Jupyter notebook阅读模式
离散化和面元划分
- 为了便于分析,连续数据常常被离散化或拆分为“面元”(bin)。假设有一组人员数据,而你希望将它们划分为不同的年龄组:
- 接下来将这些数据划分为“18到25”、“26到35”、“36到60”以及“60以上”几个面元。要实现该功能,你需要使用pandas的cut函数:
import pandas as pd
ages = [20,22,25,27,21,23,37,31,61,45,41,32]
bins = [18,25,35,60,100]
cats = pd.cut(ages,bins)
cats
[(18, 25], (18, 25], (18, 25], (25, 35], (18, 25], ..., (25, 35], (60, 100], (35, 60], (35, 60], (25, 35]]
Length: 12
Categories (4, interval[int64]): [(18, 25] < (25, 35] < (35, 60] < (60, 100]]
- pandas返回的是一个特殊的Categorical对象。结果展示了pandas.cut划分的面元。你可以将其看做一组表示面元名称的字符串。它的底层含有一个表示不同分类名称的类型数组,以及一个codes属性中的年龄数据的标签:
cats.codes
array([0, 0, 0, 1, 0, 0, 2, 1, 3, 2, 2, 1], dtype=int8)
cats.categories
IntervalIndex([(18, 25], (25, 35], (35, 60], (60, 100]]
closed='right',
dtype='interval[int64]')
pd.value_counts(cats)
(18, 25] 5
(35, 60] 3
(25, 35] 3
(60, 100] 1
dtype: int64
- 跟“区间”的数学符号一样,圆括号表示开端,而方括号则表示闭端(包括)。哪边是闭端可以通过right=False进行修改:
pd.cut(ages,bins,right=False)
[[18, 25), [18, 25), [25, 35), [25, 35), [18, 25), ..., [25, 35), [60, 100), [35, 60), [35, 60), [25, 35)]
Length: 12
Categories (4, interval[int64]): [[18, 25) < [25, 35) < [35, 60) < [60, 100)]
- 以通过传递一个列表或数组到labels,设置自己的面元名称:
group_names = ['youth','youongAdult','middleAged','Senior']
cuts = pd.cut(ages,bins,labels=group_names)
cuts
[youth, youth, youth, youongAdult, youth, ..., youongAdult, Senior, middleAged, middleAged, youongAdult]
Length: 12
Categories (4, object): [youth < youongAdult < middleAged < Senior]
pd.value_counts(cuts)
youth 5
middleAged 3
youongAdult 3
Senior 1
dtype: int64
- 如果向cut传入的是面元的数量而不是确切的面元边界,则它会根据数据的最小值和最大值计算等长面元。下面这个例子中,我们将一些均匀分布的数据分成四组:
import numpy as np
data = np.random.randn(20)
pd.cut(data,4,precision=2)
[(0.059, 0.7], (-1.23, -0.59], (-1.23, -0.59], (-0.59, 0.059], (-1.23, -0.59], ..., (-1.23, -0.59], (-0.59, 0.059], (-0.59, 0.059], (0.059, 0.7], (-1.23, -0.59]]
Length: 20
Categories (4, interval[float64]): [(-1.23, -0.59] < (-0.59, 0.059] < (0.059, 0.7] < (0.7, 1.35]]
- qcut是一个非常类似于cut的函数,它可以根据样本分位数对数据进行面元划分。根据数据的分布情况,cut可能无法使各个面元中含有相同数量的数据点。而qcut由于使用的是样本分位数,因此可以得到大小基本相等的面元:
data = np.random.randn(1000)
cats = pd.qcut(data,4)
cats
[(-3.113, -0.663], (0.0257, 0.636], (-0.663, 0.0257], (0.0257, 0.636], (-3.113, -0.663], ..., (0.636, 3.167], (0.0257, 0.636], (0.636, 3.167], (0.636, 3.167], (-0.663, 0.0257]]
Length: 1000
Categories (4, interval[float64]): [(-3.113, -0.663] < (-0.663, 0.0257] < (0.0257, 0.636] < (0.636, 3.167]]
pd.value_counts(cats)
(0.636, 3.167] 250
(0.0257, 0.636] 250
(-0.663, 0.0257] 250
(-3.113, -0.663] 250
dtype: int64
cats= pd.qcut(data,[0,0.1,0.5,0.9,1])
cats
[(-1.251, 0.0257], (0.0257, 1.186], (-1.251, 0.0257], (0.0257, 1.186], (-1.251, 0.0257], ..., (0.0257, 1.186], (0.0257, 1.186], (0.0257, 1.186], (0.0257, 1.186], (-1.251, 0.0257]]
Length: 1000
Categories (4, interval[float64]): [(-3.113, -1.251] < (-1.251, 0.0257] < (0.0257, 1.186] < (1.186, 3.167]]
pd.value_counts(cats)
(0.0257, 1.186] 400
(-1.251, 0.0257] 400
(1.186, 3.167] 100
(-3.113, -1.251] 100
dtype: int64
检测和过滤异常值
- 过滤或变换异常值(outlier)在很大程度上就是运用数组运算。
data = pd.DataFrame(np.random.randn(1000,4))
data.describe()
|
0 |
1 |
2 |
3 |
count |
1000.000000 |
1000.000000 |
1000.000000 |
1000.000000 |
mean |
0.003206 |
-0.055531 |
0.025491 |
0.006302 |
std |
0.982034 |
0.976494 |
1.014954 |
1.008318 |
min |
-3.104001 |
-2.996501 |
-3.017601 |
-3.412504 |
25% |
-0.656546 |
-0.710482 |
-0.671690 |
-0.670718 |
50% |
0.013119 |
-0.098854 |
0.062160 |
0.003732 |
75% |
0.662366 |
0.646807 |
0.684332 |
0.678343 |
max |
2.945120 |
2.863509 |
3.381626 |
2.977406 |
col = data[2]
col[np.abs(col)>3]
254 3.381626
384 3.123540
516 3.021305
699 -3.017601
Name: 2, dtype: float64
data[(np.abs(data)>3).any(1)]
|
0 |
1 |
2 |
3 |
25 |
-3.104001 |
-0.096051 |
-0.688123 |
2.458123 |
254 |
0.943157 |
0.446261 |
3.381626 |
-0.057649 |
384 |
-0.729623 |
-0.415029 |
3.123540 |
0.141025 |
516 |
0.316320 |
-0.269799 |
3.021305 |
-0.205189 |
699 |
-1.135125 |
0.200782 |
-3.017601 |
0.344913 |
914 |
0.791697 |
-1.385219 |
0.773297 |
-3.412504 |
data[np.abs(data)>3] = np.sign(data)*3
data.describe()
|
0 |
1 |
2 |
3 |
count |
1000.000000 |
1000.000000 |
1000.000000 |
1000.000000 |
mean |
0.003310 |
-0.055531 |
0.024982 |
0.006714 |
std |
0.981710 |
0.976494 |
1.013276 |
1.007002 |
min |
-3.000000 |
-2.996501 |
-3.000000 |
-3.000000 |
25% |
-0.656546 |
-0.710482 |
-0.671690 |
-0.670718 |
50% |
0.013119 |
-0.098854 |
0.062160 |
0.003732 |
75% |
0.662366 |
0.646807 |
0.684332 |
0.678343 |
max |
2.945120 |
2.863509 |
3.000000 |
2.977406 |
np.sign(data).head()
|
0 |
1 |
2 |
3 |
0 |
-1.0 |
-1.0 |
1.0 |
1.0 |
1 |
1.0 |
1.0 |
1.0 |
-1.0 |
2 |
1.0 |
-1.0 |
1.0 |
1.0 |
3 |
1.0 |
-1.0 |
1.0 |
-1.0 |
4 |
-1.0 |
1.0 |
1.0 |
-1.0 |
排列和随机采样
- 利用numpy.random.permutation函数可以轻松实现对Series或DataFrame的列的排列工作(permuting,随机重排序)。通过需要排列的轴的长度调用permutation,可产生一个表示新顺序的整数数组
df = pd.DataFrame(np.arange(5*4).reshape((5,4)))
sampler = np.random.permutation(5)
sampler
array([2, 4, 0, 1, 3])
df
|
0 |
1 |
2 |
3 |
0 |
0 |
1 |
2 |
3 |
1 |
4 |
5 |
6 |
7 |
2 |
8 |
9 |
10 |
11 |
3 |
12 |
13 |
14 |
15 |
4 |
16 |
17 |
18 |
19 |
df.take(sampler)
|
0 |
1 |
2 |
3 |
2 |
8 |
9 |
10 |
11 |
4 |
16 |
17 |
18 |
19 |
0 |
0 |
1 |
2 |
3 |
1 |
4 |
5 |
6 |
7 |
3 |
12 |
13 |
14 |
15 |
df.sample(n=3)
|
0 |
1 |
2 |
3 |
4 |
16 |
17 |
18 |
19 |
2 |
8 |
9 |
10 |
11 |
0 |
0 |
1 |
2 |
3 |
choices = pd.Series([5,7,-1,6,4])
draws = choices.sample(n=10,replace=True)
draws
2 -1
4 4
0 5
0 5
1 7
1 7
4 4
4 4
0 5
1 7
dtype: int64
计算指标和哑变量
- 另一种常用于统计建模或机器学习的转换方式是:将分类变量(categorical variable)转换为“哑变量”或“指标矩阵”。
df = pd.DataFrame({'key':['b', 'b', 'a', 'c', 'a', 'b'],
'data':range(6)})
df
|
key |
data |
0 |
b |
0 |
1 |
b |
1 |
2 |
a |
2 |
3 |
c |
3 |
4 |
a |
4 |
5 |
b |
5 |
pd.get_dummies(df['key'])
|
a |
b |
c |
0 |
0 |
1 |
0 |
1 |
0 |
1 |
0 |
2 |
1 |
0 |
0 |
3 |
0 |
0 |
1 |
4 |
1 |
0 |
0 |
5 |
0 |
1 |
0 |
dummies = pd.get_dummies(df['key'],prefix='key')
df_with_dummy = df[['data']].join(dummies)
df_with_dummy
|
data |
key_a |
key_b |
key_c |
0 |
0 |
0 |
1 |
0 |
1 |
1 |
0 |
1 |
0 |
2 |
2 |
1 |
0 |
0 |
3 |
3 |
0 |
0 |
1 |
4 |
4 |
1 |
0 |
0 |
5 |
5 |
0 |
1 |
0 |
mnames = ['movie_id','title','genres']
movies = pd.read_table('datasets/movielens/movies.dat',sep='::',header=None,names=mnames)
movies[:10]
d:\program filles\python\lib\site-packages\ipykernel_launcher.py:3: ParserWarning: Falling back to the 'python' engine because the 'c' engine does not support regex separators (separators > 1 char and different from '\s+' are interpreted as regex); you can avoid this warning by specifying engine='python'.
This is separate from the ipykernel package so we can avoid doing imports until
|
movie_id |
title |
genres |
0 |
1 |
Toy Story (1995) |
Animation|Children's|Comedy |
1 |
2 |
Jumanji (1995) |
Adventure|Children's|Fantasy |
2 |
3 |
Grumpier Old Men (1995) |
Comedy|Romance |
3 |
4 |
Waiting to Exhale (1995) |
Comedy|Drama |
4 |
5 |
Father of the Bride Part II (1995) |
Comedy |
5 |
6 |
Heat (1995) |
Action|Crime|Thriller |
6 |
7 |
Sabrina (1995) |
Comedy|Romance |
7 |
8 |
Tom and Huck (1995) |
Adventure|Children's |
8 |
9 |
Sudden Death (1995) |
Action |
9 |
10 |
GoldenEye (1995) |
Action|Adventure|Thriller |
all_genres = []
for x in movies.genres:
all_genres.extend(x.split('|'))
genres = pd.unique(all_genres)
genres
array(['Animation', "Children's", 'Comedy', 'Adventure', 'Fantasy',
'Romance', 'Drama', 'Action', 'Crime', 'Thriller', 'Horror',
'Sci-Fi', 'Documentary', 'War', 'Musical', 'Mystery', 'Film-Noir',
'Western'], dtype=object)
movies.genres[0].split('|')
['Animation', "Children's", 'Comedy']
zero_matrix = np.zeros((len(movies),len(genres)))
dummies = pd.DataFrame(zero_matrix,columns=genres)
dummies[:5]
|
Animation |
Children's |
Comedy |
Adventure |
Fantasy |
Romance |
Drama |
Action |
Crime |
Thriller |
Horror |
Sci-Fi |
Documentary |
War |
Musical |
Mystery |
Film-Noir |
Western |
0 |
0.0 |
0.0 |
0.0 |
0.0 |
0.0 |
0.0 |
0.0 |
0.0 |
0.0 |
0.0 |
0.0 |
0.0 |
0.0 |
0.0 |
0.0 |
0.0 |
0.0 |
0.0 |
1 |
0.0 |
0.0 |
0.0 |
0.0 |
0.0 |
0.0 |
0.0 |
0.0 |
0.0 |
0.0 |
0.0 |
0.0 |
0.0 |
0.0 |
0.0 |
0.0 |
0.0 |
0.0 |
2 |
0.0 |
0.0 |
0.0 |
0.0 |
0.0 |
0.0 |
0.0 |
0.0 |
0.0 |
0.0 |
0.0 |
0.0 |
0.0 |
0.0 |
0.0 |
0.0 |
0.0 |
0.0 |
3 |
0.0 |
0.0 |
0.0 |
0.0 |
0.0 |
0.0 |
0.0 |
0.0 |
0.0 |
0.0 |
0.0 |
0.0 |
0.0 |
0.0 |
0.0 |
0.0 |
0.0 |
0.0 |
4 |
0.0 |
0.0 |
0.0 |
0.0 |
0.0 |
0.0 |
0.0 |
0.0 |
0.0 |
0.0 |
0.0 |
0.0 |
0.0 |
0.0 |
0.0 |
0.0 |
0.0 |
0.0 |
gen = movies.genres[0]
gen.split('|')
['Animation', "Children's", 'Comedy']
dummies.columns.get_indexer(gen.split('|'))
array([0, 1, 2], dtype=int32)
for i, gen in enumerate(movies.genres):
indices = dummies.columns.get_indexer(gen.split('|'))
dummies.iloc[i,indices] = 1
movies_windic = movies.join(dummies.add_prefix('Genre'))
movies_windic.iloc[0]
movie_id 1
title Toy Story (1995)
genres Animation|Children's|Comedy
GenreAnimation 1
GenreChildren's 1
GenreComedy 1
GenreAdventure 0
GenreFantasy 0
GenreRomance 0
GenreDrama 0
GenreAction 0
GenreCrime 0
GenreThriller 0
GenreHorror 0
GenreSci-Fi 0
GenreDocumentary 0
GenreWar 0
GenreMusical 0
GenreMystery 0
GenreFilm-Noir 0
GenreWestern 0
Name: 0, dtype: object
- 一个对统计应用有用的秘诀是:结合get_dummies和诸如cut之类的离散化函数:
np.random.seed(12345)
values = np.random.rand(10)
values
array([0.92961609, 0.31637555, 0.18391881, 0.20456028, 0.56772503,
0.5955447 , 0.96451452, 0.6531771 , 0.74890664, 0.65356987])
bins = [0,0.2,0.4,0.6,0.8,1]
pd.get_dummies(pd.cut(values,bins))
|
(0.0, 0.2] |
(0.2, 0.4] |
(0.4, 0.6] |
(0.6, 0.8] |
(0.8, 1.0] |
0 |
0 |
0 |
0 |
0 |
1 |
1 |
0 |
1 |
0 |
0 |
0 |
2 |
1 |
0 |
0 |
0 |
0 |
3 |
0 |
1 |
0 |
0 |
0 |
4 |
0 |
0 |
1 |
0 |
0 |
5 |
0 |
0 |
1 |
0 |
0 |
6 |
0 |
0 |
0 |
0 |
1 |
7 |
0 |
0 |
0 |
1 |
0 |
8 |
0 |
0 |
0 |
1 |
0 |
9 |
0 |
0 |
0 |
1 |
0 |
7.3字符串操作
字符串对象方法
val = "a,b, guido"
val.split(',')
['a', 'b', ' guido']
pieces = [x.strip() for x in val.split(',')]
pieces
['a', 'b', 'guido']
first, second, third = pieces
first + "::" + second + "::" + third
'a::b::guido'
- 注意find和index的区别:如果找不到字符串,index将会引发一个异常(而不是返回-1):
"::".join(pieces)
'a::b::guido'
'guido' in val
True
val.index(',')
1
val.find(':')
-1
val.index(':')
---------------------------------------------------------------------------
ValueError Traceback (most recent call last)
in ()
----> 1 val.index(':')
ValueError: substring not found
val.count(',')
2
val.count('gui')
1
- replace()可以将一个模式替换为另一个模式,通过传入空字符串可以用于删除模式
val.replace(',', '::')
'a::b:: guido'
val.replace(',', '')
'ab guido'
- 以下列出了python内置字符串的方法
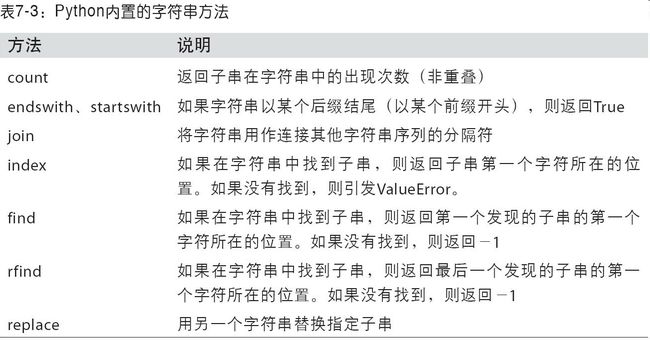
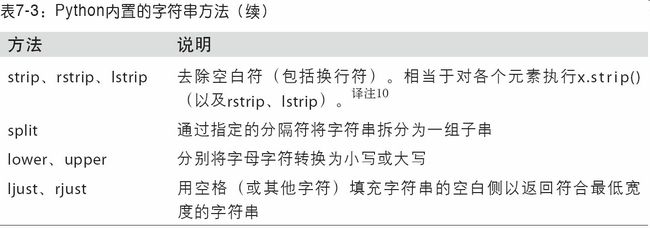
正则表达式
- 正则表达式,常称作regex,是根据正则表达式语言编写的字符串。re模块的函数可以分为三个大类:模式匹配、替换以及拆分。
import re
text = "foo bar\t baz \tqux"
re.split('\s+', text)
['foo', 'bar', 'baz', 'qux']
- 调用re.split(’\s+’,text)时,正则表达式会先被编译,然后再在text上调用其split方法。你可以用re.compile自己编译regex以得到一个可重用的regex对象:
regex = re.compile('\s+')
regex.split(text)
['foo', 'bar', 'baz', 'qux']
regex.findall(text)
[' ', '\t ', ' \t']
- 如果打算对许多字符串应用同一条正则表达式,强烈建议通过re.compile创建regex对象。这样将可以节省大量的CPU时间。
- indall返回的是字符串中所有的匹配项,而search则只返回第一个匹配项。match更加严格,它只匹配字符串的首部
import re
text = """Dave [email protected]
Steve [email protected]
Rob [email protected]
Ryan [email protected]
"""
pattern = r'[A-Z0-9._%+-]+@[A-Z0-9.-]+\.[A-Z]{2,4}'
regex = re.compile(pattern, flags=re.IGNORECASE)
regex.findall(text)
['[email protected]', '[email protected]', '[email protected]', '[email protected]']
- search返回的是文本中第一个电子邮件地址(以特殊的匹配项对象形式返回)。对于上面那个regex,匹配项对象只能告诉我们模式在原字符串中的起始和结束位置:
m = regex.search(text)
m
<_sre.SRE_Match object; span=(5, 20), match='[email protected]'>
text[m.start():m.end()]
'[email protected]'
- regex.match则将返回None,因为它只匹配出现在字符串开头的模式:
print(regex.match(text))
None
- sub方法可以将匹配到的模式替换为指定字符串,并返回所得到的新字符串:
print(regex.sub('REDACTED',text))
Dave REDACTED
Steve REDACTED
Rob REDACTED
Ryan REDACTED
- 假设你不仅想要找出电子邮件地址,还想将各个地址分成3个部分:用户名、域名以及域后缀。要实现此功能,只需将待分段的模式的各部分用圆括号包起来即可:
pattern = r'([A-Z0-9._%+-]+)@([A-Z0-9.-]+)\.([A-Z]{2,4})'
regex = re.compile(pattern,flags=re.IGNORECASE)
m = regex.match('[email protected]')
m.groups()
('wesm', 'bright', 'net')
regex.findall(text)
[('dave', 'google', 'com'),
('steve', 'gmail', 'com'),
('rob', 'gmail', 'com'),
('ryan', 'yahoo', 'com')]
- sub还能通过诸如\1、\2之类的特殊符号访问各匹配项中的分组。符号\1对应第一个匹配的组,\2对应第二个匹配的组,以此类推:
print(regex.sub(r'Username: \1, Domain: \2, Suffix: \3',text))
Dave Username: dave, Domain: google, Suffix: com
Steve Username: steve, Domain: gmail, Suffix: com
Rob Username: rob, Domain: gmail, Suffix: com
Ryan Username: ryan, Domain: yahoo, Suffix: com
pandas 的矢量化字符串函数
- 清理待分析的散乱数据时,常常需要做一些字符串规整化工作。更为复杂的情况是,含有字符串的列有时还含有缺失数据:
data = {'Dave': '[email protected]', 'Steve': '[email protected]',
'Rob': '[email protected]', 'Wes': np.nan}
data = pd.Series(data)
data
Dave [email protected]
Steve [email protected]
Rob [email protected]
Wes NaN
dtype: object
data.isnull()
Dave False
Steve False
Rob False
Wes True
dtype: bool
- 通过data.map,所有字符串和正则表达式方法都能被应用于(传入lambda表达式或其他函数)各个值,但是如果存在NA(null)就会报错。为了解决这个问题,Series有一些能够跳过NA值的面向数组方法,进行字符串操作。通过Series的str属性即可访问这些方法。例如,我们可以通过str.contains检查各个电子邮件地址是否含有"gmail":
data.str.contains('gmail')
Dave False
Steve True
Rob True
Wes NaN
dtype: object
pattern = '([A-Z0-9.%+-]+)@([A-Z0-9.-]+)\\.([A-Z]{2,4})'
data.str.findall(pattern, flags=re.IGNORECASE)
Dave [(dave, google, com)]
Steve [(steve, gmail, com)]
Rob [(rob, gmail, com)]
Wes NaN
dtype: object
- 有两个办法可以实现矢量化的元素获取操作:要么使用str.get,要么在str属性上使用索引:
matches = data.str.match(pattern, flags=re.IGNORECASE)
matches
Dave True
Steve True
Rob True
Wes NaN
dtype: object
matches.str.get(1)
Dave NaN
Steve NaN
Rob NaN
Wes NaN
dtype: float64
matches.str[0]
Dave NaN
Steve NaN
Rob NaN
Wes NaN
dtype: float64
data.str[:5]
Dave dave@
Steve steve
Rob rob@g
Wes NaN
dtype: object
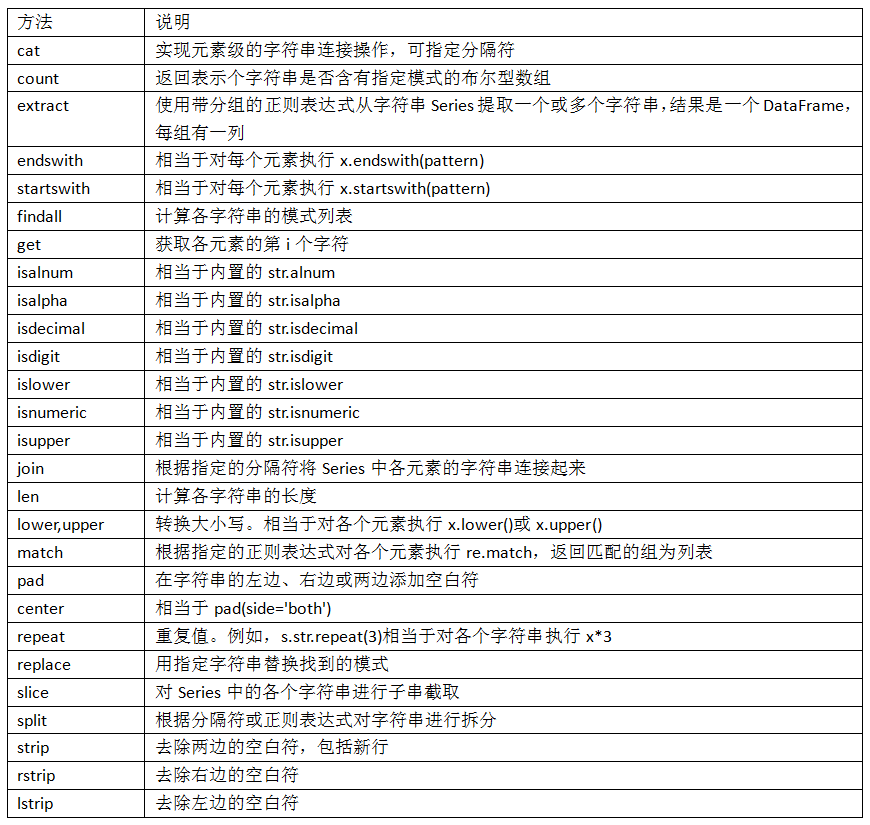