在计算loss的时候,最常见的一句话就是tf.nn.softmax_cross_entropy_with_logits,那么它到底是怎么做的呢?
首先明确一点,loss是代价值,也就是我们要最小化的值
tf.nn.softmax_cross_entropy_with_logits(logits, labels, name=None)
除去name参数用以指定该操作的name,与方法有关的一共两个参数
:
第一个参数logits:就是神经网络最后一层的输出,如果有batch的话,它的大小就是[batchsize,num_classes],单样本的话,大小就是num_classes
第二个参数labels:实际的标签,大小同上
具体的执行流程大概分为两步:
第一步是先对网络最后一层的输出做一个softmax,这一步通常是求取输出属于某一类的概率,对于单样本而言,输出就是一个num_classes
大小的向量([Y1,Y2,Y3...]其中Y1,Y2,Y3...分别代表了是属于该类的概率)
softmax的公式是: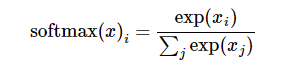
至于为什么是用的这个公式?这里不介绍了,涉及到比较多的理论证明
第二步是softmax的输出向量[Y1,Y2,Y3...]和样本的实际标签做一个交叉熵,公式如下:
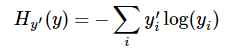
其中
指代实际的标签中第i个的值(用mnist数据举例,如果是3,那么标签是[0,0,0,1,0,0,0,0,0,0],除了第4个值为1,其他全为0)
就是softmax的输出向量[Y1,Y2,Y3...]
中,第i个元素的值
显而易见,预测
越准确,结果的值越小(别忘了前面还有负号),最后求一个平均,得到我们想要的loss
注意!!!这个函数的返回值并不是一个数,而是一个向量,如果要求交叉熵,我们要再做一步tf.reduce_sum操作,就是对向量里面所有元素求和,最后才得到
,如果求loss,则要做一步tf.reduce_mean操作,对向量求均值!
理论讲完了,上代码
- import tensorflow as tf
-
-
- logits=tf.constant([[1.0,2.0,3.0],[1.0,2.0,3.0],[1.0,2.0,3.0]])
-
- y=tf.nn.softmax(logits)
-
- y_=tf.constant([[0.0,0.0,1.0],[0.0,0.0,1.0],[0.0,0.0,1.0]])
-
- cross_entropy = -tf.reduce_sum(y_*tf.log(y))
-
- cross_entropy2=tf.reduce_sum(tf.nn.softmax_cross_entropy_with_logits(logits, y_))
-
- with tf.Session() as sess:
- softmax=sess.run(y)
- c_e = sess.run(cross_entropy)
- c_e2 = sess.run(cross_entropy2)
- print("step1:softmax result=")
- print(softmax)
- print("step2:cross_entropy result=")
- print(c_e)
- print("Function(softmax_cross_entropy_with_logits) result=")
- print(c_e2)
import tensorflow as tf
#our NN's output
logits=tf.constant([[1.0,2.0,3.0],[1.0,2.0,3.0],[1.0,2.0,3.0]])
#step1:do softmax
y=tf.nn.softmax(logits)
#true label
y_=tf.constant([[0.0,0.0,1.0],[0.0,0.0,1.0],[0.0,0.0,1.0]])
#step2:do cross_entropy
cross_entropy = -tf.reduce_sum(y_*tf.log(y))
#do cross_entropy just one step
cross_entropy2=tf.reduce_sum(tf.nn.softmax_cross_entropy_with_logits(logits, y_))#dont forget tf.reduce_sum()!!
with tf.Session() as sess:
softmax=sess.run(y)
c_e = sess.run(cross_entropy)
c_e2 = sess.run(cross_entropy2)
print("step1:softmax result=")
print(softmax)
print("step2:cross_entropy result=")
print(c_e)
print("Function(softmax_cross_entropy_with_logits) result=")
print(c_e2)
输出结果是:
- 2017-06-14 15:10:32.134178: W c:\tf_jenkins\home\workspace\release-win\device\cpu\os\windows\tensorflow\core\platform\cpu_feature_guard.cc:45] The TensorFlow library wasn't compiled to use SSE instructions, but these are available on your machine and could speed up CPU computations.
- 2017-06-14 15:10:32.134178: W c:\tf_jenkins\home\workspace\release-win\device\cpu\os\windows\tensorflow\core\platform\cpu_feature_guard.cc:45] The TensorFlow library wasn't compiled to use SSE2 instructions, but these are available on your machine and could speed up CPU computations.
- 2017-06-14 15:10:32.135179: W c:\tf_jenkins\home\workspace\release-win\device\cpu\os\windows\tensorflow\core\platform\cpu_feature_guard.cc:45] The TensorFlow library wasn't compiled to use SSE3 instructions, but these are available on your machine and could speed up CPU computations.
- 2017-06-14 15:10:32.136179: W c:\tf_jenkins\home\workspace\release-win\device\cpu\os\windows\tensorflow\core\platform\cpu_feature_guard.cc:45] The TensorFlow library wasn't compiled to use SSE4.1 instructions, but these are available on your machine and could speed up CPU computations.
- 2017-06-14 15:10:32.137179: W c:\tf_jenkins\home\workspace\release-win\device\cpu\os\windows\tensorflow\core\platform\cpu_feature_guard.cc:45] The TensorFlow library wasn't compiled to use SSE4.2 instructions, but these are available on your machine and could speed up CPU computations.
- 2017-06-14 15:10:32.137179: W c:\tf_jenkins\home\workspace\release-win\device\cpu\os\windows\tensorflow\core\platform\cpu_feature_guard.cc:45] The TensorFlow library wasn't compiled to use AVX instructions, but these are available on your machine and could speed up CPU computations.
- step1:softmax result=
- [[ 0.09003057 0.24472848 0.66524094]
- [ 0.09003057 0.24472848 0.66524094]
- [ 0.09003057 0.24472848 0.66524094]]
- step2:cross_entropy result=
- 1.22282
- Function(softmax_cross_entropy_with_logits) result=
- 1.2228
step1:softmax result=
[[ 0.09003057 0.24472848 0.66524094]
[ 0.09003057 0.24472848 0.66524094]
[ 0.09003057 0.24472848 0.66524094]]
step2:cross_entropy result=
1.22282
Function(softmax_cross_entropy_with_logits) result=
1.2228
最后大家可以试试e^1/(e^1+e^2+e^3)是不是0.09003057,发现确实一样!!这也证明了
我们的输出是符合公式逻辑的