%UKF无味卡尔曼滤波在自动驾驶车辆定位中的应用
%参考文献:
% The High-Degree Cubature Kalman Filter (Bin Jia, Ming Xin, and Yang Cheng)
% Unscented Kalman Filter Tutorial (Gabriel A. Terejanu )
% 基于正交变换的五阶容积卡尔曼滤波导航算法 (何康辉,董朝阳 )
% EKF、UKF和CKF的滤波性能对比研究 (常宇健,赵辰)
% 组合导航原理与应用 (西北工业大学出版社)
% 卡尔曼滤波与Matlab仿真 (北京航空航天大学出版社)
% 本例子使用线性状态模型,因此加速度变成了干扰项
% Lidar传感器测量模型为线性模型,Radar传感器测模型为非线性模型
% 在Radar传感器中使用滤波算然为 UKF,在Lidar传感器中使用滤波算然为 KF
clear;
clc;
tic; %计时
dt = 0.1; %步长
%分配空间
Radar_measurement = [];
Radar_measurement_p = [];
Lidar_measurement = [];
UKF_estimation = [];
ukf = []; % 结构体
%读取Radar_Lidar_Data2.csv文件保存到矩阵Data
Data = csvread('Radar_Lidar_Data1.csv',1,1);%起点为第2行第2列 612组数据
%将Data矩阵数据保存到data.csv文件中
csvwrite('Data.csv',Data);
%初始化状态,x是4维向量
if (Data(1,1) == 1)
x = [Data(1,2); Data(1,3); 0; 0]; %如果是lidar测量数据,文件 Dada2.csv,保存这个
else
x = [
Data(1,2)*cos(Data(1,3));
Data(1,2)*sin(Data(1,3));
Data(1,4)*cos(Data(1,3));
Data(1,4)*sin(Data(1,3))
]; %如果是Radar测量数据,文件 Dada1.csv,保存这个
end
%初始化状态协方差矩阵
P = diag([1,1,1000,1000]);
%状态转移矩阵,线性
F = [[1, 0, dt, 0];
[0, 1, 0, dt];
[0, 0, 1, 0];
[0, 0, 0, 1]];
%系统误差协方差矩阵
Q = [[(dt^2)/4,0,(dt^3)/2,0];
[0,(dt^2)/4,0,(dt^3)/2];
[(dt^3/2),0,(dt^2),0];
[0,(dt^3)/2,0,(dt^2)]];
%Lidar传感器观测矩阵,线性
H_L = [[1, 0, 0, 0];
[0, 1, 0, 0]];
%雷达传感器测量误差协方差矩阵
R_r = diag([0.09,0.005,0.09]);
%激光雷达传感器测量误差协方差矩阵
R_L = diag([0.0025, 0.0025]);
%4阶单位矩阵
I = eye(4);
%%%%%%%%%%%%%%%%%%%%%%%%%%%%%%%% UKF参数设置 %%%%%%%%%%%%%%%%%%%%%%%%%%%%%%%%%%%
L = numel(x); %numer of states
alpha = 0.001; %对于高斯分布来说,取值为 (0,1)
ki = -1; %对于高斯分布来说,取值为 3-n
beita = 2; %对于高斯分布来说,取值为2
lambda = alpha^2*(L+ki)-L; %表示sigma点与均值的距离
Wm = [lambda/(L+lambda),1/(2*(L+lambda)) * ones(1,2*L)]; %sigma点对期望权重
Wc = [(lambda/(L+lambda) + 1 - alpha^2 + beita),1/(2*(L+lambda)) * ones(1,2*L)]; %sigma点对方差权重
gama = sqrt(L+lambda);
% alpha 越大,第一个sigma 点(平均值处)的权重越大,其他点权重越小
%%%%%%%%%%%%%%%%%%%%%%%%%%%%%%%%% 开始迭代 %%%%%%%%%%%%%%%%%%%%%%%%%%%%%%%%%%%
for n = 1:length(Data)
%Radar传感器
%非线性
if (Data(n,1) == 2)
%Radar测量数据
z = transpose(Data(n,2:4));
z_p = [ z(1) * cos(z(2));z(1) * sin(z(2));z(3) * cos(z(2));z(3) * sin(z(2))];
%UKF 算法核心部分
%======================================================================%
%1.采样:由x0,P0产生sigma点
A1 = gama * chol(P);
Y1 = x(:,ones(1,numel(x)));
X1_sigma = [x,Y1 + A1,Y1 - A1];
%2.UT变换:sigma点经过线性映射
cols = size(X1_sigma,2); % 返回矩阵列数
x1_mean = zeros(L,1); % 加权均值:经过线性映射后均值
X1_ut = zeros(L,cols); % UT变换:sigma点经过线性映射
for k = 1 : cols
X1_ut(:,k) = F * X1_sigma(:,k); % UT变换:sigma点经过线性映射
x1_mean = x1_mean + Wm(k) * X1_ut(:,k); % 加权均值:经过线性映射后均值 2n+1
end
error1 = X1_ut - x1_mean(:,ones(1,cols)); % 经过线性映射后偏差
P1_cov = error1 * diag(Wc) * transpose(error1) + Q; % 加权方差:经过线性映射后方差
%3.采样:计算增广sigma点
A2 = gama * chol(P1_cov);
Y2 = x1_mean(:,ones(1,numel(x1_mean)));
X2_sigma = [x1_mean,Y2 + A2,Y2 - A2];
%4.非线性测量方程
h = @(x)[sqrt(x(1)^2 + x(2)^2);
atan(x(2)/x(1));
(x(1)*x(3) + x(2)*x(4)) / sqrt(x(1)^2 + x(2)^2)];
%5.UT变换:sigma点经过非线性映射
x2_mean = zeros(L-1,1); % 加权均值:经过非线性映射后均值 3x1
X2_ut = zeros(L-1,cols); % UT变换:sigma点经过非线性映射 3x9
for k = 1 : cols
X2_ut(:,k) = h(X2_sigma(:,k)); % UT变换:sigma点经过非线性映射 3x9
x2_mean= x2_mean + Wm(k) * X2_ut(:,k); % 加权均值:经过非线性映射后均值 3x1
end
error2 = X2_ut - x2_mean(:,ones(1,cols)); % 经过非线性映射后偏差 3x3
P2_cov = error2 * diag(Wc) * transpose(error2) + R_r; % 加权方差:经过非线性映射后方差 3x3
%6.量测更新
P3_cov = error1 * diag(Wc) * transpose(error2); % 方差cross 4x3
K = P3_cov / P2_cov; % 卡尔曼增益 4x3
x = x1_mean + K * (z - x2_mean); % 状态均值更新
P = P1_cov - K * P2_cov * transpose(K); % 状态方差更新
%7.保存数据
UKF_estimation = [UKF_estimation;transpose(x)];
Radar_measurement = [Radar_measurement; transpose(z)];
Radar_measurement_p = [Radar_measurement_p;transpose(z_p)];
%======================================================================%
%Lidar传感器
%线性
else
%KF 算法核心部分
%======================================================================%
%1.预测更新状态、状态协方差阵
x = F * x;
P = F * P * transpose(F) + Q;
%2.Lidar测量数据
z = transpose(Data(n,2:3));
%3.测量更新
%更新卡尔曼增益矩阵
y = z - (H_L * x);
S = H_L * P * transpose(H_L) + R_L;
K = P * transpose(H_L) / S;
x = x + (K * y); % 更新状态
P = (I - (K * H_L)) * P; % 更新状态协方差阵
%4.保存状态值
UKF_estimation = [UKF_estimation;transpose(x)];
Lidar_measurement = [Lidar_measurement; transpose(z)];
%======================================================================%
end
end
%%%%%%%%%%%%%%%%%%%%%%%%%%%%%%% 数据可视化%%%%%%%%%%%%%%%%%%%%%%%%%%%%%%%%%%
figure(1);
hold on;
plot(Data(:,5),Data(:,6),'k'); %真实状态
scatter(UKF_estimation(:,1),UKF_estimation(:,2),5,'filled','r'); %估计状态
scatter(Lidar_measurement(:,1),Lidar_measurement(:,2),5,'filled','blue'); %Lidar传感器测量
scatter(Radar_measurement_p(:,1),Radar_measurement_p(:,2),5,'filled','g'); %Radar传感器测量
legend('truth','UKF Path result','Lidar Measurement','Radar Measurement'); %标注
title('位置估计');
axis square;
grid on;
hold off;
%位置x误差
figure(2);
hold on;
UKF_x_error = Data(1:2:1223,5) - UKF_estimation(1:2:1223,1); %位置x误差
plot(1:612,UKF_x_error);
plot(1:612,Data(1:2:1223,5) - Radar_measurement_p(:,1));
legend('UKFerror','Radarerror');
title('位置x误差');
grid on;
hold off;
%位置y误差
figure(3);
hold on;
UKF_y_error = Data(1:2:1223,6) - UKF_estimation(1:2:1223,2); %位置y误差
plot(1:612,UKF_y_error);
plot(1:612,Data(1:2:1223,6) - Radar_measurement_p(:,2));
legend('UKFerror','Radarerror');
title('位置y误差');
grid on
hold off;
%速度x误差
figure(4);
hold on;
UKF_vx_error = Data(1:2:1223,7) - UKF_estimation(1:2:1223,3); %速度x误差
plot(1:612,UKF_vx_error);
plot(1:612,Data(1:2:1223,7) - Radar_measurement_p(:,3));
legend('UKFerror','Radarerror');
title('速度x误差');
grid on
hold off;
%速度y误差
figure(5);
hold on;
UKF_vy_error = Data(1:2:1223,8) - UKF_estimation(1:2:1223,4); %速度y误差
plot(1:612,UKF_vy_error);
plot(1:612,Data(1:2:1223,8) - Radar_measurement_p(:,4));
legend('UKFerror','Radarerror');
title('速度y误差');
grid on
hold off;
%将数据保存到 UKF_error.csv 文件中,用于软法比较
UKF_error = [UKF_x_error,UKF_y_error,UKF_vx_error,UKF_vy_error];
csvwrite('UKF_error.csv',UKF_error);
%保存到结构体
ukf.estimation = UKF_estimation;
ukf.error = UKF_error;
ukf.measurement_R = Radar_measurement_p;
ukf.measurement_L = Lidar_measurement;
%结束计时
toc;
结果分析:

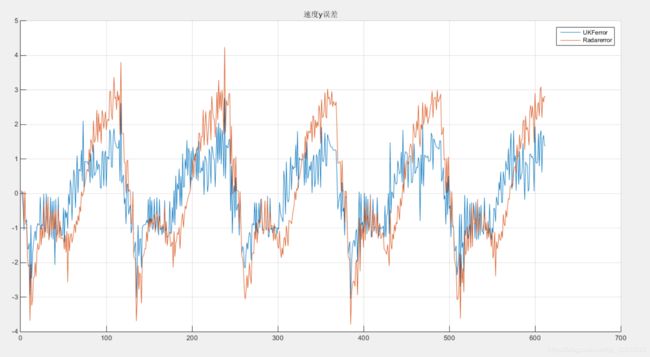
