来源:DataCastle数据城堡
TensorFlow是谷歌基于DistBelief进行研发的第二代人工智能学**系统,其命名来源于本身的运行原理。Tensor(张量)意味着N维数组,Flow(流)意味着基于数据流图的计算,TensorFlow为张量从图象的一端流动到另一端计算过程。TensorFlow是将复杂的数据结构传输至人工智能神经网中进行分析和处理过程的系统。
TensorFlow已经开源一年多了,在谷歌的支持下,TensorFlow 已成为 GitHub 上最受欢迎的机器学**开源项目。TensorFlow支持CNN、RNN和LSTM算法,这都是目前在Image,Speech和NLP最流行的深度神经网络模型。
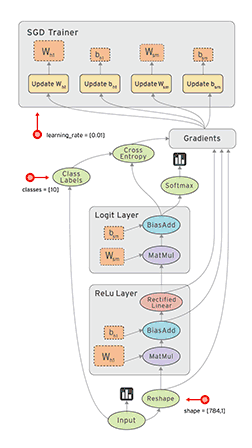
Data Flow Graph
不久前,Google Brain 工程师团队宣布在 TensorFlow 0.12 中加入初步的 Windows 支持。TensorFlow可以想象的空间非常之大,谷歌也将继续在TensorFlow的框架内继续研究,涉及到自然语言处理、机器翻译、模式识别等领域。
本文将对TensorFlow的基本使用及可以实现的案例进行介绍。
TensorFlow基础用法
1. 基本操作
import tensorflow as tf
import numpy as np
2. 乘法
a = tf.placeholder("float") # 创建符号变量
b = tf.placeholder("float")
y = tf.mul(a, b) # 乘法操作,作用在符号变量上。
sess = tf.Session() # 创建会话,计算符号变量表达式
a1 = 4
b1 = 5
print "%f + %f = %f"%(4, 5, sess.run(y, feed_dict={a: a1, b: b1})
3. 线性回归(模型:Y=W∗X+b)
# 生成训练数据 + 噪声,下面为了拟合 $$ Y = 2X $$
trX = np.linspace(-1, 1, 101)
trY = 2 * trX + np.random.randn(*trX.shape) * 0.33 # y=2x,但是加入了噪声
X = tf.placeholder("float") #输入输出符号变量
Y = tf.placeholder("float")
# 定义模型
def model(X, w):
return tf.mul(X, w) # 线性回归只需要调用乘法操作即可。
# 模型权重 W 用变量表示
w = tf.Variable(0.0, name="weights") # 共享变量
y_model = model(X, w)
# 定义损失函数
cost = (tf.pow(Y-y_model, 2)) # 平方损失函数
# 构建优化器,最小化损失函数。
train_op = tf.train.GradientDescentOptimizer(0.01).minimize(cost)
# 构建会话
sess = tf.Session()
# 初始化所有的符号共享变量
init = tf.initialize_all_variables()
# 运行会话
sess.run(init)
# 迭代训练
for i in range(100):
for (x, y) in zip(trX, trY):
sess.run(train_op, feed_dict={X: x, Y: y})
# 打印权重w
print(sess.run(w))
4. 逻辑回归(模型:y=sigmoid(X∗W+b) )
# 初始化权重w
def init_weights(shape):
return tf.Variable(tf.random_normal(shape, stddev=0.01))
# 定义模型
def model(X, w):
return tf.matmul(X, w)
# 获取mnist 数据
mnist = input_data.read_data_sets("MNIST_data/", one_hot=True)
trX, trY, teX, teY = mnist.train.images, mnist.train.labels, mnist.test.images, mnist.test.labels
# 定义占位符变量
X = tf.placeholder("float", [None, 784])
Y = tf.placeholder("float", [None, 10])
w = init_weights([784, 10])
py_x = model(X, w)
# 定义损失函数,交叉熵损失函数
cost = tf.reduce_mean(tf.nn.softmax_cross_entropy_with_logits(py_x, Y))
# 训练操作,最小化损失函数
train_op = tf.train.GradientDescentOptimizer(0.05).minimize(cost)
# 预测操作,
predict_op = tf.argmax(py_x, 1)
# 定义会话
sess = tf.Session()
init = tf.initialize_all_variables()
sess.run(init)
# 调用多次梯度下降
for i in range(100):
# 训练,每个batch,
for start, end in zip(range(0, len(trX), 128), range(128, len(trX), 128)):
sess.run(train_op, feed_dict={X: trX[start:end], Y: trY[start:end]})
# 测试,每个epoch
print i, np.mean(np.argmax(teY, axis=1) ==
sess.run(predict_op, feed_dict={X: teX, Y: teY}))
TensorFlow实现案例
https://github.com/aymericdamien/TensorFlow-Examples
这是github上的一个教程和开源项目集,涵盖了TensorFlow从入门到案例实现的各个层面的信息,足够让你从初步认识TensorFlow到自己进行项目实践。
1 - 入门
Hello World
笔记:https://github.com/aymericdamien/TensorFlow-Examples/blob/master/notebooks/1_Introduction/helloworld.ipynb
代码:https://github.com/aymericdamien/TensorFlow-Examples/blob/master/examples/1_Introduction/helloworld.py
基本操作
笔记:https://github.com/aymericdamien/TensorFlow-Examples/blob/master/notebooks/1_Introduction/basic_operations.ipynb
代码: https://github.com/aymericdamien/TensorFlow-Examples/blob/master/examples/1_Introduction/basic_operations.py
2 - 基本模型
最近邻
笔记: https://github.com/aymericdamien/TensorFlow-Examples/blob/master/notebooks/2_BasicModels/nearest_neighbor.ipynb
代码: https://github.com/aymericdamien/TensorFlow-Examples/blob/master/examples/2_BasicModels/nearest_neighbor.py
线性回归
笔记:https://github.com/aymericdamien/TensorFlow-Examples/blob/master/notebooks/2_BasicModels/linear_regression.ipynb
代码:https://github.com/aymericdamien/TensorFlow-Examples/blob/master/examples/2_BasicModels/linear_regression.py
Logistic 回归
笔记:https://github.com/aymericdamien/TensorFlow-Examples/blob/master/notebooks/2_BasicModels/logistic_regression.ipynb
代码: https://github.com/aymericdamien/TensorFlow-Examples/blob/master/examples/2_BasicModels/logistic_regression.py
3 - 神经网络
多层感知器
笔记: https://github.com/aymericdamien/TensorFlow-Examples/blob/master/notebooks/3_NeuralNetworks/multilayer_perceptron.ipynb
代码: https://github.com/aymericdamien/TensorFlow-Examples/blob/master/examples/3_NeuralNetworks/multilayer_perceptron.py
卷积神经网络
笔记: https://github.com/aymericdamien/TensorFlow-Examples/blob/master/notebooks/3_NeuralNetworks/convolutional_network.ipynb
代码:https://github.com/aymericdamien/TensorFlow-Examples/blob/master/examples/3_NeuralNetworks/convolutional_network.py
循环神经网络(LSTM)
笔记:https://github.com/aymericdamien/TensorFlow-Examples/blob/master/notebooks/3_NeuralNetworks/recurrent_network.ipynb
代码:https://github.com/aymericdamien/TensorFlow-Examples/blob/master/examples/3_NeuralNetworks/recurrent_network.py
双向循环神经网络(LSTM)
笔记: https://github.com/aymericdamien/TensorFlow-Examples/blob/master/notebooks/3_NeuralNetworks/bidirectional_rnn.ipynb
代码:https://github.com/aymericdamien/TensorFlow-Examples/blob/master/examples/3_NeuralNetworks/bidirectional_rnn.py
动态循环神经网络(LSTM)
代码: https://github.com/aymericdamien/TensorFlow-Examples/blob/master/examples/3_NeuralNetworks/dynamic_rnn.py
自编码器
笔记:https://github.com/aymericdamien/TensorFlow-Examples/blob/master/notebooks/3_NeuralNetworks/autoencoder.ipynb
代码:https://github.com/aymericdamien/TensorFlow-Examples/blob/master/examples/3_NeuralNetworks/autoencoder.py
4 - 实用技术
保存和恢复模型
笔记: https://github.com/aymericdamien/TensorFlow-Examples/blob/master/notebooks/4_Utils/save_restore_model.ipynb
代码:https://github.com/aymericdamien/TensorFlow-Examples/blob/master/examples/4_Utils/save_restore_model.py
图和损失可视化
笔记:https://github.com/aymericdamien/TensorFlow-Examples/blob/master/notebooks/4_Utils/tensorboard_basic.ipynb
代码:https://github.com/aymericdamien/TensorFlow-Examples/blob/master/examples/4_Utils/tensorboard_basic.py
Tensorboard——高级可视化
代码:https://github.com/aymericdamien/TensorFlow-Examples/blob/master/examples/4_Utils/tensorboard_advanced.py
5 - 多 GPU
多 GPU 上的基本操作
笔记:https://github.com/aymericdamien/TensorFlow-Examples/blob/master/notebooks/5_MultiGPU/multigpu_basics.ipynb
代码: https://github.com/aymericdamien/TensorFlow-Examples/blob/master/examples/5_MultiGPU/multigpu_basics.py
数据集
一些案例需要 MNIST 数据集进行训练和测试。不要担心,运行这些案例时,该数据集会被自动下载下来(使用 input_data.py)。MNIST 是一个手写数字的数据库,查看这个笔记了解关于该数据集的描述: https://github.com/aymericdamien/TensorFlow-Examples/blob/master/notebooks/0_Prerequisite/mnist_dataset_intro.ipynb
官方网站:http://yann.lecun.com/exdb/mnist/
更多案例
接下来的示例来自 TFLearn ,这是一个为 TensorFlow 提供了简化的接口的库。你可以看看,这里有很多示例和预构建的运算和层。
示例:https://github.com/tflearn/tflearn/tree/master/examples
预构建的运算和层:http://tflearn.org/doc_index/#api
教程
TFLearn 快速入门。通过一个具体的机器学**任务学** TFLearn 基础。开发和训练一个深度神经网络分类器。
笔记:
https://github.com/tflearn/tflearn/blob/master/tutorials/intro/quickstart.md
基础
线性回归,使用 TFLearn 实现线性回归:
https://github.com/tflearn/tflearn/blob/master/examples/basics/linear_regression.py
逻辑运算符。使用 TFLearn 实现逻辑运算符:
https://github.com/tflearn/tflearn/blob/master/examples/basics/logical.py
权重保持。保存和还原一个模型:
https://github.com/tflearn/tflearn/blob/master/examples/basics/weights_persistence.py
微调。在一个新任务上微调一个预训练的模型:
https://github.com/tflearn/tflearn/blob/master/examples/basics/finetuning.py
使用 HDF5。使用 HDF5 处理大型数据集:
https://github.com/tflearn/tflearn/blob/master/examples/basics/use_hdf5.py
使用 DASK。使用 DASK 处理大型数据集:
https://github.com/tflearn/tflearn/blob/master/examples/basics/use_dask.py
计算机视觉
多层感知器。一种用于 MNIST 分类任务的多层感知实现:
https://github.com/tflearn/tflearn/blob/master/examples/images/dnn.py
卷积网络(MNIST)。用于分类 MNIST 数据集的一种卷积神经网络实现:
https://github.com/tflearn/tflearn/blob/master/examples/images/convnet_mnist.py
卷积网络(CIFAR-10)。用于分类 CIFAR-10 数据集的一种卷积神经网络实现:
https://github.com/tflearn/tflearn/blob/master/examples/images/convnet_cifar10.py
网络中的网络。用于分类 CIFAR-10 数据集的 Network in Network 实现:
https://github.com/tflearn/tflearn/blob/master/examples/images/network_in_network.py
Alexnet。将 Alexnet 应用于 Oxford Flowers 17 分类任务:
https://github.com/tflearn/tflearn/blob/master/examples/images/alexnet.py
VGGNet。将 VGGNet 应用于 Oxford Flowers 17 分类任务:
https://github.com/tflearn/tflearn/blob/master/examples/images/vgg_network.py
VGGNet Finetuning (Fast Training)。使用一个预训练的 VGG 网络并将其约束到你自己的数据上,以便实现快速训练:
https://github.com/tflearn/tflearn/blob/master/examples/images/vgg_network_finetuning.py
RNN Pixels。使用 RNN(在像素的序列上)分类图像:
https://github.com/tflearn/tflearn/blob/master/examples/images/rnn_pixels.py
Highway Network。用于分类 MNIST 数据集的 Highway Network 实现:
https://github.com/tflearn/tflearn/blob/master/examples/images/highway_dnn.py
Highway Convolutional Network。用于分类 MNIST 数据集的 Highway Convolutional Network 实现:
https://github.com/tflearn/tflearn/blob/master/examples/images/convnet_highway_mnist.py
Residual Network (MNIST):
https://github.com/tflearn/tflearn/blob/master/examples/images/residual_network_mnist.py
应用于 MNIST 分类任务的一种瓶颈残差网络(bottleneck residual network):https://github.com/tflearn/tflearn/blob/master/examples/images/residual_network_mnist.py
Residual Network (CIFAR-10)。应用于 CIFAR-10 分类任务的一种残差网络:
https://github.com/tflearn/tflearn/blob/master/examples/images/residual_network_cifar10.py
Google Inception(v3)。应用于 Oxford Flowers 17 分类任务的谷歌 Inception v3 网络:
https://github.com/tflearn/tflearn/blob/master/examples/images/googlenet.py
自编码器。用于 MNIST 手写数字的自编码器:
https://github.com/tflearn/tflearn/blob/master/examples/images/autoencoder.py
自然语言处理
循环神经网络(LSTM),应用 LSTM 到 IMDB 情感数据集分类任务: https://github.com/tflearn/tflearn/blob/master/examples/nlp/lstm.py
双向 RNN(LSTM),将一个双向 LSTM 应用到 IMDB 情感数据集分类任务:
https://github.com/tflearn/tflearn/blob/master/examples/nlp/bidirectional_lstm.py
动态 RNN(LSTM),利用动态 LSTM 从 IMDB 数据集分类可变长度文本:
https://github.com/tflearn/tflearn/blob/master/examples/nlp/dynamic_lstm.py
城市名称生成,使用 LSTM 网络生成新的美国城市名:
https://github.com/tflearn/tflearn/blob/master/examples/nlp/lstm_generator_cityname.py
莎士比亚手稿生成,使用 LSTM 网络生成新的莎士比亚手稿:
https://github.com/tflearn/tflearn/blob/master/examples/nlp/lstm_generator_shakespeare.py
Seq2seq,seq2seq 循环网络的教学示例:
https://github.com/tflearn/tflearn/blob/master/examples/nlp/seq2seq_example.py
CNN Seq,应用一个 1-D 卷积网络从 IMDB 情感数据集中分类词序列:
https://github.com/tflearn/tflearn/blob/master/examples/nlp/cnn_sentence_classification.py
强化学**
Atari Pacman 1-step Q-Learning,使用 1-step Q-learning 教一台机器玩 Atari 游戏:
https://github.com/tflearn/tflearn/blob/master/examples/reinforcement_learning/atari_1step_qlearning.py
Recommender-Wide&Deep Network,推荐系统中 wide & deep 网络的教学示例:
https://github.com/tflearn/tflearn/blob/master/examples/others/recommender_wide_and_deep.py
Notebooks
Spiral Classification Problem,对斯坦福 CS231n spiral 分类难题的 TFLearn 实现:
https://github.com/tflearn/tflearn/blob/master/examples/notebooks/spiral.ipynb
可延展的 TensorFlow
层,与 TensorFlow 一起使用 TFLearn 层:
https://github.com/tflearn/tflearn/blob/master/examples/extending_tensorflow/layers.py
训练器,使用 TFLearn 训练器类训练任何 TensorFlow 图:
https://github.com/tflearn/tflearn/blob/master/examples/extending_tensorflow/layers.py
Bulit-in Ops,连同 TensorFlow 使用 TFLearn built-in 操作:
https://github.com/tflearn/tflearn/blob/master/examples/extending_tensorflow/builtin_ops.py
Summaries,连同 TensorFlow 使用 TFLearn summarizers:
https://github.com/tflearn/tflearn/blob/master/examples/extending_tensorflow/summaries.py
Variables,连同 TensorFlow 使用 TFLearn Variables:
https://github.com/tflearn/tflearn/blob/master/examples/extending_tensorflow/variables.py