Pytorch训练可视化(TensorboardX)
PyTorch 番外篇:Pytorch 中的 TensorBoard(TensorBoard in PyTorch)
TensorBoard 相关资料
TensorBoard 是 Tensorflow 官方推出的可视化工具。
官方介绍
TensorBoard: Visualizing Learning
TensorBoard 实践介绍(2017 年 TensorFlow 开发大会)
相关博客
Tensorflow 的可视化工具 Tensorboard 的初步使用
TensorFlow 教程 4 Tensorboard 可视化好帮手
PyTorch 实现
在这次的代码里,是通过简单的神经网络实现一个 MINIST 的分类器,并且通过 TensorBoard 实现训练过程的可视化。
在训练阶段,通过 scalar_summary
画出损失和精确率,通过 image_summary
可视化训练的图像。
另外,使用 histogram_summary
可视化神经网络的参数的权重和梯度值。
需要安装的 package
- tensorflow
- torch
- torchvision
- scipy
- numpy
LOG 功能实现(Logger 类)
基于 TensorBoard,给 Pytorch 的训练提供保存训练信息的接口。
Tensorboard 可以记录与展示以下数据形式:
- 标量 Scalars
- 图片 Images
- 音频 Audio
- 计算图 Graph
- 数据分布 Distribution
- 直方图 Histograms
- 嵌入向量 Embeddings
代码中实现了标量 Scalar、图片 Image、直方图 Histogram 的保存。
1
2
3
4
5
6
7
8
|
# 包
import tensorflow as tf
import numpy as np
import scipy.misc
try:
from StringIO import StringIO # Python 2.7
except ImportError:
from io import BytesIO # Python 3.x
|
1
2
3
4
5
6
7
8
9
10
11
12
13
14
15
16
17
18
19
20
21
22
23
24
25
26
27
28
29
30
31
32
33
34
35
36
37
38
39
40
41
42
43
44
45
46
47
48
49
50
51
52
53
54
55
56
57
58
59
60
61
62
63
|
class Logger(object):
def __init__(self, log_dir):
"""Create a summary writer logging to log_dir."""
# 创建一个指向log文件夹的summary writer
self.writer = tf.summary.FileWriter(log_dir)
def scalar_summary(self, tag, value, step):
"""Log a scalar variable."""
# 标量信息 日志
summary = tf.Summary(value=[tf.Summary.Value(tag=tag, simple_value=value)])
self.writer.add_summary(summary, step)
def image_summary(self, tag, images, step):
"""Log a list of images."""
# 图像信息 日志
img_summaries = []
for i, img in enumerate(images):
# Write the image to a string
try:
s = StringIO()
except:
s = BytesIO()
scipy.misc.toimage(img).save(s, format="png")
# Create an Image object
img_sum = tf.Summary.Image(encoded_image_string=s.getvalue(),
height=img.shape[0],
width=img.shape[1])
# Create a Summary value
img_summaries.append(tf.Summary.Value(tag='%s/%d' % (tag, i), image=img_sum))
# Create and write Summary
summary = tf.Summary(value=img_summaries)
self.writer.add_summary(summary, step)
def histo_summary(self, tag, values, step, bins=1000):
"""Log a histogram of the tensor of values."""
# 直方图信息 日志
# Create a histogram using numpy
counts, bin_edges = np.histogram(values, bins=bins)
# Fill the fields of the histogram proto
hist = tf.HistogramProto()
hist.min = float(np.min(values))
hist.max = float(np.max(values))
hist.num = int(np.prod(values.shape))
hist.sum = float(np.sum(values))
hist.sum_squares = float(np.sum(values**2))
# Drop the start of the first bin
bin_edges = bin_edges[1:]
# Add bin edges and counts
for edge in bin_edges:
hist.bucket_limit.append(edge)
for c in counts:
hist.bucket.append(c)
# Create and write Summary
summary = tf.Summary(value=[tf.Summary.Value(tag=tag, histo=hist)])
self.writer.add_summary(summary, step)
self.writer.flush()
|
创建模型并训练(训练过程中输出日志)
1
2
3
4
5
|
# 包
import torch
import torch.nn as nn
import torchvision
from torchvision import transforms
|
1
2
3
|
# 设备配置
torch.cuda.set_device(1) # 这句用来设置pytorch在哪块GPU上运行
device = torch.device('cuda' if torch.cuda.is_available() else 'cpu')
|
1
2
3
4
5
6
7
8
9
10
|
# MNIST 数据集
dataset = torchvision.datasets.MNIST(root='../../../data/minist',
train=True,
transform=transforms.ToTensor(),
download=True)
# Data loader
data_loader = torch.utils.data.DataLoader(dataset=dataset,
batch_size=100,
shuffle=True)
|
1
2
3
4
5
6
7
8
9
10
11
12
13
14
|
# 定义一个全连接网络(含一个隐藏层)
# Fully connected neural network with one hidden layer
class NeuralNet(nn.Module):
def __init__(self, input_size=784, hidden_size=500, num_classes=10):
super(NeuralNet, self).__init__()
self.fc1 = nn.Linear(input_size, hidden_size)
self.relu = nn.ReLU()
self.fc2 = nn.Linear(hidden_size, num_classes)
def forward(self, x):
out = self.fc1(x)
out = self.relu(out)
out = self.fc2(out)
return out
|
1
2
|
# 实例化模型
model = NeuralNet().to(device)
|
1
2
|
# 创建日志类,指定文件夹
logger = Logger('./logs')
|
1
2
3
|
# 指定损失函数和优化器
criterion = nn.CrossEntropyLoss()
optimizer = torch.optim.Adam(model.parameters(), lr=0.00001)
|
1
2
3
4
|
# 超参数
data_iter = iter(data_loader)
iter_per_epoch = len(data_loader)
total_step = 50000
|
1
2
3
4
5
6
7
8
9
10
11
12
13
14
15
16
17
18
19
20
21
22
23
24
25
26
27
28
29
30
31
32
33
34
35
36
37
38
39
40
41
42
43
44
45
46
47
48
49
50
51
52
|
# 开始训练
for step in range(total_step):
# 重置迭代器
if (step+1) % iter_per_epoch == 0:
data_iter = iter(data_loader)
# 获取图像和标签
images, labels = next(data_iter)
images, labels = images.view(images.size(0), -1).to(device), labels.to(device)
# 前向传播
outputs = model(images)
loss = criterion(outputs, labels)
# 反向传播和优化
optimizer.zero_grad()
loss.backward()
optimizer.step()
# 计算准确率
_, argmax = torch.max(outputs, 1)
accuracy = (labels == argmax.squeeze()).float().mean()
if (step+1) % 100 == 0:
print ('Step [{}/{}], Loss: {:.4f}, Acc: {:.2f}'
.format(step+1, total_step, loss.item(), accuracy.item()))
# ================================================================== #
# 该部分为保存 TensorBoard 日志信息 #
# ================================================================== #
# 1. Log scalar values (scalar summary)
# 日志输出标量信息(scalar summary)
info = { 'loss': loss.item(), 'accuracy': accuracy.item() }
for tag, value in info.items():
logger.scalar_summary(tag, value, step+1)
# 2. Log values and gradients of the parameters (histogram summary)
# 日志输出参数值和梯度(histogram summary)
for tag, value in model.named_parameters():
tag = tag.replace('.', '/')
logger.histo_summary(tag, value.data.cpu().numpy(), step+1)
logger.histo_summary(tag+'/grad', value.grad.data.cpu().numpy(), step+1)
# 3. Log training images (image summary)
# 日志输出图像(image summary)
info = { 'images': images.view(-1, 28, 28)[:10].cpu().numpy() }
for tag, images in info.items():
logger.image_summary(tag, images, step+1)
|
Step [100/50000], Loss: 2.1946, Acc: 0.44
Step [200/50000], Loss: 2.1081, Acc: 0.51
Step [300/50000], Loss: 1.9934, Acc: 0.68
Step [400/50000], Loss: 1.7980, Acc: 0.78
Step [500/50000], Loss: 1.7040, Acc: 0.71
Step [600/50000], Loss: 1.5549, Acc: 0.73
Step [700/50000], Loss: 1.4596, Acc: 0.73
Step [800/50000], Loss: 1.3418, Acc: 0.80
.....................
Step [49500/50000], Loss: 0.1180, Acc: 0.97
Step [49600/50000], Loss: 0.2404, Acc: 0.92
Step [49700/50000], Loss: 0.1864, Acc: 0.96
Step [49800/50000], Loss: 0.0704, Acc: 1.00
Step [49900/50000], Loss: 0.0792, Acc: 0.98
Step [50000/50000], Loss: 0.1406, Acc: 0.96
调用 TensorBoard 进行可视化
经过训练后,日志信息保存在./logs
文件夹下。运行命令进行可视化,
1
|
$ tensorboard --logdir='./logs' --port=6006
|
然后打开本地浏览器,打开 http://localhost:6006/
就能看到了。
标量 Scalar
标量 Scalar
图片 Image
正在上传…重新上传取消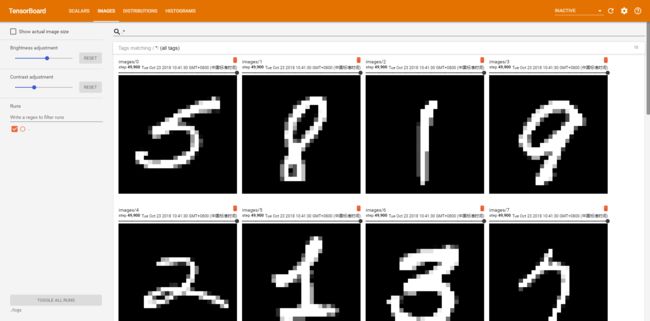
图片 Image
直方图 Histogram
直方图 Histogram