们需要改变栈顶组块的灰度值为边界像素灰度值(相当于这两层的组块进行了合并),并回到主程序,再次搜索组块;
3)、弹出栈顶组块,并与目前栈顶组块合并;
4)、如果边界像素灰度值大于栈顶组块的灰度值,则回到步骤1。
在opencv2.4.9中,MSER算法是用类的方法给出的:
- class MserFeatureDetector : public FeatureDetector
- {
- public:
- MserFeatureDetector( CvMSERParams params=cvMSERParams() );
- MserFeatureDetector( int delta, int minArea, int maxArea,
- double maxVariation, double minDiversity,
- int maxEvolution, double areaThreshold,
- double minMargin, int edgeBlurSize );
- virtual void read( const FileNode& fn );
- virtual void write( FileStorage& fs ) const;
- protected:
- ...
- };
而具体的MSER类为:
- class MSER : public CvMSERParams
- {
- public:
-
-
- MSER();
-
-
- MSER( int _delta, int _min_area, int _max_area,
- float _max_variation, float _min_diversity,
- int _max_evolution, double _area_threshold,
- double _min_margin, int _edge_blur_size );
-
-
-
- void operator()( const Mat& image, vector<vector<Point> >& msers, const Mat& mask ) const;
- };
- MSER算法所需要的参数较多:
- delta为灰度值的变化量,即公式1和2中的△;
- _min_area和_max_area为检测到的组块面积的范围;
- _max_variation为最大的变化率,即如果公式1和2中的q(i)小于该值,则被认为是最大稳定极值区域;
- _min_diversity为稳定区域的最小变换量。
- 其他的参数用于对彩色图像的MSER检测,这里不做介绍。
- MSER类通过重载( )运算符,得到了最大稳定极值区域的点集msers,其中image为输入图像,mask为掩码矩阵。
- 下面我们就对MSER类的源码进行分析:
- void MSER::operator()( const Mat& image, vector<vector<Point> >& dstcontours, const Mat& mask ) const
- {
- CvMat _image = image, _mask, *pmask = 0;
- if( mask.data )
- pmask = &(_mask = mask);
- MemStorage storage(cvCreateMemStorage(0));
- Seq<CvSeq*> contours;
-
-
- extractMSER( &_image, pmask, &contours.seq, storage,
- MSERParams(delta, minArea, maxArea, maxVariation, minDiversity,
- maxEvolution, areaThreshold, minMargin, edgeBlurSize));
- SeqIterator<CvSeq*> it = contours.begin();
- size_t i, ncontours = contours.size();
- dstcontours.resize(ncontours);
-
- for( i = 0; i < ncontours; i++, ++it )
- Seq<Point>(*it).copyTo(dstcontours[i]);
- }
extractMSER函数首先定义了一些变量,并进行参数的判断。然后根据输入图像的类型分别调用不同的函数:
- static void
- extractMSER( CvArr* _img,
- CvArr* _mask,
- CvSeq** _contours,
- CvMemStorage* storage,
- MSERParams params )
- {
- CvMat srchdr, *src = cvGetMat( _img, &srchdr );
- CvMat maskhdr, *mask = _mask ? cvGetMat( _mask, &maskhdr ) : 0;
- CvSeq* contours = 0;
-
- CV_Assert(src != 0);
- CV_Assert(CV_MAT_TYPE(src->type) == CV_8UC1 || CV_MAT_TYPE(src->type) == CV_8UC3);
- CV_Assert(mask == 0 || (CV_ARE_SIZES_EQ(src, mask) && CV_MAT_TYPE(mask->type) == CV_8UC1));
- CV_Assert(storage != 0);
-
- contours = *_contours = cvCreateSeq( 0, sizeof(CvSeq), sizeof(CvSeq*), storage );
-
-
-
-
- switch ( CV_MAT_TYPE(src->type) )
- {
- case CV_8UC1:
- extractMSER_8UC1( src, mask, contours, storage, params );
- break;
- case CV_8UC3:
- extractMSER_8UC3( src, mask, contours, storage, params );
- break;
- }
- }
灰度图像的MSER处理方法就是应用本文介绍的方法:
- static void extractMSER_8UC1( CvMat* src,
- CvMat* mask,
- CvSeq* contours,
- CvMemStorage* storage,
- MSERParams params )
- {
-
-
- int step = 8;
-
- int stepgap = 3;
-
- while ( step < src->step+2 )
- {
- step <<= 1;
- stepgap++;
- }
- int stepmask = step-1;
-
-
-
- CvMat* img = cvCreateMat( src->rows+2, step, CV_32SC1 );
-
- int* ioptr = img->data.i+step+1;
- int* imgptr;
-
-
-
-
- int** heap = (int**)cvAlloc( (src->rows*src->cols+256)*sizeof(heap[0]) );
- int** heap_start[256];
-
- heap_start[0] = heap;
-
-
-
- LinkedPoint* pts = (LinkedPoint*)cvAlloc( src->rows*src->cols*sizeof(pts[0]) );
-
- MSERGrowHistory* history = (MSERGrowHistory*)cvAlloc( src->rows*src->cols*sizeof(history[0]) );
-
- MSERConnectedComp comp[257];
-
-
-
-
- imgptr = preprocessMSER_8UC1( img, heap_start, src, mask );
-
- extractMSER_8UC1_Pass( ioptr, imgptr, heap_start, pts, history, comp, step, stepmask, stepgap, params, -1, contours, storage );
-
-
- imgptr = preprocessMSER_8UC1( img, heap_start, src, mask );
- extractMSER_8UC1_Pass( ioptr, imgptr, heap_start, pts, history, comp, step, stepmask, stepgap, params, 1, contours, storage );
-
-
-
- cvFree( &history );
- cvFree( &heap );
- cvFree( &pts );
- cvReleaseMat( &img );
- }
preprocessMSER_8UC1函数为预处理的过程,主要就是对宽度扩展以后的图像矩阵进行赋值,每一位都赋予不同的含义:
// to preprocess src image to followingformat
// 32-bit image
// > 0 is available, < 0 is visited
// 17~19 bits is the direction
// 8~11 bits is the bucket it falls to (forBitScanForward)
// 0~8 bits is the color
/*定义图像矩阵的数据格式为有符号32位整型,最高一位表示是否被访问过,0表示没有被访问过,1表示被访问过(因为是有符号数,所以最高一位是1也就是负数);16~18位(这里源码注解有误)表示4邻域的方向;0~7位表示该像素的灰度值*/
//img代表图像矩阵,MSER处理的是该矩阵
//src为输入原图
//heap_cur为边界像素堆
//mask为掩码
- static int* preprocessMSER_8UC1( CvMat* img,
- int*** heap_cur,
- CvMat* src,
- CvMat* mask )
- {
- int srccpt = src->step-src->cols;
-
- int cpt_1 = img->cols-src->cols-1;
-
- int* imgptr = img->data.i;
-
- int* startptr;
-
-
- int level_size[256];
- for ( int i = 0; i < 256; i++ )
- level_size[i] = 0;
-
- for ( int i = 0; i < src->cols+2; i++ )
- {
- *imgptr = -1;
- imgptr++;
- }
-
- imgptr += cpt_1-1;
-
- uchar* srcptr = src->data.ptr;
- if ( mask )
- {
- startptr = 0;
-
- uchar* maskptr = mask->data.ptr;
-
- for ( int i = 0; i < src->rows; i++ )
- {
- *imgptr = -1;
- imgptr++;
- for ( int j = 0; j < src->cols; j++ )
- {
- if ( *maskptr )
- {
- if ( !startptr )
- startptr = imgptr;
- *srcptr = 0xff-*srcptr;
- level_size[*srcptr]++;
-
- *imgptr = ((*srcptr>>5)<<8)|(*srcptr);
- } else {
- *imgptr = -1;
- }
-
- imgptr++;
- srcptr++;
- maskptr++;
- }
-
- *imgptr = -1;
- imgptr += cpt_1;
- srcptr += srccpt;
- maskptr += srccpt;
- }
- } else {
- startptr = imgptr+img->cols+1;
-
- for ( int i = 0; i < src->rows; i++ )
- {
- *imgptr = -1;
- imgptr++;
- for ( int j = 0; j < src->cols; j++ )
- {
- *srcptr = 0xff-*srcptr;
- level_size[*srcptr]++;
- *imgptr = ((*srcptr>>5)<<8)|(*srcptr);
- imgptr++;
- srcptr++;
- }
- *imgptr = -1;
- imgptr += cpt_1;
- srcptr += srccpt;
- }
- }
-
- for ( int i = 0; i < src->cols+2; i++ )
- {
- *imgptr = -1;
- imgptr++;
- }
-
-
-
- heap_cur[0][0] = 0;
- for ( int i = 1; i < 256; i++ )
- {
- heap_cur[i] = heap_cur[i-1]+level_size[i-1]+1;
- heap_cur[i][0] = 0;
- }
-
- return startptr;
- }
extractMSER_8UC1_Pass函数执行MSER算法,MSER-和MSER+都执行该函数,但可以通过参数color来区分。
- static void extractMSER_8UC1_Pass( int* ioptr,
- int* imgptr,
- int*** heap_cur,
- LinkedPoint* ptsptr,
- MSERGrowHistory* histptr,
- MSERConnectedComp* comptr,
- int step,
- int stepmask,
- int stepgap,
- MSERParams params,
- int color,
- CvSeq* contours,
- CvMemStorage* storage )
- {
-
- comptr->grey_level = 256;
-
-
- comptr++;
-
- comptr->grey_level = (*imgptr)&0xff;
-
- initMSERComp( comptr );
-
- *imgptr |= 0x80000000;
-
- heap_cur += (*imgptr)&0xff;
-
- int dir[] = { 1, step, -1, -step };
- #ifdef __INTRIN_ENABLED__
- unsigned long heapbit[] = { 0, 0, 0, 0, 0, 0, 0, 0 };
- unsigned long* bit_cur = heapbit+(((*imgptr)&0x700)>>8);
- #endif
-
- for ( ; ; )
- {
-
-
-
- while ( ((*imgptr)&0x70000) < 0x40000 )
- {
-
-
- int* imgptr_nbr = imgptr+dir[((*imgptr)&0x70000)>>16];
-
- if ( *imgptr_nbr >= 0 )
- {
-
- *imgptr_nbr |= 0x80000000;
-
- if ( ((*imgptr_nbr)&0xff) < ((*imgptr)&0xff) )
- {
-
-
-
-
-
- (*heap_cur)++;
-
- **heap_cur = imgptr;
-
- *imgptr += 0x10000;
-
-
- heap_cur += ((*imgptr_nbr)&0xff)-((*imgptr)&0xff);
- #ifdef __INTRIN_ENABLED__
- _bitset( bit_cur, (*imgptr)&0x1f );
- bit_cur += (((*imgptr_nbr)&0x700)-((*imgptr)&0x700))>>8;
- #endif
- imgptr = imgptr_nbr;
-
- comptr++;
- initMSERComp( comptr );
- comptr->grey_level = (*imgptr)&0xff;
-
- continue;
- } else {
-
-
-
- heap_cur[((*imgptr_nbr)&0xff)-((*imgptr)&0xff)]++;
-
- *heap_cur[((*imgptr_nbr)&0xff)-((*imgptr)&0xff)] = imgptr_nbr;
- #ifdef __INTRIN_ENABLED__
- _bitset( bit_cur+((((*imgptr_nbr)&0x700)-((*imgptr)&0x700))>>8), (*imgptr_nbr)&0x1f );
- #endif
- }
- }
- *imgptr += 0x10000;
- }
-
- int imsk = (int)(imgptr-ioptr);
-
-
- ptsptr->pt = cvPoint( imsk&stepmask, imsk>>stepgap );
-
-
-
-
- accumulateMSERComp( comptr, ptsptr );
-
- ptsptr++;
-
-
-
- if ( **heap_cur )
- {
- imgptr = **heap_cur;
- (*heap_cur)--;
- #ifdef __INTRIN_ENABLED__
- if ( !**heap_cur )
- _bitreset( bit_cur, (*imgptr)&0x1f );
- #endif
-
-
- } else {
- #ifdef __INTRIN_ENABLED__
- bool found_pixel = 0;
- unsigned long pixel_val;
- for ( int i = ((*imgptr)&0x700)>>8; i < 8; i++ )
- {
- if ( _BitScanForward( &pixel_val, *bit_cur ) )
- {
- found_pixel = 1;
- pixel_val += i<<5;
- heap_cur += pixel_val-((*imgptr)&0xff);
- break;
- }
- bit_cur++;
- }
- if ( found_pixel )
- #else
- heap_cur++;
- unsigned long pixel_val = 0;
-
- for ( unsigned long i = ((*imgptr)&0xff)+1; i < 256; i++ )
- {
- if ( **heap_cur )
- {
- pixel_val = i;
- break;
- }
-
- heap_cur++;
- }
- if ( pixel_val )
- #endif
- {
- imgptr = **heap_cur;
- (*heap_cur)--;
- #ifdef __INTRIN_ENABLED__
- if ( !**heap_cur )
- _bitreset( bit_cur, pixel_val&0x1f );
- #endif
-
- if ( pixel_val < comptr[-1].grey_level )
-
- {
-
-
- if ( MSERStableCheck( comptr, params ) )
- {
-
- CvContour* contour = MSERToContour( comptr, storage );
- contour->color = color;
-
- cvSeqPush( contours, &contour );
- }
- MSERNewHistory( comptr, histptr );
-
- comptr[0].grey_level = pixel_val;
- histptr++;
- } else {
-
-
-
- for ( ; ; )
- {
-
- comptr--;
-
- MSERMergeComp( comptr+1, comptr, comptr, histptr );
- histptr++;
-
- if ( pixel_val <= comptr[0].grey_level )
- break;
-
- if ( pixel_val < comptr[-1].grey_level )
- {
-
- if ( MSERStableCheck( comptr, params ) )
- {
- CvContour* contour = MSERToContour( comptr, storage );
- contour->color = color;
- cvSeqPush( contours, &contour );
- }
- MSERNewHistory( comptr, histptr );
- comptr[0].grey_level = pixel_val;
- histptr++;
- break;
- }
- }
- }
- } else
-
- break;
- }
- }
- }
MSER就分析到这里,至于其中用到的一些子函数就不再分析。这里还要说明一点的是,MSER+和MSER-使用的都是同一个函数,两者的区别是一个组块面积增加,另一个减少。说实话,该部分的内容数据结构较为复杂,有一些内容我理解得也不透彻,比如结构MSERGrowHistory真正的含义还比较模糊。
下面就给出最大稳定极值区域检测的应用实例:
- #include "opencv2/core/core.hpp"
- #include "opencv2/highgui/highgui.hpp"
- #include "opencv2/imgproc/imgproc.hpp"
- #include <opencv2/features2d/features2d.hpp>
- #include <iostream>
- using namespace cv;
- using namespace std;
-
- int main(int argc, char *argv[])
- {
- Mat src,gray;
- src = imread("lenna.bmp");
- cvtColor( src, gray, CV_BGR2GRAY );
-
- MSER ms;
-
- vector<vector<Point>> regions;
- ms(gray, regions, Mat());
-
- for (int i = 0; i < regions.size(); i++)
- {
- ellipse(gray, fitEllipse(regions[i]), Scalar(255));
- }
- imshow("mser", gray);
- waitKey(0);
- return 0;
- }
结果如下图所示。本文介绍的方法只适用于灰度图像,所以如果输入的是彩色图像,要把它转换为灰度图像。另外在创建MSER类的时候,我们使用的是缺省构造函数,因此MSER算法所需的参数是系统默认的。所以我们检测的结果与David Nister等人在原著中的检测结果略有不同。
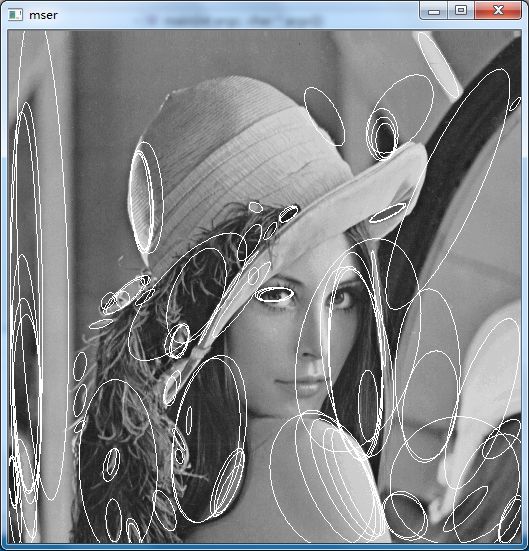