原图
模板
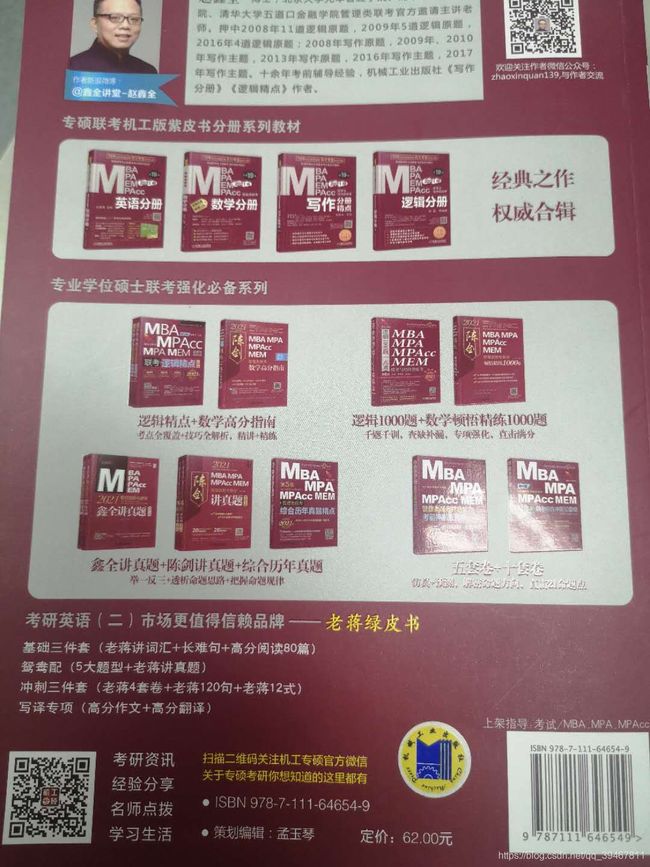
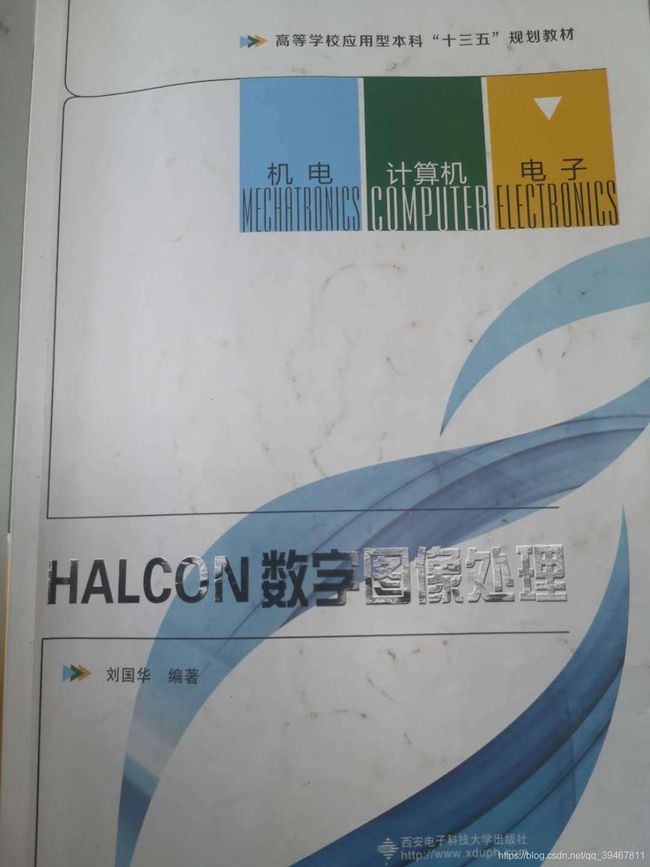
识别图
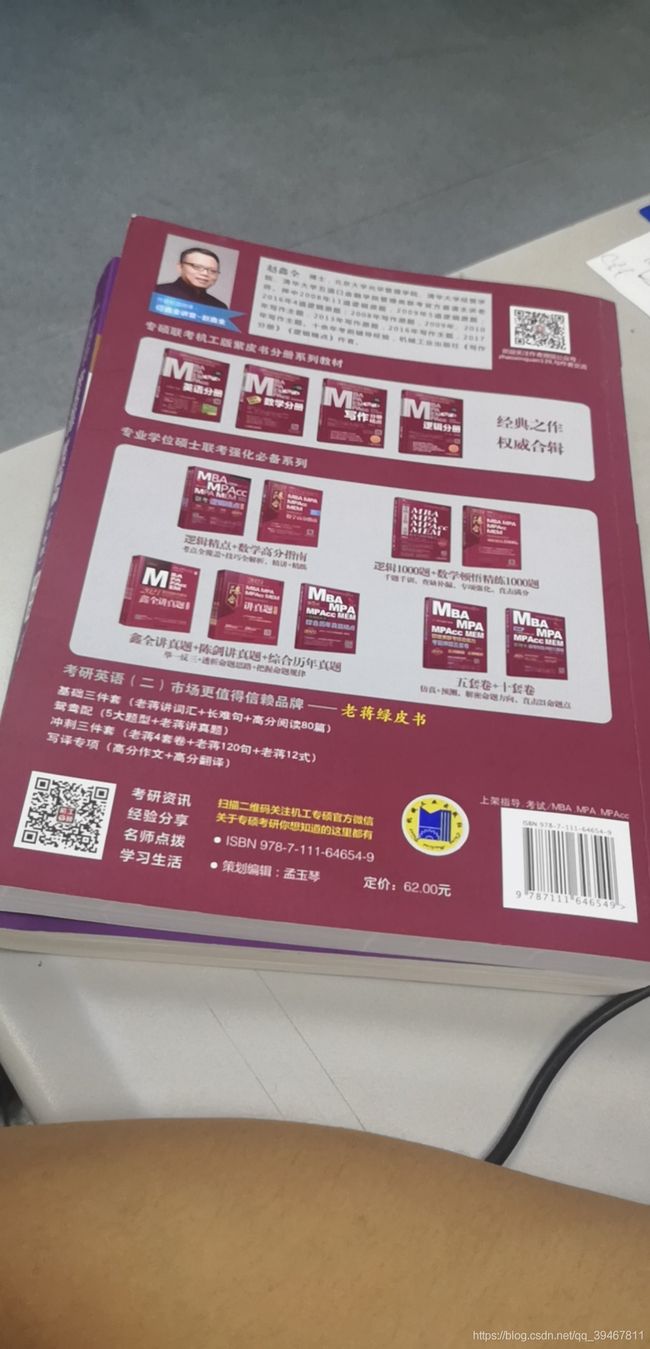
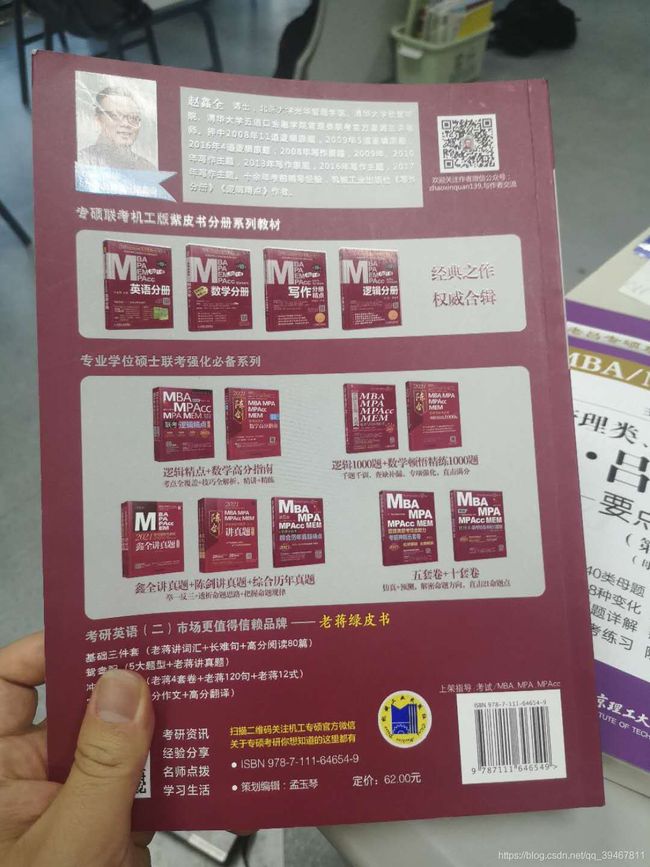
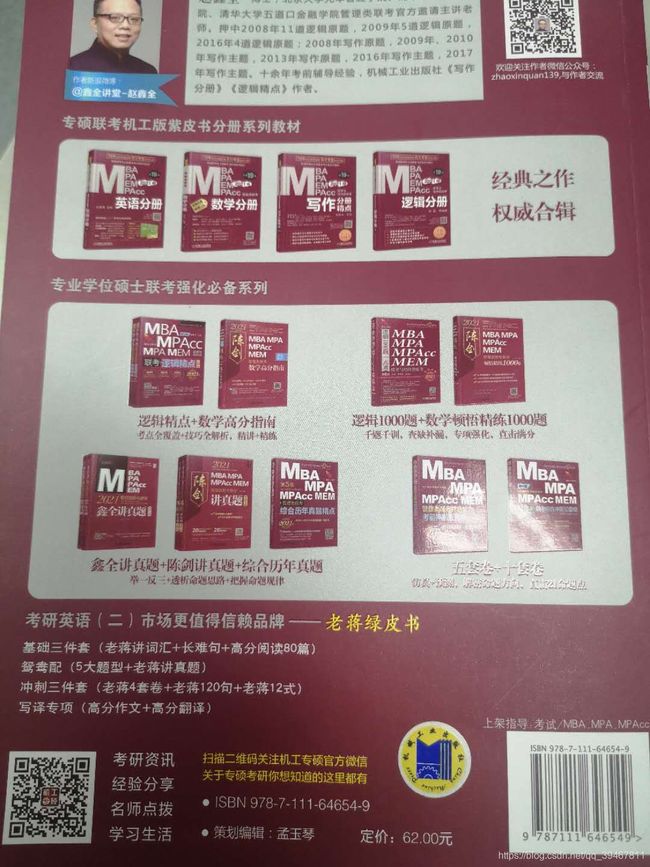
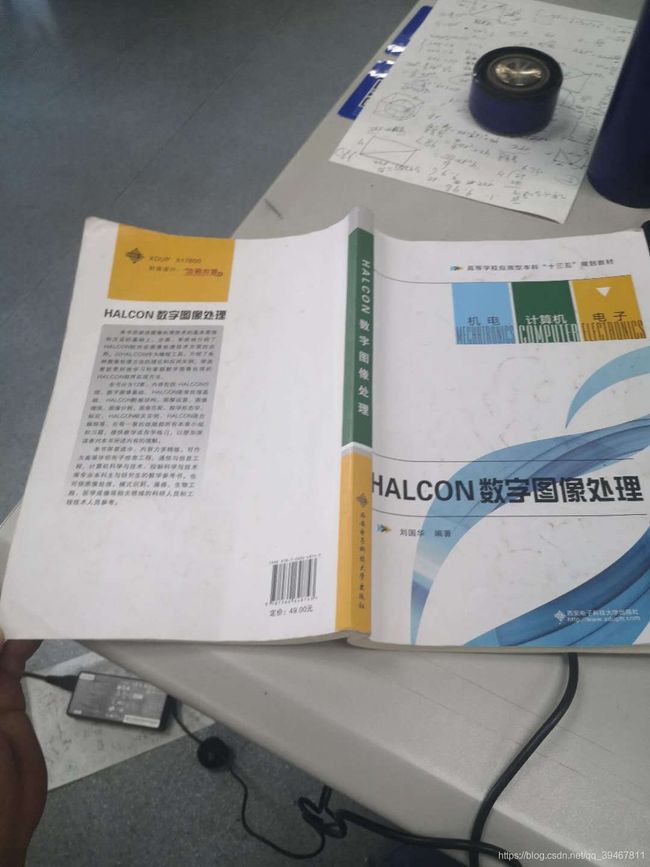
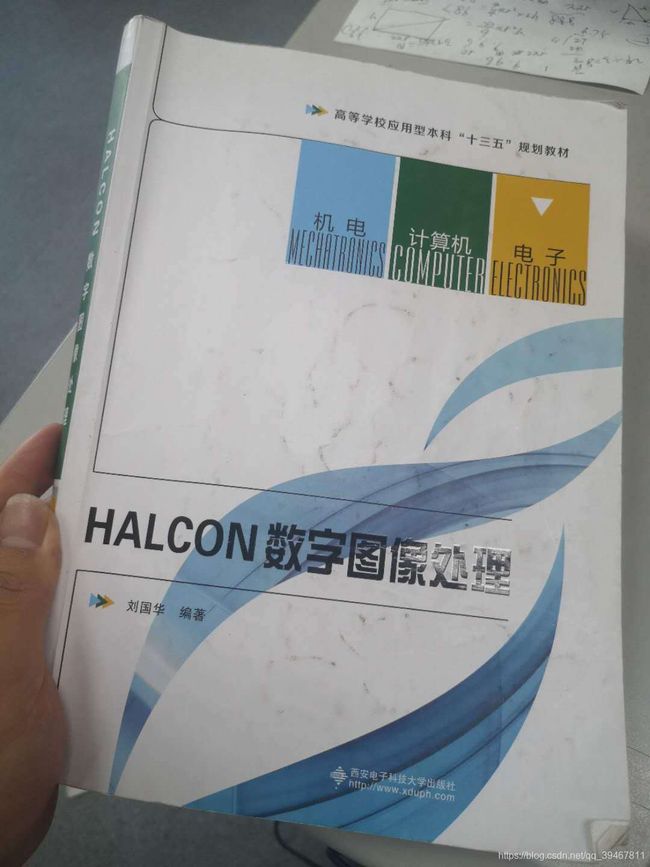
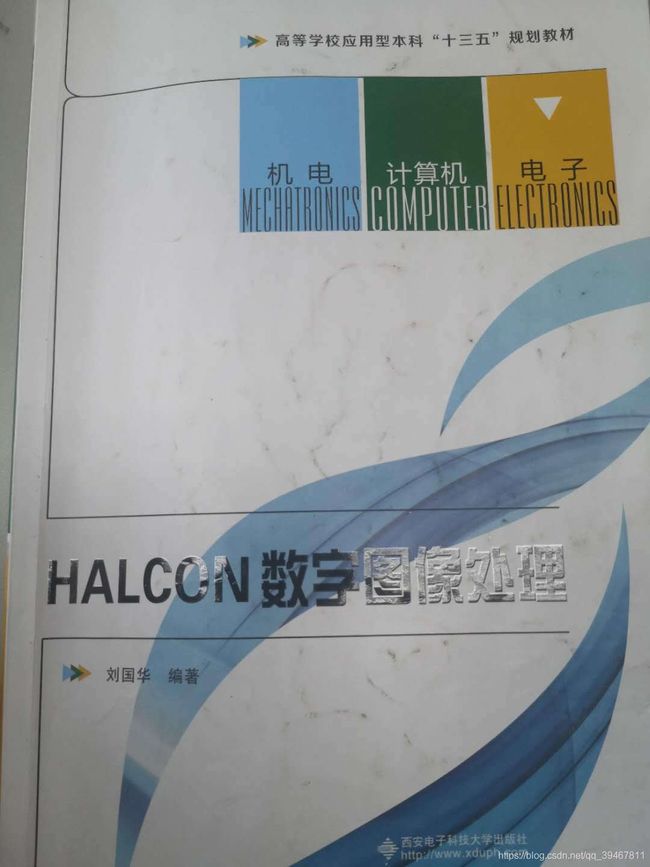
代码
*这个例子在图片数据库中查找文章的页面。
*第一步是训练不同的页面并创建模型。
*然后搜索未知图像并检测出正确的文章页面。
*请注意,这个例子需要一些内存来训练模型。
dev_update_off ()
dev_close_window ()
read_image (Image, 'book/1')
get_image_size (Image, Width, Height)
dev_open_window_fit_image (Image, 0, 0, -1, -1, WindowHandle)
set_display_font (WindowHandle, 14, 'mono', 'true', 'false')
dev_set_draw ('margin')
dev_display (Image)
*定义需要使用变量
ModelIDs := []
ModelsFound := 0
NumPoints := []
NumModels := 2
TotalTime := 0
*
* Create region for visualization purpose.
*生成需要处理区域矩形
RowRoi := [10,10,Height - 10,Height - 10]
ColRoi := [10,Width - 10,Width - 10,10]
gen_rectangle1 (Rectangle, 10, 10, Height - 10, Width - 10)
disp_message (WindowHandle, ['Press \'Run\' to start model creation ...','(may take a few minutes)'], 'window', 10, 10, 'black', 'true')
disp_continue_message (WindowHandle, 'black', 'true')
stop ()
*
* For every page the descriptor model is created.
for Index := 1 to NumModels by 1
read_image (Image, 'book/' + Index)
rgb1_to_gray (Image, ImageGray)
get_image_size (ImageGray, Width, Height)
*截取需要处理的区域
reduce_domain (ImageGray, Rectangle, ImageReduced)
dev_clear_window ()
dev_display (ImageGray)
disp_message (WindowHandle, 'Creating model no. ' + Index + '/' + NumModels + ' ... please wait.', 'window', 10, 10, 'black', 'true')
*
* Create the descriptor model with default parameters (except scaling)
* For a fast detection, the harris binomial point detector is chosen.
*运算符create_uncalib_descriptor_model准备图像区域的描述符模型,该模型在图像模板中传递,可用于基于描述符的匹配。
*通过随后调用find_uncalib_descriptor_model,可以获得从模板到搜索图像的投影2D变换(单应性)。
*模板中区域的重心作为模型的原点。与create_calib_descriptor_model相比,不需要校准相机,因此后续匹配的结果是2D投影。
*请注意,在模板图像中可见的对象部分必须是平面的。
*create_uncalib_descriptor_model(Template : : DetectorType, DetectorParamName, DetectorParamValue,
* DescriptorParamName, DescriptorParamValue, Seed : ModelID)
*描述符模型描述了一组感兴趣的点。它存储了它们的位置和对其局部灰度邻域的区分性描述。
*兴趣点提取由DetectorType、DetectorParamName和DetectorParamValue参数化。
*兴趣点周围的相应描述符由DescriptorParamName和DescriptorParamValue参数化。
*Seed种子随机数生成器,在构造描述符时使用。
*返回的ModelID是对生成的描述符模型的引用。该模型可以有效地检测学习模板的实例,并允许模型和搜索图像之间的透视变换。
*由于基于描述子的匹配依赖于稳定的、有区别的兴趣点的存在,因此需要对待检测对象进行纹理化处理,但不能重复。
** Detector parameters
*如前所述,检测器用于提取图像中稳定的兴趣点。通过参数DetectorType,可以选择要使用的兴趣点运算符。
*目前,支持points_lepetit、points_harris及其二项近似点points_harris_binordinary('lepetit','harris','harris_binormal')。
*对于低对比度的模板或搜索图像,应使用harris点运算符之一。
*根据所选的DetectorType,可以在DetectorParamName和DetectorParamValue中设置适当的参数名称和值。
*DetectorParamName的可能参数名称和相应的默认值为:
* 'lepetit':
* ['radius','check_neighbor', 'min_check_neighbor_diff','min_score','subpix']
*[3, 1, 15, 30, 'interpolation']
*'harris':
*['sigma_grad','sigma_smooth','alpha', 'threshold']
*[ 0.7, 2.0, 0.08, 1000]
*'harris_binomial':
*['mask_size_grd','mask_size_smooth', 'alpha','threshold','subpix']
*[ 5, 15, 0.08, 1000, 'on']
*Descriptor parameters 描述符参数
*有关这些参数含义的更多详细信息,请分别在points_harris、points_harris_binordinary和points_lepetit中找到。
*如果传递了空元组或DetectorParamName中未提供参数,则采用上述默认值。
*在调整算子参数的同时,应针对性地提取一组50到450个特征点(取决于模板的纹理和大小),这些特征点均匀分布在模板的ROI上。
*因此,建议事先运行所选的点运算符,并通过gen_cross_contour_xld可视化结果。在大多数情况下,使用默认设置就足够了。
*点描述子是一种分类器,它为兴趣点建立灰度邻域的特征描述。
*目前,描述符是用所谓的随机化蕨类植物来实现的,它可以学习在兴趣点周围区域的随机位置确定的成对像素的灰度差的极性。
*在该模型中寻找匹配点(find_uncalib_descriptor_model)的特征点。
*描述符只需要存储投射稳定的兴趣点(这些兴趣点将出现在模板的许多投影视图中)。
*为了评估兴趣点的稳定性,我们进行了一个模拟:模板经过多次仿射变换,在大多数视图中可以提取的点被认为是稳定的。
*仿射变换是兴趣点局部邻域内射影变换的一个很好的近似。
*可以使用DescriptorParamName和DescriptorParamValue设置以下描述符参数:
***描述符大小参数:
*'depth':
*分类蕨类植物的深度。更深入的随机蕨类植物可以更好地区分兴趣点。然而,蕨类植物的内存需求增长了2倍,达到了“深度”的幂次。典型值为[5。。11] ,默认值为11。
*'number_ferns':
*所用蕨类植物结构的数量。使用更多的蕨类植物提高了识别的鲁棒性,但也增加了匹配的运行时间。如果描述符所需的内存应该很小,则应使用许多深度较小的蕨类植物(例如,“number_ferns”=150,“depth”=5)
*如果探测速度更为重要,则应使用较少深度较大的蕨类植物(例如,“数量蕨类”=10,“深度”=11)。典型值为[1。。150],默认值为30。
*'patch_size':
*描述兴趣点的二次邻域的边长。此参数的值太大会影响运行时。典型值为[15。。33],默认值为17。
* 总之,参数'depth'、'number_ferns'和'patch_size'允许对检测鲁棒性、速度和内存消耗进行透明控制。
****Simulation parameters:
*'tilt':
*在模拟阶段打开或关闭投影变换。当开关打开时,模型的鲁棒性得到了提高,并且可以发现倾斜较大的物体。当关闭时,训练时间可以大大减少,并且模型仍然能够识别射影不变的物体。
*可能的值为['on'、'off'],默认值为'on'。
*'min_rot':
*围绕模板法向量的最小旋转角度。典型值为[-180。。0],默认值为-180。
*'max_rot':
*模板法向量的最大旋转角。典型值为[0。。180],默认值为180。
*'min_scale':
*模板的最小比例。典型值为[0.1。。1.0],默认值为0.5。
*'max_scale':
*模板的最大尺度。典型值为[1.0。。3.5],默认值为1.4。
**参数“min_rot”、“max_rot”、“min_scale”和“max_scale”允许手动设置模板的哪些仿射变换视图用于训练描述符。
*设置这些参数有助于减少训练时间,尤其是与“倾斜”参数结合使用时。
*请注意,这些参数对find_uncalib_descriptor_model的结果有直接影响,因此必须谨慎设置。
*例如,如果旋转范围被限制在“min_rot”=-10到“max_rot”=10,则无法找到旋转角度超出该范围的模板视图。
*有限的训练范围需要较少的蕨类植物/蕨类植物的深度来找到模板。
*在这种情况下,蕨类植物的数量和深度可以进一步减少,从而优化了模型。
count_seconds (Seconds1)
*1、生成图像区域的描述符模型
create_uncalib_descriptor_model (ImageReduced, 'harris_binomial', [], [], ['min_rot','max_rot','min_scale','max_scale'], [-90,90,0.2,1.1], 30, ModelID)
count_seconds (Seconds2)
TotalTime := TotalTime + (Seconds2 - Seconds1)
*
* For the correct projection of the rectangles in a later step the origin
* of the model has to be set to the image origin
***set_descriptor_model_origin( : : ModelID, Row, Column : )
*为descriptor 模型设置原点(参照点)
*描述:参照点 通常和创建模型时候(create_uncalib_descriptor_model, orcreate_calib_descriptor_model)使用的region输入的重力中心点相关,参数的设定即表示相对于重力中心点的位移,eg:一个原点是(-20,-10)表示这个原点在重力中心点的左上角。
* 在设定了参照点之后,使用find_uncalib_descriptor_modeland find_calib_descriptor_model来搜索模型的形态和转换信息
*注意:这里设置的参照点是属于模型的一部分,是模型的一个属性get_descriptor_model_points( : : ModelID, Set, Subset : Row, Column)
*Set可以控制是取出模型中的兴趣点还是上一次搜索的图片中的兴趣点,
*subset表示取出几个点,默认为‘all'(这里用all也会比较慢,可以考虑选取其他数值),后两个参数是输出,保存了这些点的信息。
*Subset : 每个如果是搜索的话,每个正确的匹配的兴趣点是所有兴趣点的一个子集
*3、获取模型中的兴趣点
get_descriptor_model_points (ModelID, 'model', 'all', Row_D, Col_D)
NumPoints := [NumPoints,|Row_D|]
write_descriptor_model (ModelID, Index+'.dsm')
endfor
*
* Model creation finished.
dev_display (ImageGray)
disp_message (WindowHandle, NumModels + ' models created in ' + TotalTime$'.4' + ' seconds.', 'window', 10, 10, 'black', 'true')
disp_continue_message (WindowHandle, 'black', 'true')
stop ()
read_image (Image, 'book/1')
*再次初始化窗口,因为图像大小已更改。
dev_resize_window_fit_image (Image, 0, 0, -1, -1)
set_display_font (WindowHandle, 14, 'mono', 'true', 'false')
*
* Main loop:
* Search the models in all images
*对6张图进行遍历,找出图中对应的匹配项
for Index1 := 1 to 6 by 1
OutputString := []
NumMsgs := 0
ModelsFound := 0
TotalTime := 0
read_image (Image, 'book/' + Index1)
rgb1_to_gray (Image, ImageGray)
dev_display (Image)
disp_message (WindowHandle, 'Searching image ...', 'window', 10, 10, 'black', 'true')
*
* Search every model in each image
*对每张图像进行所有模型的遍历搜索
for Index2 := 0 to |ModelIDs| - 1 by 1
read_descriptor_model ((Index2+1)+'.dsm' ,ModelID1)
ModelIDs[Index2] := ModelID1
*
* Find model (using default parameters)
count_seconds (Seconds1)
*4、在图像中查找描述符模型的最佳匹配项。
find_uncalib_descriptor_model (ImageGray, ModelIDs[Index2], [], [], ['min_score_descr','guided_matching'], [0.003,'on'], 0.25, 1, 'num_points', HomMat2D, Score)
*** find_uncalib_descriptor_model(Image : : ModelID, DetectorParamName, DetectorParamValue,
* DescriptorParamName, DescriptorParamValue, MinScore, NumMatches, ScoreType
* : HomMat2D, Score)
*描述:寻找描述模型,DetectorParamName和DescriptorParamName应该与创建模型时候相同。
*MinScore:当Score超过MinScore时候,这个匹配才被接受。
*对于每一个接受的匹配,都会产生一个3x3的矩阵HomMat2D用来描述转换。
*当一张图片中有多个匹配被接受的时候,单应性转换矩阵会串行的保存在tuple中。
* (应该是很多3x3矩阵串行保存)匹配的个数等于NumOfMatch =|HomMat2D|/9
*NumMatches:用来限制匹配的个数,最多有几个,选择最优的
*ScoreType: 可以选择匹配的点的个数或者是相关点的半径两种来表示匹配的优劣程度
*所以,这里的Score并不是一个0到1的值,而是一个可能比较大的整数
count_seconds (Seconds2)
*单个模型搜索花费时间
Time := Seconds2 - Seconds1
*所有模型搜索花费时间
TotalTime := TotalTime + Time
*
* Check if the found instance is to be considered as a possible right match
* depending on the number of points which were considered
if ((|HomMat2D| > 0) and (Score > NumPoints[Index2] / 4))
*5、获取描述点坐标
get_descriptor_model_points (ModelIDs[Index2], 'search', 0, Row, Col)
gen_cross_contour_xld (Cross, Row, Col, 6, 0.785398)
*
* Project the ROI rectangle and points
*6、投影变换ROI区域及边界点投影
projective_trans_region (Rectangle, TransRegion, HomMat2D, 'bilinear')
projective_trans_pixel (HomMat2D, RowRoi, ColRoi, RowTrans, ColTrans)
*7、根据边界点求夹角
angle_ll (RowTrans[2], ColTrans[2], RowTrans[1], ColTrans[1], RowTrans[1], ColTrans[1], RowTrans[0], ColTrans[0], Angle)
*8、夹角转换为角度值
Angle := deg(Angle)
*
* Check if the projected rectangle is to be considered as a right match
* depending on the angle in the right upper edge.
*9、根据夹角角度值范围筛选 并显示结果
if (Angle > 70 and Angle < 110)
area_center (TransRegion, Area, Row, Column)
ModelsFound := ModelsFound + 1
dev_set_color ('green')
dev_set_line_width (4)
dev_display (TransRegion)
dev_set_colored (12)
dev_set_line_width (1)
dev_display (Cross)
disp_message (WindowHandle, 'Page ' + (Index2 + 1), 'window', Row, Column, 'black', 'true')
OutputString := [OutputString,'Page ' + (Index2 + 1) + ' found in ' + (Time * 1000)$'.4' + ' ms\n']
endif
endif
endfor
if (ModelsFound == 0)
OutputString := 'No model found'
endif
NumMsgs := NumMsgs + 1
OutputString := ['Search time over all pages: ' + (TotalTime * 1000)$'.4' + ' ms',OutputString]
disp_message (WindowHandle, OutputString, 'window', 10, 10, 'black', 'true')
disp_continue_message (WindowHandle, 'black', 'true')
stop ()
endfor
dev_display (ImageGray)
disp_message (WindowHandle, 'Program finished.\nPress \'Run\' to clear all descriptor models.', 'window', 10, 10, 'black', 'true')
for i := 0 to |ModelIDs|-1 by 1
*10、清楚模型
clear_descriptor_model (ModelIDs[i])
endfor
结果
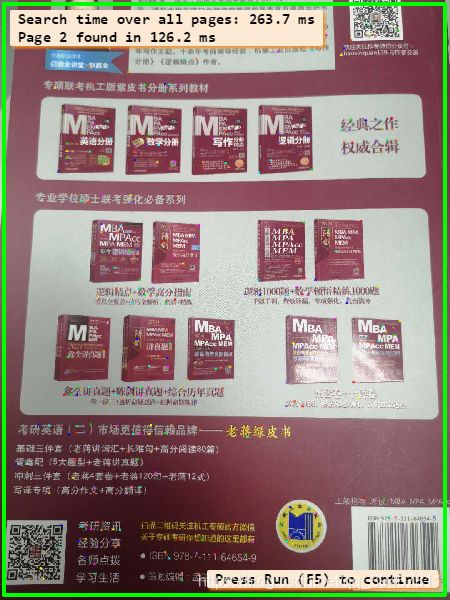
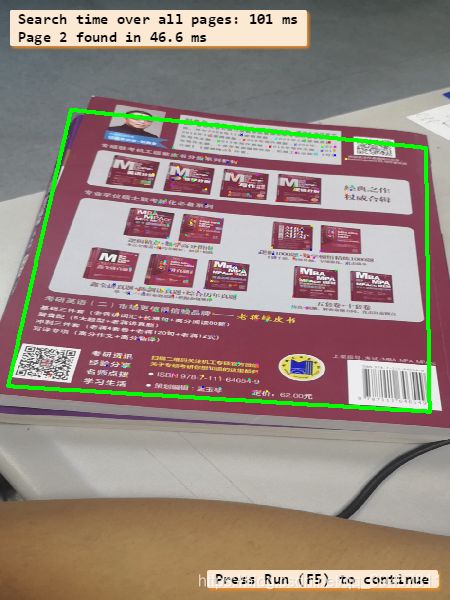
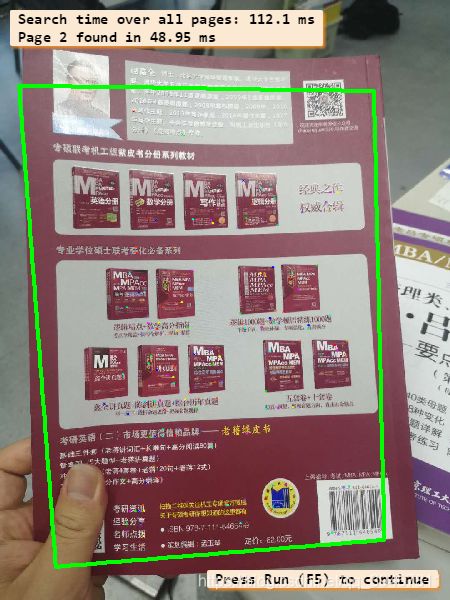
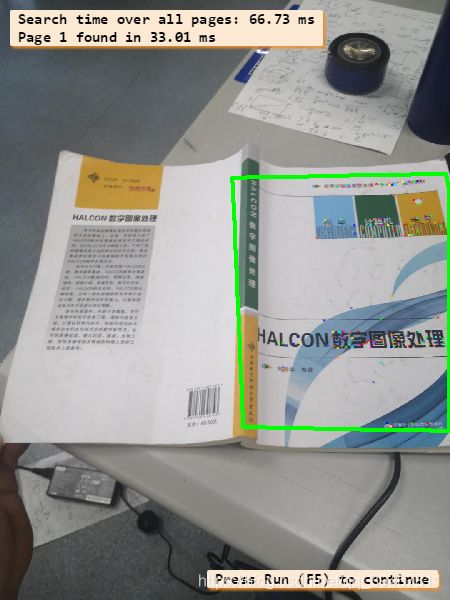
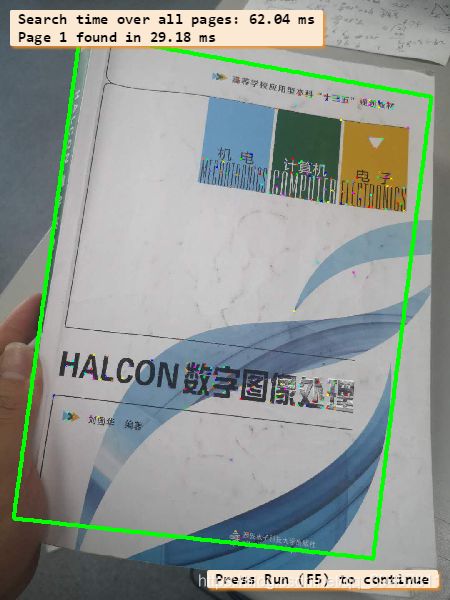
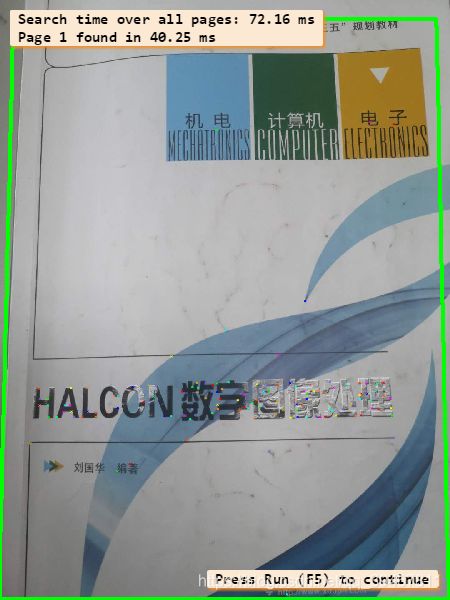