环境搭建
- 本文初始环境为
PyTorch 2.0.0
、Python 3.8(ubuntu20.04)
、Cuda 11.8
OpenMMLab基础环境
- 首先安装
OpenMMLab
基础环境,以下代码均在命令窗口下输入
pip install openmim
mim install mmcv-full
mim install mmcv
mim install mmdeploy_runtime
git clone https://github.com/open-mmlab/mmdetection.git
cd mmdetection
pip install -e . >> /dev/null
mkdir checkpoint
mkdir output
cd ..
mim install mmcv-full
这一步需要大概15~30分钟,没报错就耐心等待。
MMDeploy环境
git clone https://github.com/open-mmlab/mmdeploy.git --recursive
cd mmdeploy
sudo cp /etc/apt/sources.list /etc/apt/sources.list.backup
sudo sed -i "s@http://.*archive.ubuntu.com@https://mirrors.tuna.tsinghua.edu.cn@g" /etc/apt/sources.list
sudo sed -i "s@http://.*security.ubuntu.com@https://mirrors.tuna.tsinghua.edu.cn@g" /etc/apt/sources.list
sudo apt-get update
python tools/scripts/build_ubuntu_x64_ort.py
export PYTHONPATH=$(pwd)/build/lib:$PYTHONPATH
export LD_LIBRARY_PATH=$(pwd)/build/lib:$(pwd)/../mmdeploy-dep/onnxruntime-linux-x64-1.8.1/lib:$LD_LIBRARY_PATH
- 可以使用脚本检查环境安装状态
python tools/check_env.py
07/14 19:14:16 - mmengine - INFO - **********Environmental information**********
07/14 19:14:17 - mmengine - INFO - sys.platform: linux
07/14 19:14:17 - mmengine - INFO - Python: 3.8.10 (default, Jun 4 2021, 15:09:15) [GCC 7.5.0]
07/14 19:14:17 - mmengine - INFO - CUDA available: True
07/14 19:14:17 - mmengine - INFO - numpy_random_seed: 2147483648
07/14 19:14:17 - mmengine - INFO - GPU 0: NVIDIA GeForce RTX 2080 Ti
07/14 19:14:17 - mmengine - INFO - CUDA_HOME: /usr/local/cuda
07/14 19:14:17 - mmengine - INFO - NVCC: Cuda compilation tools, release 11.8, V11.8.89
07/14 19:14:17 - mmengine - INFO - GCC: gcc (Ubuntu 9.4.0-1ubuntu1~20.04.1) 9.4.0
07/14 19:14:17 - mmengine - INFO - PyTorch: 2.0.0+cu118
07/14 19:14:17 - mmengine - INFO - PyTorch compiling details: PyTorch built with:
- GCC 9.3
- C++ Version: 201703
- Intel(R) oneAPI Math Kernel Library Version 2022.2-Product Build 20220804 for Intel(R) 64 architecture applications
- Intel(R) MKL-DNN v2.7.3 (Git Hash 6dbeffbae1f23cbbeae17adb7b5b13f1f37c080e)
- OpenMP 201511 (a.k.a. OpenMP 4.5)
- LAPACK is enabled (usually provided by MKL)
- NNPACK is enabled
- CPU capability usage: AVX2
- CUDA Runtime 11.8
- NVCC architecture flags: -gencode;arch=compute_37,code=sm_37;-gencode;arch=compute_50,code=sm_50;-gencode;arch=compute_60,code=sm_60;-gencode;arch=compute_70,code=sm_70;-gencode;arch=compute_75,code=sm_75;-gencode;arch=compute_80,code=sm_80;-gencode;arch=compute_86,code=sm_86;-gencode;arch=compute_90,code=sm_90
- CuDNN 8.7
- Magma 2.6.1
- Build settings: BLAS_INFO=mkl, BUILD_TYPE=Release, CUDA_VERSION=11.8, CUDNN_VERSION=8.7.0, CXX_COMPILER=/opt/rh/devtoolset-9/root/usr/bin/c++, CXX_FLAGS= -D_GLIBCXX_USE_CXX11_ABI=0 -fabi-version=11 -Wno-deprecated -fvisibility-inlines-hidden -DUSE_PTHREADPOOL -DNDEBUG -DUSE_KINETO -DLIBKINETO_NOROCTRACER -DUSE_FBGEMM -DUSE_QNNPACK -DUSE_PYTORCH_QNNPACK -DUSE_XNNPACK -DSYMBOLICATE_MOBILE_DEBUG_HANDLE -O2 -fPIC -Wall -Wextra -Werror=return-type -Werror=non-virtual-dtor -Werror=bool-operation -Wnarrowing -Wno-missing-field-initializers -Wno-type-limits -Wno-array-bounds -Wno-unknown-pragmas -Wunused-local-typedefs -Wno-unused-parameter -Wno-unused-function -Wno-unused-result -Wno-strict-overflow -Wno-strict-aliasing -Wno-error=deprecated-declarations -Wno-stringop-overflow -Wno-psabi -Wno-error=pedantic -Wno-error=redundant-decls -Wno-error=old-style-cast -fdiagnostics-color=always -faligned-new -Wno-unused-but-set-variable -Wno-maybe-uninitialized -fno-math-errno -fno-trapping-math -Werror=format -Werror=cast-function-type -Wno-stringop-overflow, LAPACK_INFO=mkl, PERF_WITH_AVX=1, PERF_WITH_AVX2=1, PERF_WITH_AVX512=1, TORCH_DISABLE_GPU_ASSERTS=ON, TORCH_VERSION=2.0.0, USE_CUDA=ON, USE_CUDNN=ON, USE_EXCEPTION_PTR=1, USE_GFLAGS=OFF, USE_GLOG=OFF, USE_MKL=ON, USE_MKLDNN=ON, USE_MPI=OFF, USE_NCCL=1, USE_NNPACK=ON, USE_OPENMP=ON, USE_ROCM=OFF,
07/14 19:14:17 - mmengine - INFO - TorchVision: 0.15.1+cu118
07/14 19:14:17 - mmengine - INFO - OpenCV: 4.8.0
07/14 19:14:17 - mmengine - INFO - MMEngine: 0.7.2
07/14 19:14:17 - mmengine - INFO - MMCV: 2.0.0
07/14 19:14:17 - mmengine - INFO - MMCV Compiler: GCC 9.3
07/14 19:14:17 - mmengine - INFO - MMCV CUDA Compiler: 11.8
07/14 19:14:17 - mmengine - INFO - MMDeploy: 1.2.0+0a8cbe2
07/14 19:14:17 - mmengine - INFO -
07/14 19:14:17 - mmengine - INFO - **********Backend information**********
07/14 19:14:17 - mmengine - INFO - tensorrt: None
07/14 19:14:17 - mmengine - INFO - ONNXRuntime: 1.8.1
07/14 19:14:17 - mmengine - INFO - ONNXRuntime-gpu: None
07/14 19:14:17 - mmengine - INFO - ONNXRuntime custom ops: Available
07/14 19:14:17 - mmengine - INFO - pplnn: None
07/14 19:14:17 - mmengine - INFO - ncnn: None
07/14 19:14:17 - mmengine - INFO - snpe: None
07/14 19:14:17 - mmengine - INFO - openvino: None
07/14 19:14:17 - mmengine - INFO - torchscript: 2.0.0+cu118
07/14 19:14:17 - mmengine - INFO - torchscript custom ops: NotAvailable
07/14 19:14:18 - mmengine - INFO - rknn-toolkit: None
07/14 19:14:18 - mmengine - INFO - rknn-toolkit2: None
07/14 19:14:18 - mmengine - INFO - ascend: None
07/14 19:14:18 - mmengine - INFO - coreml: None
07/14 19:14:18 - mmengine - INFO - tvm: None
07/14 19:14:18 - mmengine - INFO - vacc: None
07/14 19:14:18 - mmengine - INFO -
07/14 19:14:18 - mmengine - INFO - **********Codebase information**********
07/14 19:14:18 - mmengine - INFO - mmdet: 3.1.0
07/14 19:14:18 - mmengine - INFO - mmseg: None
07/14 19:14:18 - mmengine - INFO - mmpretrain: None
07/14 19:14:18 - mmengine - INFO - mmocr: None
07/14 19:14:18 - mmengine - INFO - mmagic: None
07/14 19:14:18 - mmengine - INFO - mmdet3d: None
07/14 19:14:18 - mmengine - INFO - mmpose: None
07/14 19:14:18 - mmengine - INFO - mmrotate: None
07/14 19:14:18 - mmengine - INFO - mmaction: None
07/14 19:14:18 - mmengine - INFO - mmrazor: None
07/14 19:14:18 - mmengine - INFO - mmyolo: None
YOLO-x转ONNX格式
cd ..
cd mmdetection
wget https://download.openmmlab.com/mmdetection/v2.0/yolox/yolox_x_8x8_300e_coco/yolox_x_8x8_300e_coco_20211126_140254-1ef88d67.pth -P checkpoint
cd ..
python mmdeploy/tools/deploy.py \
mmdeploy/configs/mmdet/detection/detection_onnxruntime_dynamic.py \
mmdetection/configs/yolox/yolox_x_8xb8-300e_coco.py \
mmdetection/checkpoint/yolox_x_8x8_300e_coco_20211126_140254-1ef88d67.pth \
mmdetection/demo/demo.jpg \
--work-dir mmdeploy_models/mmdet/yolox \
--device cpu \
--show \
--dump-info
- mmdeploy_models
- mmdet
- yolox
- deploy.json
- detail.json
- end2end.onnx
- pipeline.json
- 其中,
end2end.onnx
: 推理引擎文件。可用ONNX Runtime
推理。*.json
: mmdeploy SDK
推理所需的meta
信息。
模型推理
后端推理
from mmdeploy.apis.utils import build_task_processor
from mmdeploy.utils import get_input_shape, load_config
import torch
deploy_cfg = '/kaggle/working/mmdeploy/configs/mmdet/detection/detection_onnxruntime_dynamic.py'
model_cfg = '/kaggle/working/mmdetection/configs/yolox/yolox_x_8xb8-300e_coco.py'
device = 'cpu'
backend_model = ['/kaggle/input/yolo-x-onnx-model/end2end.onnx']
image = '/kaggle/working/mmdetection/demo/demo.jpg'
deploy_cfg, model_cfg = load_config(deploy_cfg, model_cfg)
task_processor = build_task_processor(model_cfg, deploy_cfg, device)
model = task_processor.build_backend_model(backend_model)
input_shape = get_input_shape(deploy_cfg)
model_inputs, _ = task_processor.create_input(image, input_shape)
with torch.no_grad():
result = model.test_step(model_inputs)
task_processor.visualize(
image=image,
model=model,
result=result[0],
window_name='visualize',
output_file='output_detection.png')
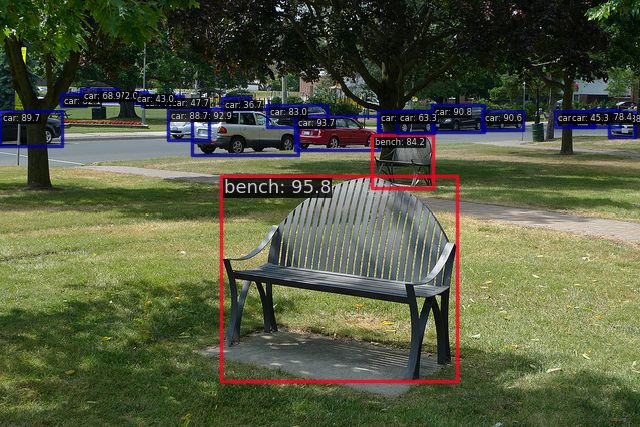
SDK推理
from mmdeploy_runtime import Detector
import cv2
import numpy as np
_COLORS = np.array([
0.000, 0.447, 0.741, 0.850, 0.325, 0.098, 0.929, 0.694, 0.125, 0.494,
0.184, 0.556, 0.466, 0.674, 0.188, 0.301, 0.745, 0.933, 0.635, 0.078,
0.184, 0.300, 0.300, 0.300, 0.600, 0.600, 0.600, 1.000, 0.000, 0.000,
1.000, 0.500, 0.000, 0.749, 0.749, 0.000, 0.000, 1.000, 0.000, 0.000,
0.000, 1.000, 0.667, 0.000, 1.000, 0.333, 0.333, 0.000, 0.333, 0.667,
0.000, 0.333, 1.000, 0.000, 0.667, 0.333, 0.000, 0.667, 0.667, 0.000,
0.667, 1.000, 0.000, 1.000, 0.333, 0.000, 1.000, 0.667, 0.000, 1.000,
1.000, 0.000, 0.000, 0.333, 0.500, 0.000, 0.667, 0.500, 0.000, 1.000,
0.500, 0.333, 0.000, 0.500, 0.333, 0.333, 0.500, 0.333, 0.667, 0.500,
0.333, 1.000, 0.500, 0.667, 0.000, 0.500, 0.667, 0.333, 0.500, 0.667,
0.667, 0.500, 0.667, 1.000, 0.500, 1.000, 0.000, 0.500, 1.000, 0.333,
0.500, 1.000, 0.667, 0.500, 1.000, 1.000, 0.500, 0.000, 0.333, 1.000,
0.000, 0.667, 1.000, 0.000, 1.000, 1.000, 0.333, 0.000, 1.000, 0.333,
0.333, 1.000, 0.333, 0.667, 1.000, 0.333, 1.000, 1.000, 0.667, 0.000,
1.000, 0.667, 0.333, 1.000, 0.667, 0.667, 1.000, 0.667, 1.000, 1.000,
1.000, 0.000, 1.000, 1.000, 0.333, 1.000, 1.000, 0.667, 1.000, 0.333,
0.000, 0.000, 0.500, 0.000, 0.000, 0.667, 0.000, 0.000, 0.833, 0.000,
0.000, 1.000, 0.000, 0.000, 0.000, 0.167, 0.000, 0.000, 0.333, 0.000,
0.000, 0.500, 0.000, 0.000, 0.667, 0.000, 0.000, 0.833, 0.000, 0.000,
1.000, 0.000, 0.000, 0.000, 0.167, 0.000, 0.000, 0.333, 0.000, 0.000,
0.500, 0.000, 0.000, 0.667, 0.000, 0.000, 0.833, 0.000, 0.000, 1.000,
0.000, 0.000, 0.000, 0.143, 0.143, 0.143, 0.286, 0.286, 0.286, 0.429,
0.429, 0.429, 0.571, 0.571, 0.571, 0.714, 0.714, 0.714, 0.857, 0.857,
0.857, 0.000, 0.447, 0.741, 0.314, 0.717, 0.741, 0.50, 0.5, 0
]).astype(np.float32).reshape(-1, 3)
coco_labels = [
'person', 'bicycle', 'car', 'motorcycle', 'airplane', 'bus',
'train', 'truck', 'boat', 'traffic light', 'fire hydrant', 'stop sign',
'parking meter', 'bench', 'bird', 'cat', 'dog', 'horse', 'sheep',
'cow', 'elephant', 'bear', 'zebra', 'giraffe', 'backpack', 'umbrella',
'handbag', 'tie', 'suitcase', 'frisbee', 'skis', 'snowboard',
'sports ball', 'kite', 'baseball bat', 'baseball glove', 'skateboard', 'surfboard',
'tennis racket', 'bottle', 'wine glass', 'cup', 'fork', 'knife', 'spoon',
'bowl', 'banana', 'apple', 'sandwich', 'orange', 'broccoli', 'carrot',
'hot dog', 'pizza', 'donut', 'cake', 'chair', 'couch', 'potted plant',
'bed', 'dining table', 'toilet', 'tv', 'laptop', 'mouse', 'remote',
'keyboard', 'cell phone', 'microwave', 'oven', 'toaster', 'sink', 'refrigerator',
'book', 'clock', 'vase', 'scissors', 'teddy bear', 'hair drier', 'toothbrush'
]
img = cv2.imread('demo/resources/lqq.jpg')
detector = Detector(model_path='/root/mmdeploy_models/mmdet/yolox', device_name='cpu', device_id=0)
bboxes, labels, masks = detector(img)
indices = [i for i in range(len(bboxes))]
for index, bbox, label_id in zip(indices, bboxes, labels):
[left, top, right, bottom], score = bbox[0:4].astype(int), bbox[4]
if score < 0.5:
continue
color = (_COLORS[label_id] * 255).astype(np.uint8).tolist()
text = '{}:{:.1f}%'.format(coco_labels[label_id], score * 100)
txt_color = (0, 0, 0) if np.mean(_COLORS[label_id]) > 0.5 else (255, 255,255)
font = cv2.FONT_HERSHEY_SIMPLEX
txt_size = cv2.getTextSize(text, font, 0.4, 1)[0]
cv2.rectangle(img, (left, top), (right, bottom), color,thickness=2)
txt_bk_color = (_COLORS[label_id] * 255 * 0.7).astype(np.uint8).tolist()
cv2.rectangle(img, (left, top + 1),
(left + txt_size[0] + 1, top + int(1.5 * txt_size[1])),
txt_bk_color, -1)
cv2.putText(img,
text, (left, top + txt_size[1]),
font,
0.4,
txt_color,
thickness=1)
cv2.imwrite('output_detection.png', img)