Transformer之时序预测(单特征or多特征)
(python3.8+pytorch)
股票的数据具有时序性,采用股票数据来进行预测
下载随机一只股票历史数据进行处理,此次选用600243的数据
数据处理思路如下
用前一段的x数据预测其后一段的y数据
每隔5取一组数据
import pandas as pd
import torch
import numpy as 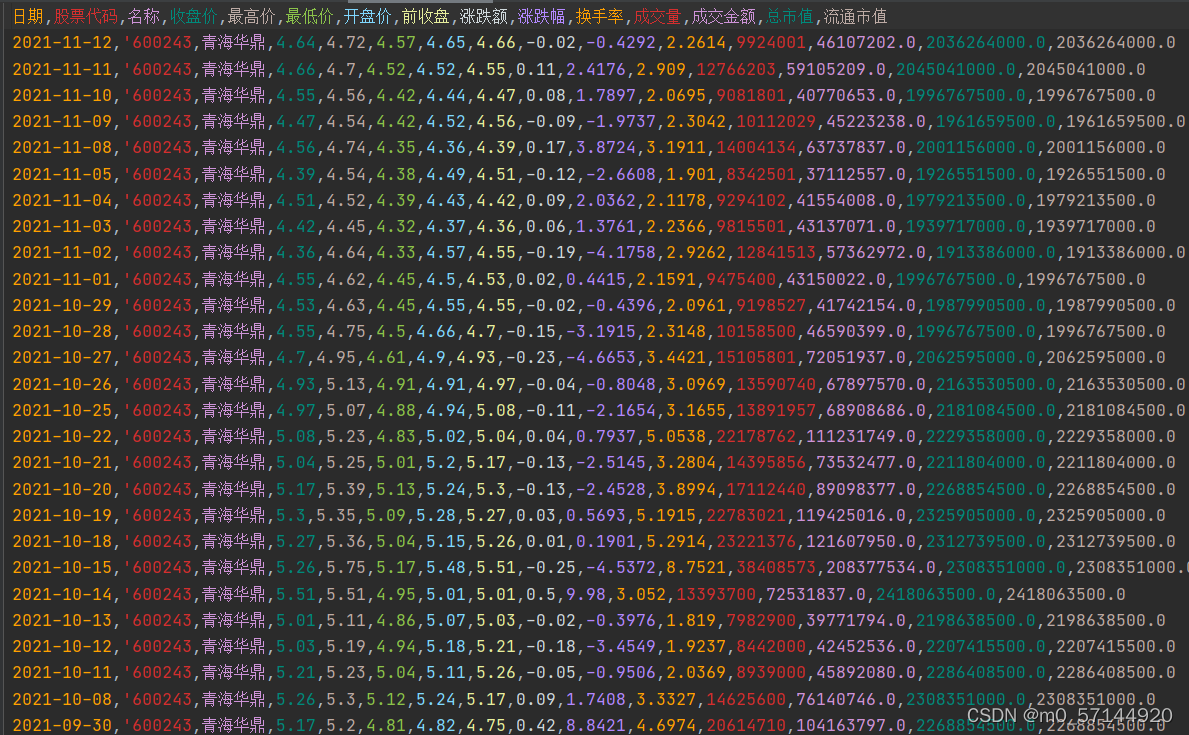
np
from torch.utils.data import TensorDataset
from torch.utils.data import DataLoader
#最后的效果 x---[batch,seq,feature_size] y---[batch,seq]
data_path=r'D:\pytorch_learn\second\600243pre\600243.csv'
elements=['收盘价','最高价','最低价','开盘价','前收盘']
#element='开盘价'
def single_data():#以收盘价为y,且x归一化
data_all = pd.read_csv(data_path, encoding='gbk')
data_ha = []
length = len(data_all)
for index, element in enumerate(elements):
data_element = data_all[element].values.astype(np.float64)
data_element = data_element.reshape(length, 1)
data_ha.append(data_element)
X_hat = np.concatenate(data_ha, axis=1) #[none,feture_size]
# X_hat=data_all[element].values.astype(np.float64)
max1 = np.max(X_hat,axis=0)
# X_hat=X_hat.reshape(-1,1)
temp = np.zeros(shape=(X_hat.shape[0],X_hat.shape[1]))
a = X_hat.shape[0]
for i in range(a):
temp[i, :] = X_hat[a - 1 - i, :]
y = temp[5:,3]
# y = temp[5:]
if len(y.shape)<2:
y = np.expand_dims(y,1)
X=temp/max1
X = X[0:-5, :]
return X,y #[none,feature_size] [none,feature_size]默认out_size为1
def data_load(seq_len):
x,y=single_data()
len=x.shape[0]
data_last_index=len-seq_len
X=[]
Y=[]
for i in range(0,data_last_index,5):
data_x=np.expand_dims(x[i:i+seq_len],0) #[1,seq,feature_size]
data_y=np.expand_dims(y[i:i+seq_len],0) #[1,seq,out_size]
# data_y=np.expand_dims(y[,0) #[1,seq,out_size]
X.append(data_x)
Y.append(data_y)
data_x= np.concatenate(X, axis=0)
data_y=np.concatenate(Y, axis=0)
data=torch.from_numpy(data_x).type(torch.float32)
label=torch.from_numpy(data_y).type(torch.float32)
return data,label #[num_data,seq,feature_size] [num_data,seq] 默认out_size为1
def dataset(seq_len,batch):
X,Y=data_load(seq_len)
feature_size=X.shape[-1]
out_size=Y.shape[-1]
dataset_train=TensorDataset(X,Y)
dataloader=DataLoader(dataset_train,batch_size=batch,shuffle=False)
return dataloader,feature_size,out_size
位置编码采用原transformer的位置编码,最大seq_len设置为64(可以随意)
通过传入【seq_len,batch,d_model】的输入,加上【seq_len,1,d_model】的位置编码,通过广播给每个batch加上位置编码
!!!!!!!!!!!!!!!!!
最重要的input_embedding部分
transformer想用于序列数据预测,需要将原模型的embedding部分改成线性层或其他结构,这里采用线性层
将【seq_len,batch,feature_size】转换为【seq_len,batch,d_model】
pytorch中transformer模型的输入shape如下
我们用于时序预测只采用encoding部分
nn.TransformerEncoder有两个参数需要传入
其中input_padding_mask也就是src_key_padding_mask这一步舍去了,直接采用全为一的mask矩阵
input也就是src的输入要求是【seq_len,batch,feature_size】,记得转换过来哦
decoding层采用线性层替换
框架如下
import torch
import torch.nn as nn
import math
class PositionalEncoding(nn.Module):
def __init__(self, d_model, max_len=64):
super(PositionalEncoding, self).__init__()
pe = torch.zeros(max_len, d_model) #64*512
position = torch.arange(0, max_len, dtype=torch.float).unsqueeze(1) #64*1
div_term = torch.exp(torch.arange(0, d_model, 2).float() * (-math.log(10000.0) / d_model)) #256 model/2
pe[:, 0::2] = torch.sin(position * div_term)
pe[:, 1::2] = torch.cos(position * div_term)
pe = pe.unsqueeze(0).transpose(0, 1)
# pe.requires_grad = False
self.register_buffer('pe', pe) #64*1*512
def forward(self, x): #[seq,batch,d_model]
return x + self.pe[:x.size(0), :] #64*64*512
class TransAm(nn.Module):
def __init__(self, feature_size,out_size,d_model=512, num_layers=1, dropout=0):
super(TransAm, self).__init__()
self.model_type = 'Transformer'
self.src_mask = None
self.embedding=nn.Linear(feature_size,512)
self.pos_encoder = PositionalEncoding(d_model) #50*512
self.encoder_layer = nn.TransformerEncoderLayer(d_model=d_model, nhead=8, dropout=dropout)
self.transformer_encoder = nn.TransformerEncoder(self.encoder_layer, num_layers=num_layers)
self.decoder = nn.Linear(d_model, out_size)
self.init_weights()
self.src_key_padding_mask = None
def init_weights(self):
initrange = 0.1
self.decoder.bias.data.zero_()
self.decoder.weight.data.uniform_(-initrange, initrange)
def forward(self, src, src_padding):
#shape of src [seq,batch,feature_size]
if self.src_key_padding_mask is None:
mask_key = src_padding #[batch,seq]
self.src_key_padding_mask = mask_key
src=self.embedding(src) #[seq,batch,d_model]
src = self.pos_encoder(src) #[seq,batch,d_model]
output = self.transformer_encoder(src, self.src_mask, self.src_key_padding_mask) # , self.src_mask)
output = self.decoder(output) #[seq,batch,output_size]
self.src_key_padding_mask = None
return output
此次是速成用法,主要是体验一下transformer用于时序预测的用法,所以没考虑到设置测试集,属于重大失误
根据数据集划分方式,用预测的最后五个参与loss计算
from model import TransAm
import torch.nn as nn
import torch
import torch.optim as optim
from matplotlib import pyplot
import numpy as np
import os
from data import dataset
png_save_path=r'D:\pytorch_learn\second\12.25transformer\png\4'
seq_len=64
batch=8
loader,feature_size,out_size=dataset(seq_len,batch)
model = TransAm(feature_size,out_size)
criterion = nn.MSELoss()
optimizer = optim.SGD(model.parameters(), lr=1e-3, momentum=0.99)
def train(epochs,isplot):
for epoch in range(epochs):
epoch_loss = 0
y_pre = []
y_true = []
for X, y in loader: #X--[batch,seq,feature_size] y--[batch,seq]
enc_inputs = X.permute([1,0,2]) #[seq,batch,feature_size]
y=y.permute([1,0,2])
key_padding_mask = torch.ones(enc_inputs.shape[1], enc_inputs.shape[0]) # [batch,seq]
optimizer.zero_grad()
output = model(enc_inputs, key_padding_mask)
#output--[seq,batch,out_size] enc_self_attns--[seq,seq]
output=output[-5:]
y=y[-5:]
loss=criterion(output,y)
loss.backward()
torch.nn.utils.clip_grad_norm_(model.parameters(), 0.5)
optimizer.step()
epoch_loss+=loss.item()
pres=output.detach().numpy() #[seq,batch,out_size]
pres=pres.transpose(0,1,2).reshape(-1,out_size) #[none,out_size]
tru=y.detach().numpy()
tru=tru.transpose(0,1,2).reshape(-1,out_size)
y_pre.append(pres)
y_true.append(tru)
pre=np.concatenate(y_pre,axis=0)
true=np.concatenate(y_true,axis=0)
if isplot:
pyplot.plot(true, color="blue", alpha=0.5)
pyplot.plot(pre, color="red", alpha=0.5)
pyplot.plot(pre - true, color="green", alpha=0.8)
pyplot.grid(True, which='both')
pyplot.axhline(y=0, color='k')
# pyplot.savefig(os.path.join(png_save_path, 'pre.png'))
pyplot.savefig(os.path.join(png_save_path, '%d.png' % epoch))
pyplot.close()
print('Epoch:', '%04d' % (epoch + 1), 'loss =', '{:.6f}'.format(epoch_loss))
train(30,True)
总体来说,能够逐步拟合
此次体验了pytorch中内置框架来预测时序数据,有以下几点需要注意的地方
1、input_embedding部分用Linear代替
2、decoding部分用Linear代替
3、训练中这一行代码特别重要,不然会梯度爆炸(消失)torch.nn.utils.clip_grad_norm_(model.parameters(), 0.5)
2022.4.24更新:
最近私信的小伙伴有点多(可能是大家都开始接手课题的原因吧哈哈),如想要代码的话可以在后台私信我留下邮箱,我看到了会发过去的。
接下来补充一下代码部分的缺点:
代码是去年写的,现在来看还有很多不足之处,有些地方写的也很稚嫩
1、没有配置gpu环境
2、batch_size在数据处理时设置为1了,导致loss看起来很大,而且训练效果不是很理想(我的理解是batch_size设置为1就相当于随机梯度下降了,收敛效果应该没有batch下降好),感兴趣的兄弟们可以在数据预处理代码部分改一下
3、超参数设置不是很方便,当时写代码的只想着快点跑起来,没考虑代码的可读性,现在写的话应该会用parser库把超参数都集中到一起
链接:https://pan.baidu.com/s/1EwWbaFwLDmCXeOUzv1UGBQ
提取码:7777
–来自百度网盘超级会员V4的分享
需要代码的小伙伴有点多。。。。。兄弟们代码自取吧,链接如上