import numpy as np
import matplotlib.pylab as plt
from sklearn.cluster import KMeans
from sklearn.metrics import pairwise_distances_argmin
from skimage.io import imread
from sklearn.utils import shuffle
from skimage import img_as_float
from time import time
pepper = imread('./9781789343731_Code/images/pepper.jpg')
# plt.figure(1)
# plt.clf()
# # ax = plt.axis([0, 0, 1, 1])
# plt.axis('off')
# plt.title('Original image %d colors' % (len(np.unique(pepper))))
# plt.imshow(pepper)
# plt.show()
n_colors = 64
pepper = np.array(pepper, dtype=np.float64) / 255
w, h, d = original_shape = tuple(pepper.shape)
assert d == 3
image_array = np.reshape(pepper, (w*h, d))
def recreate_image(codebook, labels, w, h):
d = codebook.shape[1]
image = np.zeros((w, h, d))
label_idx = 0
for i in range(w):
for j in range(h):
image[i][j] = codebook[labels[label_idx]]
label_idx += 1
return image
# plt.figure(1)
# plt.clf()
# plt.axis('off')
# plt.title('Original image (96, 615, colors)')
# plt.imshow(pepper)
plt.figure(2, figsize=(20, 20))
plt.clf()
i = 1
for k in [64, 32, 16, 4]:
t0 = time()
plt.subplot(4,2,i)
plt.axis('off')
image_array_sample = shuffle(image_array, random_state=0)[:1000]
kmeans = KMeans(n_clusters=k, random_state=0).fit(image_array_sample)
print('done in %0.3fs.' % (time() - t0))
print('Predicting color indices on the full image (k-means)')
t0 = time()
labels = kmeans.predict(image_array)
print('done in %0.3fs.' % (time() - t0))
plt.title('Quantized image (' + str(k) + ' colors, kmeans)')
plt.imshow(recreate_image(kmeans.cluster_centers_, labels, w, h))
i += 1
# plt.show()
# plt.figure(3, figsize=(10, 10))
# plt.clf()
i = 1
for k in [64, 32, 16, 4]:
t0 = time()
plt.subplot(4, 2, 4+i)
plt.axis('off')
codebook_random = shuffle(image_array, random_state=0)[:k+1]
print('done in %0.3fs.' % (time() - t0))
print('Predicted color indices on the full image random')
t0 = time()
labels_random = pairwise_distances_argmin(codebook_random, image_array, axis=0)
print('done in %0.3fs.' % (time() - t0))
plt.title('Quantized image ('+str(k) +'colors, random)')
plt.imshow(recreate_image(codebook_random, labels_random, w, h))
i += 1
plt.show()
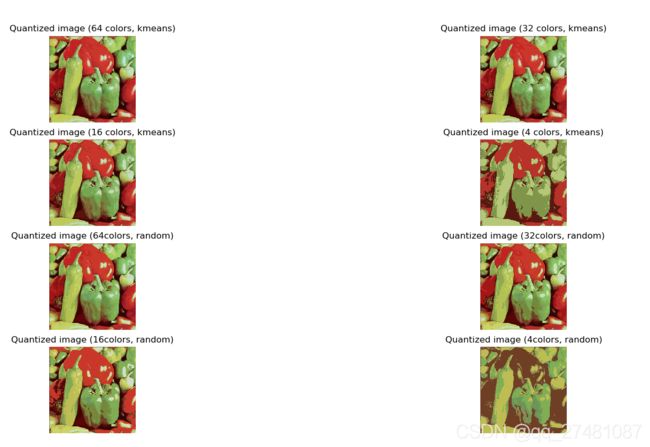