我们主要介绍基于TensorFlow的程序实现,为了实现以下程序,你需要安装 TensorFlow, Numpy, Scipy, 以及下载 VGG-19 model。
参考来源:
https://github.com/ckmarkoh/neuralart_tensorflow
https://github.com/log0/neural-style-painting/blob/master/TensorFlow%20Implementation%20of%20A%20Neural%20Algorithm%20of%20Artistic%20Style.ipynb
import os
import sys
import numpy as np
import scipy.io
import scipy.misc
import tensorflow as tf
OUTPUT_DIR = 'output/'
STYLE_IMAGE = '/images/ocean.jpg'
CONTENT_IMAGE = '/images/Taipei101.jpg'
IMAGE_WIDTH = 800
IMAGE_HEIGHT = 600
COLOR_CHANNELS = 3
NOISE_RATIO = 0.6
ITERATIONS = 1000
alpha = 1
beta = 500
VGG_Model = 'Downloads/imagenet-vgg-verydeep-19.mat'
MEAN_VALUES = np.array([123.68, 116.779, 103.939]).reshape((1, 1, 1, 3))
CONTENT_LAYERS = [('conv4_2', 1.)]
STYLE_LAYERS = [('conv1_1', 0.2), ('conv2_1', 0.2), ('conv3_1', 0.2), ('conv4_1', 0.2), ('conv5_1', 0.2)]
def generate_noise_image(content_image, noise_ratio = NOISE_RATIO):
"""
Returns a noise image intermixed with the content image at a certain ratio.
"""
noise_image = np.random.uniform(
-20, 20,
(1, IMAGE_HEIGHT, IMAGE_WIDTH, COLOR_CHANNELS)).astype('float32')
img = noise_image * noise_ratio + content_image * (1 - noise_ratio)
return img
def load_image(path):
image = scipy.misc.imread(path)
image = np.reshape(image, ((1,) + image.shape))
image = image - MEAN_VALUES
return image
def save_image(path, image):
image = image + MEAN_VALUES
image = image[0]
image = np.clip(image, 0, 255).astype('uint8')
scipy.misc.imsave(path, image)
def build_net(ntype, nin, nwb=None):
if ntype == 'conv':
return tf.nn.relu(tf.nn.conv2d(nin, nwb[0], strides=[1, 1, 1, 1], padding='SAME') + nwb[1])
elif ntype == 'pool':
return tf.nn.avg_pool(nin, ksize=[1, 2, 2, 1],
strides=[1, 2, 2, 1], padding='SAME')
def get_weight_bias(vgg_layers, i):
weights = vgg_layers[i][0][0][2][0][0]
weights = tf.constant(weights)
bias = vgg_layers[i][0][0][2][0][1]
bias = tf.constant(np.reshape(bias, (bias.size)))
return weights, bias
def build_vgg19(path):
net = {}
vgg_rawnet = scipy.io.loadmat(path)
vgg_layers = vgg_rawnet['layers'][0]
net['input'] = tf.Variable(np.zeros((1, IMAGE_HEIGHT, IMAGE_WIDTH, 3)).astype('float32'))
net['conv1_1'] = build_net('conv', net['input'], get_weight_bias(vgg_layers, 0))
net['conv1_2'] = build_net('conv', net['conv1_1'], get_weight_bias(vgg_layers, 2))
net['pool1'] = build_net('pool', net['conv1_2'])
net['conv2_1'] = build_net('conv', net['pool1'], get_weight_bias(vgg_layers, 5))
net['conv2_2'] = build_net('conv', net['conv2_1'], get_weight_bias(vgg_layers, 7))
net['pool2'] = build_net('pool', net['conv2_2'])
net['conv3_1'] = build_net('conv', net['pool2'], get_weight_bias(vgg_layers, 10))
net['conv3_2'] = build_net('conv', net['conv3_1'], get_weight_bias(vgg_layers, 12))
net['conv3_3'] = build_net('conv', net['conv3_2'], get_weight_bias(vgg_layers, 14))
net['conv3_4'] = build_net('conv', net['conv3_3'], get_weight_bias(vgg_layers, 16))
net['pool3'] = build_net('pool', net['conv3_4'])
net['conv4_1'] = build_net('conv', net['pool3'], get_weight_bias(vgg_layers, 19))
net['conv4_2'] = build_net('conv', net['conv4_1'], get_weight_bias(vgg_layers, 21))
net['conv4_3'] = build_net('conv', net['conv4_2'], get_weight_bias(vgg_layers, 23))
net['conv4_4'] = build_net('conv', net['conv4_3'], get_weight_bias(vgg_layers, 25))
net['pool4'] = build_net('pool', net['conv4_4'])
net['conv5_1'] = build_net('conv', net['pool4'], get_weight_bias(vgg_layers, 28))
net['conv5_2'] = build_net('conv', net['conv5_1'], get_weight_bias(vgg_layers, 30))
net['conv5_3'] = build_net('conv', net['conv5_2'], get_weight_bias(vgg_layers, 32))
net['conv5_4'] = build_net('conv', net['conv5_3'], get_weight_bias(vgg_layers, 34))
net['pool5'] = build_net('pool', net['conv5_4'])
return net
def content_layer_loss(p, x):
M = p.shape[1] * p.shape[2]
N = p.shape[3]
loss = (1. / (2 * N * M)) * tf.reduce_sum(tf.pow((x - p), 2))
return loss
def content_loss_func(sess, net):
layers = CONTENT_LAYERS
total_content_loss = 0.0
for layer_name, weight in layers:
p = sess.run(net[layer_name])
x = net[layer_name]
total_content_loss += content_layer_loss(p, x)*weight
total_content_loss /= float(len(layers))
return total_content_loss
def gram_matrix(x, area, depth):
x1 = tf.reshape(x, (area, depth))
g = tf.matmul(tf.transpose(x1), x1)
return g
def style_layer_loss(a, x):
M = a.shape[1] * a.shape[2]
N = a.shape[3]
A = gram_matrix(a, M, N)
G = gram_matrix(x, M, N)
loss = (1. / (4 * N ** 2 * M ** 2)) * tf.reduce_sum(tf.pow((G - A), 2))
return loss
def style_loss_func(sess, net):
layers = STYLE_LAYERS
total_style_loss = 0.0
for layer_name, weight in layers:
a = sess.run(net[layer_name])
x = net[layer_name]
total_style_loss += style_layer_loss(a, x) * weight
total_style_loss /= float(len(layers))
return total_style_loss
def main():
net = build_vgg19(VGG_Model)
sess = tf.Session()
sess.run(tf.initialize_all_variables())
content_img = load_image(CONTENT_IMAGE)
style_img = load_image(STYLE_IMAGE)
sess.run([net['input'].assign(content_img)])
cost_content = content_loss_func(sess, net)
sess.run([net['input'].assign(style_img)])
cost_style = style_loss_func(sess, net)
total_loss = alpha * cost_content + beta * cost_style
optimizer = tf.train.AdamOptimizer(2.0)
init_img = generate_noise_image(content_img)
train_op = optimizer.minimize(total_loss)
sess.run(tf.initialize_all_variables())
sess.run(net['input'].assign(init_img))
for it in range(ITERATIONS):
sess.run(train_op)
if it % 100 == 0:
mixed_image = sess.run(net['input'])
print('Iteration %d' % (it))
print('sum : ', sess.run(tf.reduce_sum(mixed_image)))
print('cost: ', sess.run(total_loss))
if not os.path.exists(OUTPUT_DIR):
os.mkdir(OUTPUT_DIR)
filename = 'output/%d.png' % (it)
save_image(filename, mixed_image)
if __name__ == '__main__':
main()
- 1
- 2
- 3
- 4
- 5
- 6
- 7
- 8
- 9
- 10
- 11
- 12
- 13
- 14
- 15
- 16
- 17
- 18
- 19
- 20
- 21
- 22
- 23
- 24
- 25
- 26
- 27
- 28
- 29
- 30
- 31
- 32
- 33
- 34
- 35
- 36
- 37
- 38
- 39
- 40
- 41
- 42
- 43
- 44
- 45
- 46
- 47
- 48
- 49
- 50
- 51
- 52
- 53
- 54
- 55
- 56
- 57
- 58
- 59
- 60
- 61
- 62
- 63
- 64
- 65
- 66
- 67
- 68
- 69
- 70
- 71
- 72
- 73
- 74
- 75
- 76
- 77
- 78
- 79
- 80
- 81
- 82
- 83
- 84
- 85
- 86
- 87
- 88
- 89
- 90
- 91
- 92
- 93
- 94
- 95
- 96
- 97
- 98
- 99
- 100
- 101
- 102
- 103
- 104
- 105
- 106
- 107
- 108
- 109
- 110
- 111
- 112
- 113
- 114
- 115
- 116
- 117
- 118
- 119
- 120
- 121
- 122
- 123
- 124
- 125
- 126
- 127
- 128
- 129
- 130
- 131
- 132
- 133
- 134
- 135
- 136
- 137
- 138
- 139
- 140
- 141
- 142
- 143
- 144
- 145
- 146
- 147
- 148
- 149
- 150
- 151
- 152
- 153
- 154
- 155
- 156
- 157
- 158
- 159
- 160
- 161
- 162
- 163
- 164
- 165
- 166
- 167
- 168
- 169
- 170
- 171
- 172
- 173
- 174
- 175
- 176
- 177
- 178
- 179
- 180
- 181
- 182
- 183
- 184
- 185
- 186
- 187
- 188
- 189
- 190
- 191
- 192
- 193
- 194
- 195
- 196
- 197
- 198
- 199
- 200
- 201
- 202
效果图
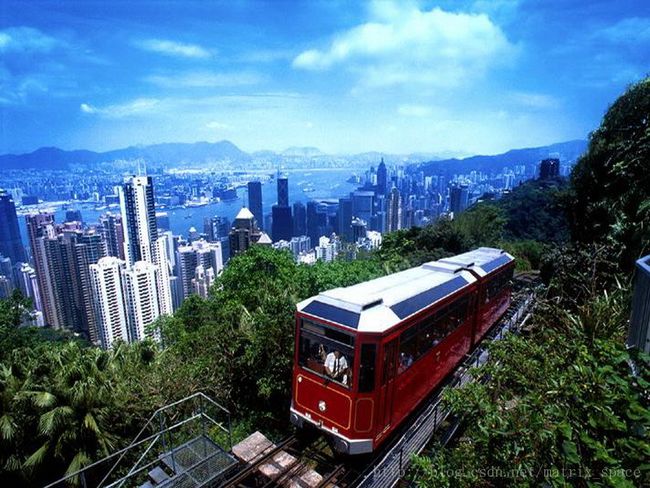
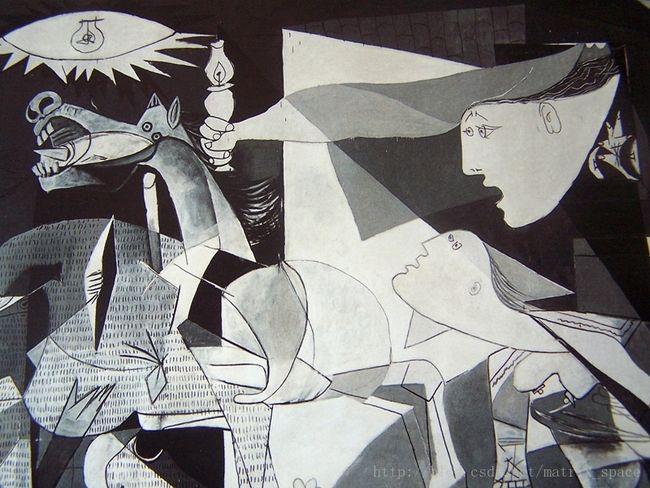
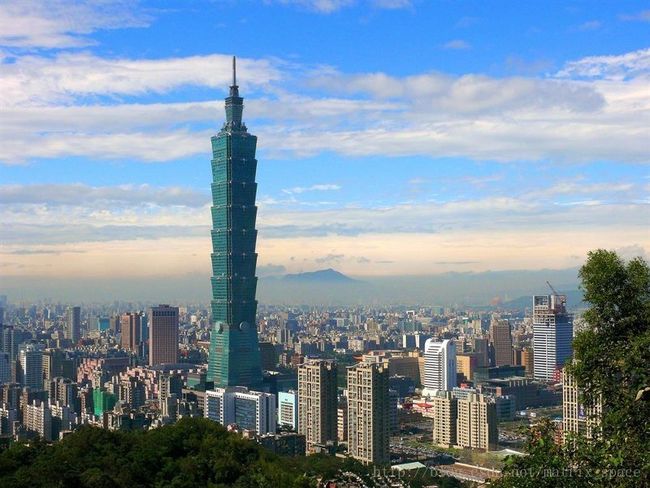
对前面的代码做了一些改变,设置了一个 image resize 函数,这样可以处理任意size的 input image,而且我们尝试利用 L-BFGS 优化算法替代之前的 Adam 优化算法,对卷积层以及pooling层函数做了修改。
import numpy as np
import scipy.io
import scipy.misc
from scipy.misc import imresize, imread
import tensorflow as tf
OUTPUT_DIR = 'output/'
STYLE_IMAGE = 'images/the_scream.jpg'
CONTENT_IMAGE = 'images/Taipei101.jpg'
IMAGE_WIDTH = 600
IMAGE_HEIGHT = 400
COLOR_CHANNELS = 3
NOISE_RATIO = 0.5
ITERATIONS = 500
alpha = 1
beta = 500
VGG_Model = 'imagenet-vgg-verydeep-19.mat'
MEAN_VALUES = np.array([123.68, 116.779, 103.939]).reshape((1, 1, 1, 3))
CONTENT_LAYERS = [('conv4_2', 1.)]
STYLE_LAYERS = [('conv1_1', 0.2), ('conv2_1', 0.2), ('conv3_1', 0.2), ('conv4_1', 0.2), ('conv5_1', 0.2)]
def generate_noise_image(content_image, noise_ratio = NOISE_RATIO):
"""
Returns a noise image intermixed with the content image at a certain ratio.
"""
noise_image = np.random.uniform(
-20, 20,
(1, IMAGE_HEIGHT, IMAGE_WIDTH, COLOR_CHANNELS)).astype('float32')
img = noise_image * noise_ratio + content_image * (1 - noise_ratio)
return img
def load_image(path):
image = imread(path)
image = imresize(image, (IMAGE_HEIGHT, IMAGE_WIDTH))
image = np.reshape(image, ((1,) + image.shape))
image = image - MEAN_VALUES
return image
def save_image(path, image):
image = image + MEAN_VALUES
image = image[0]
image = np.clip(image, 0, 255).astype('uint8')
scipy.misc.imsave(path, image)
def get_weight_bias(vgg_layers, layer_i):
weights = vgg_layers[layer_i][0][0][2][0][0]
w = tf.constant(weights)
bias = vgg_layers[layer_i][0][0][2][0][1]
b = tf.constant(np.reshape(bias, (bias.size)))
layer_name = vgg_layers[layer_i][0][0][0]
print layer_name
return w, b
def conv_relu_layer(layer_input, nwb):
conv_val = tf.nn.conv2d(layer_input, nwb[0], strides=[1, 1, 1, 1], padding='SAME')
relu_val = tf.nn.relu(conv_val + nwb[1])
return relu_val
def pool_layer(pool_style, layer_input):
if pool_style == 'avg':
return tf.nn.avg_pool(layer_input, ksize=[1, 2, 2, 1],
strides=[1, 2, 2, 1], padding='SAME')
elif pool_style == 'max':
return tf.nn.max_pool(layer_input, ksize=[1, 2, 2, 1],
strides=[1, 2, 2, 1], padding='SAME')
def build_vgg19(path):
net = {}
vgg_rawnet = scipy.io.loadmat(path)
vgg_layers = vgg_rawnet['layers'][0]
net['input'] = tf.Variable(np.zeros((1, IMAGE_HEIGHT, IMAGE_WIDTH, 3)).astype('float32'))
net['conv1_1'] = conv_relu_layer(net['input'], get_weight_bias(vgg_layers, 0))
net['conv1_2'] = conv_relu_layer(net['conv1_1'], get_weight_bias(vgg_layers, 2))
net['pool1'] = pool_layer('avg', net['conv1_2'])
net['conv2_1'] = conv_relu_layer(net['pool1'], get_weight_bias(vgg_layers, 5))
net['conv2_2'] = conv_relu_layer(net['conv2_1'], get_weight_bias(vgg_layers, 7))
net['pool2'] = pool_layer('max', net['conv2_2'])
net['conv3_1'] = conv_relu_layer(net['pool2'], get_weight_bias(vgg_layers, 10))
net['conv3_2'] = conv_relu_layer(net['conv3_1'], get_weight_bias(vgg_layers, 12))
net['conv3_3'] = conv_relu_layer(net['conv3_2'], get_weight_bias(vgg_layers, 14))
net['conv3_4'] = conv_relu_layer(net['conv3_3'], get_weight_bias(vgg_layers, 16))
net['pool3'] = pool_layer('avg', net['conv3_4'])
net['conv4_1'] = conv_relu_layer(net['pool3'], get_weight_bias(vgg_layers, 19))
net['conv4_2'] = conv_relu_layer(net['conv4_1'], get_weight_bias(vgg_layers, 21))
net['conv4_3'] = conv_relu_layer(net['conv4_2'], get_weight_bias(vgg_layers, 23))
net['conv4_4'] = conv_relu_layer(net['conv4_3'], get_weight_bias(vgg_layers, 25))
net['pool4'] = pool_layer('max', net['conv4_4'])
net['conv5_1'] = conv_relu_layer(net['pool4'], get_weight_bias(vgg_layers, 28))
net['conv5_2'] = conv_relu_layer(net['conv5_1'], get_weight_bias(vgg_layers, 30))
net['conv5_3'] = conv_relu_layer(net['conv5_2'], get_weight_bias(vgg_layers, 32))
net['conv5_4'] = conv_relu_layer(net['conv5_3'], get_weight_bias(vgg_layers, 34))
net['pool5'] = pool_layer('avg', net['conv5_4'])
return net
def content_layer_loss(p, x):
M = p.shape[1] * p.shape[2]
N = p.shape[3]
loss = (1. / (2 * N * M)) * tf.reduce_sum(tf.pow((x - p), 2))
return loss
def content_loss_func(sess, net):
layers = CONTENT_LAYERS
total_content_loss = 0.0
for layer_name, weight in layers:
p = sess.run(net[layer_name])
x = net[layer_name]
total_content_loss += content_layer_loss(p, x)*weight
total_content_loss /= float(len(layers))
return total_content_loss
def gram_matrix(x, area, depth):
x1 = tf.reshape(x, (area, depth))
g = tf.matmul(tf.transpose(x1), x1)
return g
def style_layer_loss(a, x):
M = a.shape[1] * a.shape[2]
N = a.shape[3]
A = gram_matrix(a, M, N)
G = gram_matrix(x, M, N)
loss = (1. / (4 * N ** 2 * M ** 2)) * tf.reduce_sum(tf.pow((G - A), 2))
return loss
def style_loss_func(sess, net):
layers = STYLE_LAYERS
total_style_loss = 0.0
for layer_name, weight in layers:
a = sess.run(net[layer_name])
x = net[layer_name]
total_style_loss += style_layer_loss(a, x) * weight
total_style_loss /= float(len(layers))
return total_style_loss
def main():
net = build_vgg19(VGG_Model)
sess = tf.Session()
sess.run(tf.initialize_all_variables())
content_img = load_image(CONTENT_IMAGE)
style_img = load_image(STYLE_IMAGE)
sess.run([net['input'].assign(content_img)])
cost_content = content_loss_func(sess, net)
sess.run([net['input'].assign(style_img)])
cost_style = style_loss_func(sess, net)
total_loss = alpha * cost_content + beta * cost_style
optimizer = tf.contrib.opt.ScipyOptimizerInterface(
total_loss, method='L-BFGS-B',
options={'maxiter': ITERATIONS,
'disp': 0})
init_img = generate_noise_image(content_img)
sess.run(tf.initialize_all_variables())
sess.run(net['input'].assign(init_img))
optimizer.minimize(sess)
mixed_img = sess.run(net['input'])
filename = 'output/out.png'
save_image(filename, mixed_img)
if __name__ == '__main__':
main()
- 1
- 2
- 3
- 4
- 5
- 6
- 7
- 8
- 9
- 10
- 11
- 12
- 13
- 14
- 15
- 16
- 17
- 18
- 19
- 20
- 21
- 22
- 23
- 24
- 25
- 26
- 27
- 28
- 29
- 30
- 31
- 32
- 33
- 34
- 35
- 36
- 37
- 38
- 39
- 40
- 41
- 42
- 43
- 44
- 45
- 46
- 47
- 48
- 49
- 50
- 51
- 52
- 53
- 54
- 55
- 56
- 57
- 58
- 59
- 60
- 61
- 62
- 63
- 64
- 65
- 66
- 67
- 68
- 69
- 70
- 71
- 72
- 73
- 74
- 75
- 76
- 77
- 78
- 79
- 80
- 81
- 82
- 83
- 84
- 85
- 86
- 87
- 88
- 89
- 90
- 91
- 92
- 93
- 94
- 95
- 96
- 97
- 98
- 99
- 100
- 101
- 102
- 103
- 104
- 105
- 106
- 107
- 108
- 109
- 110
- 111
- 112
- 113
- 114
- 115
- 116
- 117
- 118
- 119
- 120
- 121
- 122
- 123
- 124
- 125
- 126
- 127
- 128
- 129
- 130
- 131
- 132
- 133
- 134
- 135
- 136
- 137
- 138
- 139
- 140
- 141
- 142
- 143
- 144
- 145
- 146
- 147
- 148
- 149
- 150
- 151
- 152
- 153
- 154
- 155
- 156
- 157
- 158
- 159
- 160
- 161
- 162
- 163
- 164
- 165
- 166
- 167
- 168
- 169
- 170
- 171
- 172
- 173
- 174
- 175
- 176
- 177
- 178
- 179
- 180
- 181
- 182
- 183
- 184
- 185
- 186
- 187
- 188
- 189
- 190
- 191
- 192
- 193
- 194
- 195
- 196
- 197
- 198
- 199
- 200
- 201
- 202
- 203
- 204
- 205
- 206
- 207
- 208
- 209
- 210
- 211
- 212
- 213
- 214
- 215
- 216
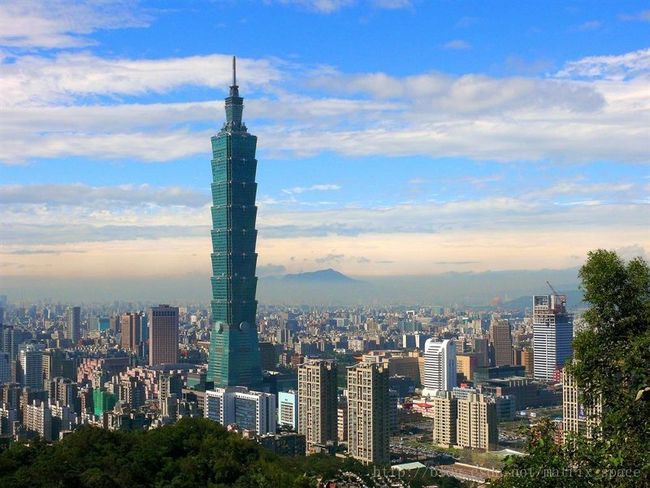
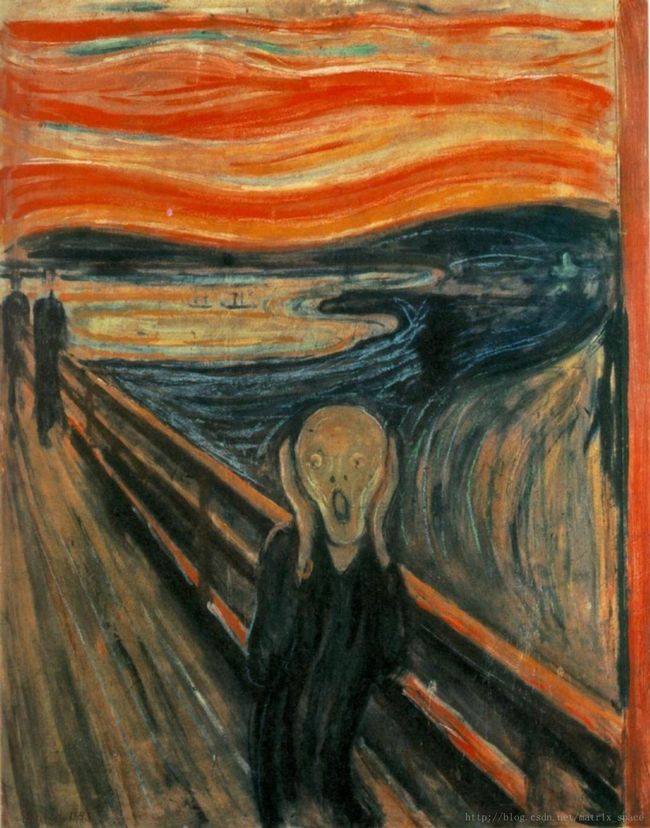
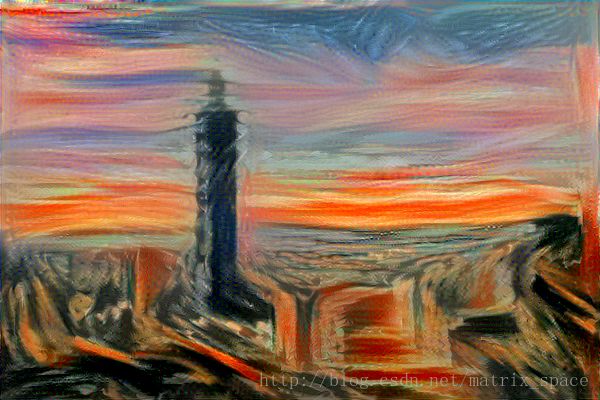
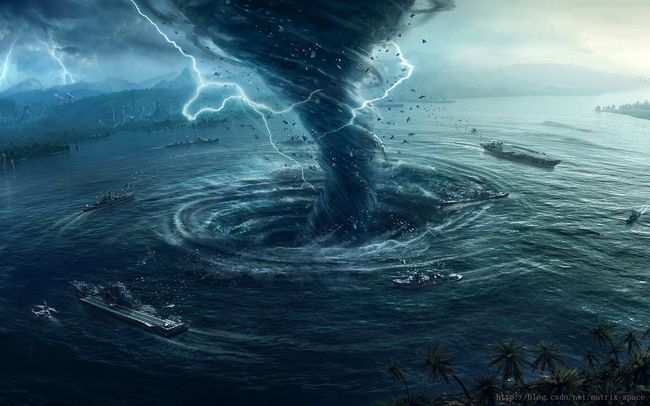
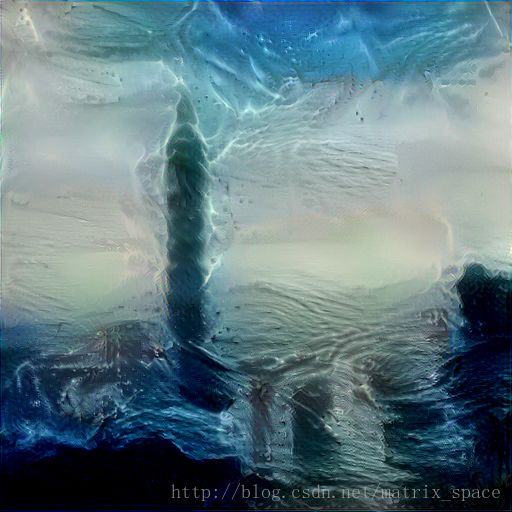