除了霍夫变换之外,还有别的方法来提取图像空间信息。最常用到的三种空间特征分别为HOG特征、LBP特征及Haar特征。
一、HOG特征
1、概念与思想
方向梯度直方图(Histogram of Oriented Gradient, HOG)特征是一种在计算机视觉和图像处理中用来描述图像局部特征的描述子。
在一幅图像中,局部目标的表象和形状(appearance and shape)能够被梯度或边缘的方向密度分布很好地描述。其本质是梯度的统计信息,而梯度主要存在于边缘所在的地方。
2、特点
与其他特征相比,HOG的优势在于能更好的描述形状,在行人识别方面有很好的效果。
由于HOG是在图像的局部方格单元上操作,所以它对图像几何的和光学的形变都能保持很好的不变性,这两种形变只会出现在更大的空间领域上。其次,在粗的空域抽样、精细的方向抽样以及较强的局部光学归一化等条件下,只要行人大体上能够保持直立的姿势,可以容许行人有一些细微的肢体动作,这些细微的动作可以被忽略而不影响检测效果。
3、流程
简单来说,首先需要将图像分成小的连通区域,称之为细胞单元。然后采集细胞单元中各像素点的梯度或边缘的方向直方图。最后把这些直方图组合起来就可以构成特征描述器。
STEP 1:读入所需要的检测目标即输入的image
STEP 2:将图像进行灰度化(将输入的彩色的图像的r,g,b值通过特定公式转换为灰度值)
STEP 3:采用Gamma校正法对输入图像进行颜色空间的标准化(归一化)
STEP 4:计算图像每个像素的梯度(包括大小和方向),捕获轮廓信息
统计每个cell的梯度直方图(不同梯度的个数),形成每个cell的descriptor
将每几个cell组成一个block(以3*3为例),一个block内所有cell的特征串联起来得到该block的HOG特征descriptor
STEP 5:将图像image内所有block的HOG特征descriptor串联起来得到该image(检测目标)的HOG特征descriptor,这就是最终分类的特征向量
4、源码实现
import cv2
import numpy as np
import math
import matplotlib.pyplot as plt
class Hog_descriptor():
def __init__(self, img, cell_size=16, bin_size=8):
self.img = img
self.img = np.sqrt(img / np.max(img))
self.img = img * 255
self.cell_size = cell_size
self.bin_size = bin_size
self.angle_unit = 360 / self.bin_size
assert type(self.bin_size) == int, "bin_size should be integer,"
assert type(self.cell_size) == int, "cell_size should be integer,"
assert type(self.angle_unit) == int, "bin_size should be divisible by 360"
def extract(self):
height, width = self.img.shape
gradient_magnitude, gradient_angle = self.global_gradient()
gradient_magnitude = abs(gradient_magnitude)
cell_gradient_vector = np.zeros((height / self.cell_size, width / self.cell_size, self.bin_size))
for i in range(cell_gradient_vector.shape[0]):
for j in range(cell_gradient_vector.shape[1]):
cell_magnitude = gradient_magnitude[i * self.cell_size:(i + 1) * self.cell_size,
j * self.cell_size:(j + 1) * self.cell_size]
cell_angle = gradient_angle[i * self.cell_size:(i + 1) * self.cell_size,
j * self.cell_size:(j + 1) * self.cell_size]
cell_gradient_vector[i][j] = self.cell_gradient(cell_magnitude, cell_angle)
hog_image = self.render_gradient(np.zeros([height, width]), cell_gradient_vector)
hog_vector = []
for i in range(cell_gradient_vector.shape[0] - 1):
for j in range(cell_gradient_vector.shape[1] - 1):
block_vector = []
block_vector.extend(cell_gradient_vector[i][j])
block_vector.extend(cell_gradient_vector[i][j + 1])
block_vector.extend(cell_gradient_vector[i + 1][j])
block_vector.extend(cell_gradient_vector[i + 1][j + 1])
mag = lambda vector: math.sqrt(sum(i ** 2 for i in vector))
magnitude = mag(block_vector)
if magnitude != 0:
normalize = lambda block_vector, magnitude: [element / magnitude for element in block_vector]
block_vector = normalize(block_vector, magnitude)
hog_vector.append(block_vector)
return hog_vector, hog_image
def global_gradient(self):
gradient_values_x = cv2.Sobel(self.img, cv2.CV_64F, 1, 0, ksize=5)
gradient_values_y = cv2.Sobel(self.img, cv2.CV_64F, 0, 1, ksize=5)
gradient_magnitude = cv2.addWeighted(gradient_values_x, 0.5, gradient_values_y, 0.5, 0)
gradient_angle = cv2.phase(gradient_values_x, gradient_values_y, angleInDegrees=True)
return gradient_magnitude, gradient_angle
def cell_gradient(self, cell_magnitude, cell_angle):
orientation_centers = [0] * self.bin_size
for i in range(cell_magnitude.shape[0]):
for j in range(cell_magnitude.shape[1]):
gradient_strength = cell_magnitude[i][j]
gradient_angle = cell_angle[i][j]
min_angle, max_angle, mod = self.get_closest_bins(gradient_angle)
orientation_centers[min_angle] += (gradient_strength * (1 - (mod / self.angle_unit)))
orientation_centers[max_angle] += (gradient_strength * (mod / self.angle_unit))
return orientation_centers
def get_closest_bins(self, gradient_angle):
idx = int(gradient_angle / self.angle_unit)
mod = gradient_angle % self.angle_unit
return idx, (idx + 1) % self.bin_size, mod
def render_gradient(self, image, cell_gradient):
cell_width = self.cell_size / 2
max_mag = np.array(cell_gradient).max()
for x in range(cell_gradient.shape[0]):
for y in range(cell_gradient.shape[1]):
cell_grad = cell_gradient[x][y]
cell_grad /= max_mag
angle = 0
angle_gap = self.angle_unit
for magnitude in cell_grad:
angle_radian = math.radians(angle)
x1 = int(x * self.cell_size + magnitude * cell_width * math.cos(angle_radian))
y1 = int(y * self.cell_size + magnitude * cell_width * math.sin(angle_radian))
x2 = int(x * self.cell_size - magnitude * cell_width * math.cos(angle_radian))
y2 = int(y * self.cell_size - magnitude * cell_width * math.sin(angle_radian))
cv2.line(image, (y1, x1), (y2, x2), int(255 * math.sqrt(magnitude)))
angle += angle_gap
return image
img = cv2.imread('person_037.png', cv2.IMREAD_GRAYSCALE)
hog = Hog_descriptor(img, cell_size=8, bin_size=8)
vector, image = hog.extract()
print np.array(vector).shape
plt.imshow(image, cmap=plt.cm.gray)
plt.show()
5、scikit-image库中的HOG
from skimage import feature as ft
features = ft.hog(image, # input image
orientations=ori, # number of bins
pixels_per_cell=ppc, # pixel per cell
cells_per_block=cpb, # cells per blcok
block_norm = 'L1', # block norm : str {‘L1’, ‘L1-sqrt’, ‘L2’, ‘L2-Hys’}
transform_sqrt = True, # power law compression (also known as gamma correction)
feature_vector=True, # flatten the final vectors
visualise=False) # return HOG map
二、LBP特征
1、概念与思想
局部二值模式(Local Binary Pattern,LBP)是一种计算机视觉和图像处理中用来描述图像局部特征的描述子。
其基本思想是用其中心像素的灰度值作为阈值,与它的邻域相比较得到的二进制码来表述局部纹理特征。
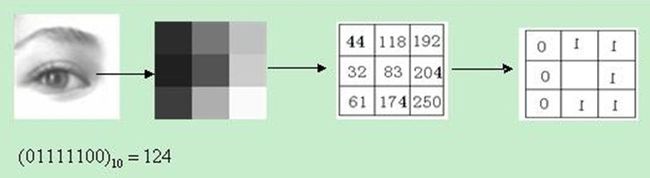
2、特点
与其他特征相比,LBP的优势在于能更好地描述纹理,在人脸识别方面有很好的效果,比Haar快很多倍(提取的准确率会低)因此适合用在移动设备上。
3、流程
STEP 1:首先将检测窗口划分为16X16的小区域(cell)
STEP 2:对于每个cell中的一个像素,将相邻的8个像素的灰度值与其进行比较,若周围像素值大于中心像素值,则该像素点的位置被标记为1,否则为0。这样,3X3领域内的8个点经过比较可产生8位二进制数,即得到该窗口中心像素点的LBP值
STEP 3:然后计算每个cell的直方图,即每个数字出现的频率,然后对该直方图进行归一化处理
STEP 4:最后将得到的每个cell的统计直方图进行连接成一个特征向量,也就是整幅图的LBP纹理特征向量
4、源码实现
# -*- coding: utf-8 -*-
import cv2
import numpy as np
import math
import os
import matplotlib.pyplot as plt
# 取得给定的LBP字符串的最小二进制值,实现旋转不变形
def Rotate_LBP_Min(str_lbp_input):
str_lbp_tmp = str_lbp_input
MinValue = int(str_lbp_tmp, 2) #转换二进制数
nLen = len(str_lbp_tmp)
for npos in range(nLen):
str_head = str_lbp_tmp[0]
str_tail = str_lbp_tmp[1:]
str_lbp_tmp = str_tail + str_head
CurrentValue = int(str_lbp_tmp, 2)
if CurrentValue image[row, col]: #像素比较
str_lbp_tmp = str_lbp_tmp + '1'
else:
str_lbp_tmp = str_lbp_tmp + '0'
res[row - 1][col - 1] =Rotate_LBP_Min(str_lbp_tmp) #写入结果中
return res
5、scikit-image库中的LBP
from skimage import feature as ft
features = ft.local_binary_pattern(image, # input image
P=n_points, # Number of circularly symmetric neighbour set points
R=radius, # Radius of circle
method='default') # {'default', 'ror', 'uniform', 'var'}
三、Haar特征
1、概念与思想
Haar特征分为三类:边缘特征、线性特征、中心特征和对角线特征,组合成特征模板。特征模板内有白色和黑色两种矩形,并定义该模板的特征值为白色矩形像素和减去黑色矩形像素和。
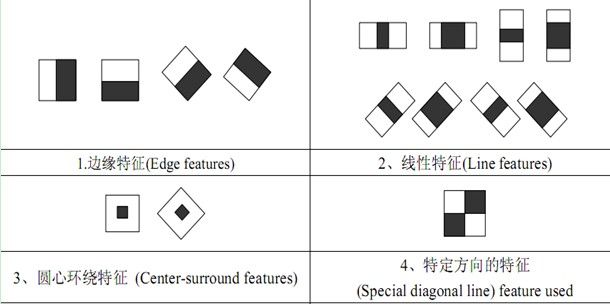
2、特点
与其他特征相比,Haar的优势在于能更好地描述灰度变化情况,用于检测正面的人脸(正脸由于鼻子等凸起的存在,使得脸上的光影变化十分明显)。
3、源码实现
# coding = utf-8
import cv2
import numpy as np
import matplotlib.pyplot as plt
# 计算积分图
def integral(img):
integ_graph = np.zeros((img.shape[0],img.shape[1]),dtype = np.int32)
for x in range(img.shape[0]):
sum_clo = 0
for y in range(img.shape[1]):
sum_clo = sum_clo + img[x][y]
integ_graph[x][y] = integ_graph[x-1][y] + sum_clo;
return integ_graph
#就算所有需要计算haar特征的区域
def getHaarFeaturesArea(width,height):
widthLimit = width-1
heightLimit = height/2-1
features = []
for w in range(1,int(widthLimit)):
for h in range(1,int(heightLimit)):
wMoveLimit = width - w
hMoveLimit = height - 2*h
for x in range(0, wMoveLimit):
for y in range(0, hMoveLimit):
features.append([x, y, w, h])
return features
#通过积分图特征区域计算haar特征
def calHaarFeatures(integral_graph,features_graph):
haarFeatures = []
for num in range(len(features_graph)):
#计算左面的矩形区局的像素和
haar1 = integral_graph[features_graph[num][0]][features_graph[num][1]]-\
integral_graph[features_graph[num][0]+features_graph[num][2]][features_graph[num][1]] -\
integral_graph[features_graph[num][0]][features_graph[num][1]+features_graph[num][3]] +\
integral_graph[features_graph[num][0]+features_graph[num][2]][features_graph[num][1]+features_graph[num][3]]
#计算右面的矩形区域的像素和
haar2 = integral_graph[features_graph[num][0]][features_graph[num][1]+features_graph[num][3]]-\
integral_graph[features_graph[num][0]+features_graph[num][2]][features_graph[num][1]+features_graph[num][3]] -\
integral_graph[features_graph[num][0]][features_graph[num][1]+2*features_graph[num][3]] +\
integral_graph[features_graph[num][0]+features_graph[num][2]][features_graph[num][1]+2*features_graph[num][3]]
#右面的像素和减去左面的像素和
haarFeatures.append(haar2-haar1)
return haarFeatures
img = cv2.imread("faces/face00001.bmp",0)
integeralGraph = integral(img)
featureAreas = getHaarFeaturesArea(img.shape[0],img.shape[1])
haarFeatures = calHaarFeatures(integeralGraph,featureAreas)
print(haarFeatures)
4、scikit-image库中的Haar
from skimage import feature as ft
features = ft.haar_like_feature(image, # input image
r, # Row-coordinate of top left corner of the detection window.
c, # Column-coordinate of top left corner of the detection window.
width, # Width of the detection window.
height, # Height of the detection window.
feature_type=None # The type of feature to consider:
)