[标题]
《Relational Graph Attention Network for Aspect-based Sentiment Analysis》
[代码地址]
https://github.com/shenwzh3/RGAT-ABSA
[知识储备]
目录
- 一、背景与概览
-
- 1.1 相关研究
- 1.2 贡献点
- 1.3 相关工作
- 二、模型
-
- 2.1 attention还是syntax
- 2.2 以aspect为方向的依赖树
- 2.3 GAT(Graph Attention Network)
- 2.4 RGAT(Relational Graph Attention Network)
- 2.5 train object
- 三、实验与评估
- 四、结论与个人总结
- 五、个人参考
- 六、bert
一、背景与概览
1.1 相关研究
"great food but the service was dreadful"一句话中,"food"和"service"是aspects,"great"和"dreadful"是opinion words。如何把这两者connect起来,是任务的核心。
1.2 贡献点
- 发现"任务相关的语法结构"能够解决GNN的问题,并提出了一种新的面向aspects的依赖树结构(三步)
- 使用外部的parser得到dependency tree
- 把一个目标aspect作为root
- 对树进行修剪,只保留与aspects有直接依赖关系的边
- 提出GAT用于编码dependency tree
1.3 相关工作
- attention mechanism
“So delicious was the noodles but terrible vegetables”,对于这样的一句话,terrible这个opinion words会比delicious更接近noodles这个aspects,进而获得更高的权重、产生错误。
- hand-crafted syntactic rules
受到各种限制
- Dependency-based parse trees、
[在给定parse trees的情况下],利用递归神经网络对整个依赖树从叶到根进行编码,或者计算内部节点之间的句子用于attention weight decay。
- GNN
使用GNN来从依赖树中学习到表示有三个缺点:1. 表明aspects和opinion words之间的依赖关系被忽略了;2. 根据经验,只有解析树的一小部分与此任务有关,没有必要对整个树进行编码 3. 编码过程是依赖于树的,这使得在优化时批处理操作不方便!!!
二、模型
2.1 attention还是syntax
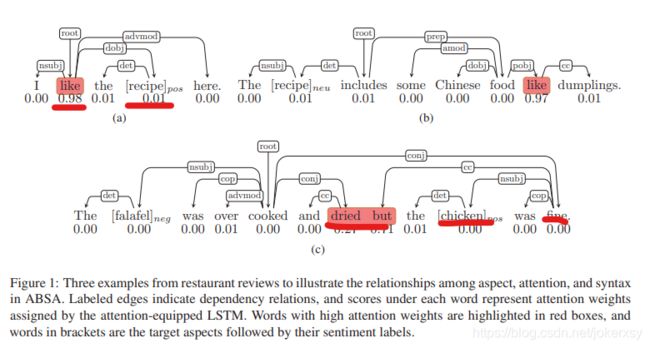
如上图所示,基于attention的序列模型,在(b)中错误的把"like"这个介词赋予了很高的权重;在©中错误的把"dried but"赋予了很高的权重。而如果直接基于句子的语法结构,问题则得到解决。
2.2 以aspect为方向的依赖树
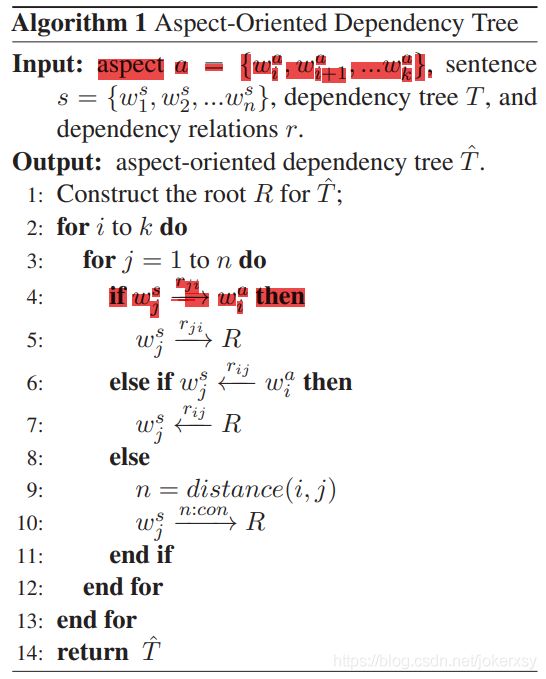
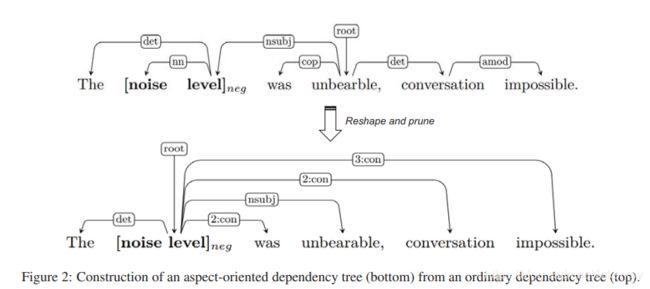
大致分为四个步骤:
- 使用外部dependency parser得到dependency tree
- 把target aspect作为root
- 保留所有原先与root这个词(也有可能是一个span,如上图的[noise level])相连的边
- 舍弃所有原先与root不相连的边
两个不懂的地方:
- 转化过的tree中,"the"和"unbearable"对于"noise level"的方向
- "noise level"对于"was"的distance
两个注意的地方:
- 对于多个aspect的句子,单独建树
- distance=∞ if distance > 4
2.3 GAT(Graph Attention Network)
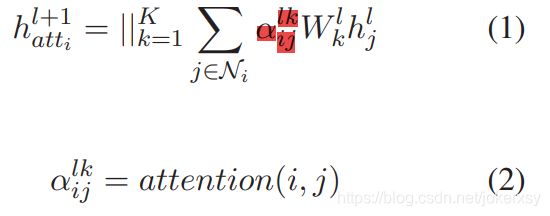
其中的attention代表点积。
GAT沿着依赖的路径聚合了邻接节点,但没有把依赖关系本身考虑进来。
2.4 RGAT(Relational Graph Attention Network)
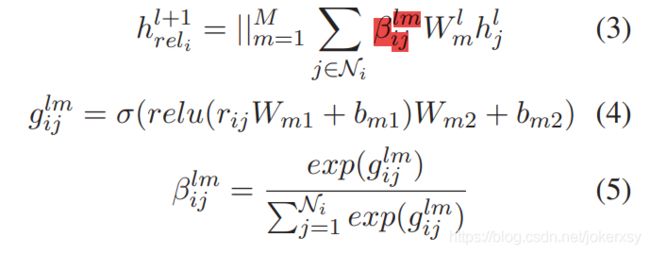
额外增加relational heads。
2.5 train object

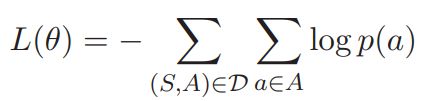
h a l h_a^l hal是第l层R-GAT,aspect节点的输出。
三、实验与评估
- 使用Biaffine Parser
- dependency relation embeddings 为300维
- word embedding 为300维glove
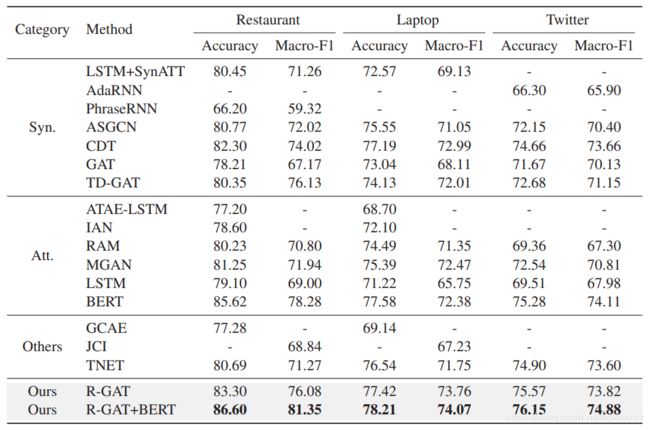
四、结论与个人总结
不是很懂
五、个人参考
https://www.zhihu.com/question/54504471/answer/611222866
https://www.zhihu.com/question/275866887
六、bert
如果使用bert,单词会被tokenize成子词,那么如何得到单词的embedding呢?
feature = torch.stack([torch.index_select(f, 0, w_i)
for f, w_i in zip(feature_output, word_indexer)])
这篇的做法是:用子词段中的第一个子词的向量来作为这个单词的向量表示。