- 【论文研读】Better Together:Unifying Datalog and Equality Saturation
被制作时长两年半的个人练习生
Datalog编程语言Datalog程序分析
最近研究ReassociatePass整的头大,翻两篇Datalog的论文看看。今天看的一篇是比较新的文章,23年4月贴到arxiv上的。本文的主要贡献是提出了egglog,将Datalog和Eqsat结合起来,继承了Datalog的efficientincrementalexecution,cooperatinganalysisandlattice目录Introduction部分BackGrou
- 经典论文研读:《Bigtable: A Distributed Storage System for Structured Data》
WanderingScorpion
论文研读检索技术论文研读数据存储原力计划
一概述BigTable是以大神JeffreyDean为首的Google团队在2006年公开的分布式存储系统,是Google“三驾马车”论文中(GFS、MapReduce、BigTable)中最后公开的。在BigTable论文中,Google构思、设计并实现了一套支持结构化数据存储的超大容量分布式存储系统。BigTable中关于数据模型、底层存储技术和架构模型的设计思路直到今日仍被奉为经典,下面我们
- 深度学习学习笔记-论文研读4-基于深度强化学习的多用户边缘计算任务卸载调度与资源分配算法
丰。。
神经网络论文研读学报论文研读学习边缘计算算法人工智能深度学习
本人学识浅薄,如有理解不到位的地方还请大佬们指出,相互学习,共同进步概念引入强化学习DQN算法边缘计算边缘计算,是指在靠近物或数据源头的一侧,采用网络、计算、存储、应用核心能力为一体的开放平台,就近提供最近端服务。其应用程序在边缘侧发起,产生更快的网络服务响应,满足行业在实时业务、应用智能、安全与隐私保护等方面的基本需求。边缘计算处于物理实体和工业连接之间,或处于物理实体的顶端。而云端计算,仍然可
- 通俗科普文:贝叶斯优化与SMBO、高斯过程回归、TPE(附新书)
科技州与数据州
以下文章来源于SimpleAI,作者郭必扬贝叶斯优化是AutoML中的重要概念,近年来变得很火热。作为一种重要的基于先验的调参/策略选择技术,贝叶斯的应用范围也很广。但这个概念对于初次接触的同学可能较难理解,经过数天的论文研读、博客/教程/代码查阅,我总结了这篇科普文,也手绘了一些示意图,希望尽量在一篇文章内、通俗易懂地讲清楚什么是贝叶斯优化。本文目录:理清基本概念的关系各种超参数调节方法的对比G
- 【论文研读】基于卷积神经网络的图像局部风格迁移
lexonT
自2015年Gatys首次提出神经艺术风格迁移框架以来,图像风格迁移逐渐成为计算机图形学和计算机视觉领域的一个研究热点,但是当前针对图像风格迁移的研究大多难以提取图像中的局部进行风格迁移,而将重心放在图像全局风格迁移上,针对局部风格迁移这一研究领域上的空白,浙江工业大学缪永伟与浙江理工大学、中科院自动化研究所合作发表了《基于卷积神经网络的图像局部风格迁移》一文。文中提出了一种基于卷积神经网络的图像
- 2024 1.6~1.12 周报
shengMio
周报深度学习机器学习
一、上周工作论文研读二、本周计划思考毕业论文要用到的方法或者思想,多查多看积累可取之处。学习ppt和上周组会内容、卷积神经网络。三、完成情况1.数据训练的方式1.1迁移学习迁移学习是一种机器学习方法,把任务A训练出的模型作为初始模型,并使用它来改进新目标任务B的学习。即通过从已学习的相关任务中转移知识来改进学习的新任务。这可以包括使用模型作为特征提取器,微调模型,或使用模型的部分作为初始化。找到目
- OpenFWI 论文研读
shengMio
论文深度学习
论文title:OPENFWI:Large-scaleMulti-structuralBenchmarkDatasetsforFullWaveformInversion——OPENFWI:基于全波形反演的大规模多结构基准数据集摘要Abstract:全波形反演(FWI)在地球物理中被广泛用于从地震数据中重建高分辨率速度图。OPENFWI由12个数据集(共2.1TB)组成,这些数据集是从多个来源合成的
- 【论文研读】Detection of redundant expressions: A precise, efficient, and pragmatic algorithm in SSA.
被制作时长两年半的个人练习生
编程语言c++编译器值编号程序优化LLVM
继续研读GVN领域的文章,又是一篇重要的文章,此文提出的算法已经在LLVM中实现为NewGVN。能够找到所有Herbrand等值关系且时间复杂度为polynomial。目录IntroductionTheProblemTerminologyBasicConceptAlgorithmCorrectnessproofandcomplexityanalysisExperimentalresultsRela
- 【论文研读】Furthering Datalog in the pursuit of program analysis
被制作时长两年半的个人练习生
编程语言linux运维服务器
最近准备开一个新坑,记录一下读过的一些论文,主要聚焦笔者在阅读过程中的感悟,一些重点算法的理解,以及笔者觉得可以改进的地方。本文为系列的第一篇,试试水先。本文选择的论文是FurtheringDataloginthepursuitofprogramanalysis。是一篇剑桥大学的博士论文,发现此文的契机是在对valuenumbering技术进行跟踪时发现了一篇2004年的APolynomial-T
- 论文研读:基于统计重加权的方法减少通用回复
飞剑客阿飞
论文研读:基于统计重加权的方法减少通用回复会议名称:EMNLP2018文章题目:TowardsLessGenericResponsesinNeuralConversationModels:AStatisticalRe-weightingMethod原文链接:https://link.zhihu.com/?target=https%3A//www.paperweekly.site/papers/24
- 【论文研读】Minimax and Biobjective Portfolio Selection Based on Collaborative Neurodynamic Optimization
如果皮卡会coding
论文研读投资组合论文阅读minimax
MinimaxandBiobjectivePortfolioSelectionBasedonCollaborativeNeurodynamicOptimization基于协同神经动力学优化的极大极小双目标投资组合选择文章目录MinimaxandBiobjectivePortfolioSelectionBasedonCollaborativeNeurodynamicOptimization一.基本信
- 【10大专题,2.8w字详解】:从张量开始到GPT的《动手学深度学习》要点笔记
hadiii
gpt深度学习笔记人工智能transformer
《动手学深度学习PyTorch版》复习要点全记录专注于查漏补缺、巩固基础,这份笔记将带你深入理解深度学习的核心概念。通过一系列精心整理的小专题,逐步构建起你的AI知识框架。从最基础的张量操作,到最新的GPT模型,每个专题都配备了直观的图示和详细的公式解析。初版笔记以《动手学深度学习PyTorch版》书籍为基准,随后将根据视频讲解和最新论文研读内容进行实时更新。所有专题都配备了精美的图表和公式推导。
- 论文研读:基于统计重加权的方法减少通用回复
飞剑客阿飞
论文研读:基于统计重加权的方法减少通用回复会议名称:EMNLP2018文章题目:TowardsLessGenericResponsesinNeuralConversationModels:AStatisticalRe-weightingMethod原文链接:https://link.zhihu.com/?target=https%3A//www.paperweekly.site/papers/24
- 基于边缘计算的电力智慧物联系统设计与实现(论文研读)
椒椒。
边缘计算人工智能大数据
基于边缘计算的电力智慧物联系统设计与实现摘要:0引言1电力智慧物联系统架构设计1.1总体框架设计1.2物模型设计1.3边缘计算1.4交互协议1.5面向云边协同的智能生态1.5.1应用开发1.5.2智能生态1.5.3云边协同2实验验证及试点建设2.1实验测试2.1.1功能型测试2.1.2非功能性测试2.2现场试点建设3结语参考文献:基于边缘计算的电力智慧物联系统设计与实现.-论文研读崔恒志1,蒋承伶
- FreeMatch: Self-adaptive Thresholding for Semi-supervised Learning[论文研读笔记2023的ICLR]
白兔1205
汇报论文人工智能
原文链接:https://arxiv.org/abs/2205.07246代码链接:https://github.com/microsoft/Semi-supervised-learning作者视频讲解链接:https://www.bilibili.com/video/BV14L411k7De/?spm_id_from=333.999.0.0&vd_source=90e27a3caa4ef021d
- 论文研读|An Embarrassingly Simple Approach for Intellectual Property Rights Protection on RNNs
_Meilinger_
神经网络水印论文研读AI安全神经网络水印语言模型白盒水印版权保护模型水印RNN
目录论文信息文章简介研究动机研究方法白盒水印水印信号构造黑盒水印触发集构造水印嵌入实验结果保真度&有效性鲁棒性抗移除攻击(RemovalAttack)抗模型剪枝(ModelPruning)抗微调攻击(Fine-Tuning)抗水印覆写攻击(Overwriting)抗伪造攻击(Anti-AmbiguityAttacl)隐蔽性迁移性CaseStudy方法评估相关文献论文信息论文名称:AnEmbarra
- pointNet复现、论文和代码研读
苏钟白
python
文章目录论文复现论文研读1.动机2.模型结构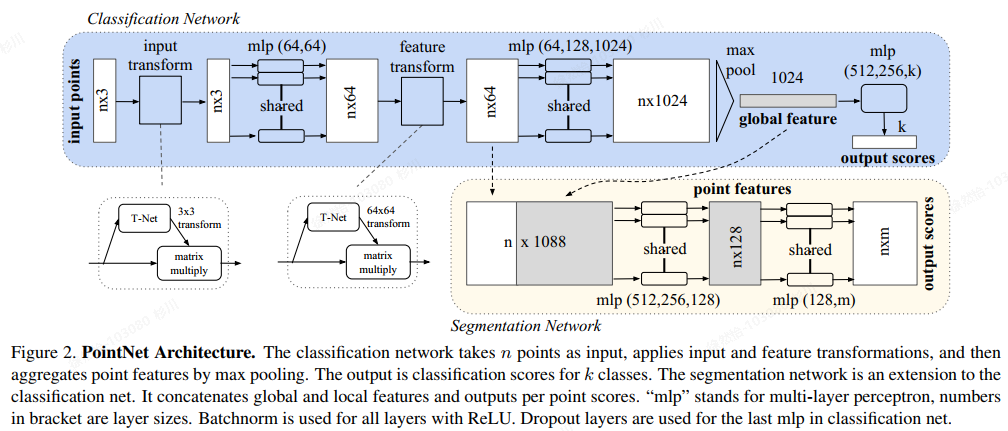3.实验效果4.总结代码研读模型什么时候保存,保存到哪里?模型训练的数据集?为什么是在CPU上运行的?运行的时候有输入gpu号如何测试模型的语义分割的效果?如何测试模型的分类效果?论文复现https:
- RFNet模型论文和代码研读
苏钟白
python
论文研读论文的代码:https://github.com/AHupuJR/RFNet论文动机截止到2020年,很少有实时的基于RGBD的分割模型。本文提出一种基于RGB-D的实时分割模型,可用于自动驾驶场景。模型的结构在编码器部分,两个独立的分支分别提取RGB的特征和深度的特征,RGB分支为主分支,深度分支为下级分支。每个分支都采用的ResNet18为骨干网络。深度分支输出的特征会通过AFC模块融
- 论文研读|Turning Your Weakness Into a Strength: Watermarking Deep Neural Networks by Backdooring
_Meilinger_
论文研读神经网络水印AI安全神经网络水印模型水印黑盒水印后门攻击深度学习
目录论文信息文章简介研究动机研究方法水印生成水印嵌入版权验证实验结果保真度(Functionality-Preserving)&有效性(Effectiveness)鲁棒性(Unremovability)抗微调攻击抗伪造攻击(OwnershipPiracy)抗迁移学习ImageNet方法评估相关文献论文信息论文名称:TurningYourWeaknessIntoaStrength:Watermark
- 论文研读|Watermarking Deep Neural Networks for Embedded Systems
_Meilinger_
神经网络水印论文研读人工智能AI安全网络空间安全神经网络水印模型水印黑盒水印后门攻击
目录论文信息文章简介研究动机研究方法水印生成水印嵌入版权验证实验结果方法评估有效性(Effectiveness)保真度(Fidelity)嵌入容量(Payload)假阳性(FalsePositiveRate)安全性(Security)篡改攻击(TamperingAttack)伪造攻击(GhostSignatureAttack)相关文献论文信息论文名称:WatermarkingDeepNeuralN
- 论文研读|Protecting Intellectual Property of Deep Neural Networks with Watermarking
_Meilinger_
神经网络水印论文研读人工智能深度学习机器学习AI安全神经网络水印黑盒水印后门攻击
目录论文信息文章简介研究动机研究方法水印生成水印嵌入版权验证实验结果有效性(Effectiveness)高效性(ConvergeSpeed)保真度(Functionality)鲁棒性(Robustness)Anti-剪枝攻击(Pruning)Anti-微调攻击(Fine-tuning)安全性(Security)Anti-模型逆向攻击(ModelInversion)方法评估相关文献论文信息论文名称:
- 论文研读 - share work - QPipe:一种并行流水线的查询执行引擎
yzs87
java开发语言
QPipe:一种并行流水线的查询执行引擎QPipe:ASimultaneouslyPipelinedRelationalQueryEngine关系型数据库通常独立执行并发的查询,每个查询都需执行一系列相关算子。为了充分利用并发查询中的数据扫描与计算,现有研究提出了丰富的技术:从缓存磁盘页以构建物化视图到优化多查询。然而,现有研究所提出的思想本质上受现代以查询为中心的引擎设计哲学所限制。理想状态下,
- MV-Map论文研读
高的好想出去玩啊
论文研读深度学习人工智能
MV-MapMV-Map:OffboardHD-MapGenerationwithMulti-viewConsistency论文:https://arxiv.org/pdf/2305.08851.pdfcode:https://github.com/ZiYang-xie/MV-Map代码未开源总体网络结构简述论文首次提出以非车载的方式产生高精度地图。可以视为在HDMapNet的优化版本,多三阶段网
- BEVFromer论文研读
高的好想出去玩啊
论文研读深度学习
1.总体结构上图为BEVFormer在t时刻的网络结构。图(a)表示的是BEVFormer的encoder层。BEVFormer有6个encoder层,每一个encoder除了本文自定义的三个组件外都和传统的transformers结果一致。自定义的三个组件分别是网格状的BEVqueries,TSA和SCA。其中BEVqueries的参数是可学习的,它通过注意力机制查询多相机视角下的BEV空间特征
- 论文研读|生成式跨模态隐写发展综述
_Meilinger_
文本隐写论文研读生成式隐写跨模态隐写SteganographyImageTextSpeech
前言:本文介绍近5年来生成式跨模态隐写领域的相关工作。相关阅读:生成式文本隐写发展综述不同于文本隐写,跨模态隐写需要考虑不同模态间的相关性,常见的跨模态场景有:Image-to-Text(如图像描述),Text-to-Speech(如语音助手),Text-to-Image(如按文作画)等。下面对基于深度学习的生成式跨模态隐写相关工作进行介绍。[1]-基于图像描述的文本信息隐藏(北京邮电大学学报,2
- 【连载】深度学习笔记14:CNN经典论文研读之Le-Net5及其Tensorflow实现
linux那些事
在前几次笔记中,笔者基本上将卷积神经网络的基本原理给讲完了。从本次笔记开始,笔者在深度学习笔记中会不定期的对CNN发展过程中的经典论文进行研读并推送研读笔记。今天笔者就和大家一起学习卷积神经网络和深度学习发展历史上具有奠基性的经典论文之一的关于LeNet-5网络一文。LeNet-5是由具有卷积神经网络之父之美誉的YannLeCun在1998年发表在IEEE上面的一篇Gradient-basedle
- TimeGAN学习记录
河马小白
GAN学习
一、学习TimeGAN主要参考的链接如下:(1)知乎上的TimeGAN论文研读(2)csdn上的一篇博客,论文阅读:《TimeSeriesGenerativeAdversrialNetworks》(TimeGAN,时间序列GAN)(3)时间序列丨基于TimeGAN模型生成时间序列数据及其Python实践二、我的理解TimeGAN无预测功能,只是对数据进行了分段处理并可以捕捉时序特征,但效果并不好?
- 第三周
YYYlan
论文研读研究方向:插画与动画叠加效果的运用与表现1.付博宇.动画前期设计中插画艺术的应用[J].明日风尚,2020(06):33-34.https://kns.cnki.net/KXReader/Detail?autoLogin=1&TIMESTAMP=637381423548572500&DBCODE=CJFD&TABLEName=CJFDLASN2020&FileName=MRFS202006
- 神经网络论文研读-多模态方向-综述研读(上)
丰。。
学报论文研读神经网络论文研读机器学习笔记神经网络人工智能深度学习
翻译以机翻为主原文目录前言图1:LMU印章(左)风格转移到梵高的向日葵绘画(中)并与提示混合-梵高,向日葵-通过CLIP+VGAN(右)。在过去的几年中,自然语言处理(NLP)和计算机视觉中使用的方法取得了一些突破。除了对单模态模型的这些改进之外,大规模多模态方法已成为一个非常活跃的研究领域。在本次研讨会中,我们回顾了这些方法,并试图创建一个坚实的该领域的概述,从当前最先进的方法分别是深度学习的两
- 深度学习神经网络学习笔记-自然语言处理方向-论文研读-情感分析/文本分类-textcnn
丰。。
深度学习神经网络-NLP方向神经网络论文研读神经网络自然语言处理深度学习人工智能神经网络语言模型
本文目录概念引入摘要大意TextCNN模型的结构正则化手段该模型的超参数研究成果概念引入逻辑回归线性回归时间序列分析神经网络self-attention与softmax的推导word2evcglove摘要大意在使用简单的CNN模型在预训练词向量的基础上进行微调就可以在文本分类任务上就能得到很好的结果。通过对词向量进行微调而获得的任务指向的词向量就能得到更好的结果。同时也提出了一种即使用静态预训练词
- html页面js获取参数值
0624chenhong
html
1.js获取参数值js
function GetQueryString(name)
{
var reg = new RegExp("(^|&)"+ name +"=([^&]*)(&|$)");
var r = windo
- MongoDB 在多线程高并发下的问题
BigCat2013
mongodbDB高并发重复数据
最近项目用到 MongoDB , 主要是一些读取数据及改状态位的操作. 因为是结合了最近流行的 Storm进行大数据的分析处理,并将分析结果插入Vertica数据库,所以在多线程高并发的情境下, 会发现 Vertica 数据库中有部分重复的数据. 这到底是什么原因导致的呢?笔者开始也是一筹莫 展,重复去看 MongoDB 的 API , 终于有了新发现 :
com.mongodb.DB 这个类有
- c++ 用类模版实现链表(c++语言程序设计第四版示例代码)
CrazyMizzz
数据结构C++
#include<iostream>
#include<cassert>
using namespace std;
template<class T>
class Node
{
private:
Node<T> * next;
public:
T data;
- 最近情况
麦田的设计者
感慨考试生活
在五月黄梅天的岁月里,一年两次的软考又要开始了。到目前为止,我已经考了多达三次的软考,最后的结果就是通过了初级考试(程序员)。人啊,就是不满足,考了初级就希望考中级,于是,这学期我就报考了中级,明天就要考试。感觉机会不大,期待奇迹发生吧。这个学期忙于练车,写项目,反正最后是一团糟。后天还要考试科目二。这个星期真的是很艰难的一周,希望能快点度过。
- linux系统中用pkill踢出在线登录用户
被触发
linux
由于linux服务器允许多用户登录,公司很多人知道密码,工作造成一定的障碍所以需要有时踢出指定的用户
1/#who 查出当前有那些终端登录(用 w 命令更详细)
# who
root pts/0 2010-10-28 09:36 (192
- 仿QQ聊天第二版
肆无忌惮_
qq
在第一版之上的改进内容:
第一版链接:
http://479001499.iteye.com/admin/blogs/2100893
用map存起来号码对应的聊天窗口对象,解决私聊的时候所有消息发到一个窗口的问题.
增加ViewInfo类,这个是信息预览的窗口,如果是自己的信息,则可以进行编辑.
信息修改后上传至服务器再告诉所有用户,自己的窗口
- java读取配置文件
知了ing
1,java读取.properties配置文件
InputStream in;
try {
in = test.class.getClassLoader().getResourceAsStream("config/ipnetOracle.properties");//配置文件的路径
Properties p = new Properties()
- __attribute__ 你知多少?
矮蛋蛋
C++gcc
原文地址:
http://www.cnblogs.com/astwish/p/3460618.html
GNU C 的一大特色就是__attribute__ 机制。__attribute__ 可以设置函数属性(Function Attribute )、变量属性(Variable Attribute )和类型属性(Type Attribute )。
__attribute__ 书写特征是:
- jsoup使用笔记
alleni123
java爬虫JSoup
<dependency>
<groupId>org.jsoup</groupId>
<artifactId>jsoup</artifactId>
<version>1.7.3</version>
</dependency>
2014/08/28
今天遇到这种形式,
- JAVA中的集合 Collectio 和Map的简单使用及方法
百合不是茶
listmapset
List ,set ,map的使用方法和区别
java容器类类库的用途是保存对象,并将其分为两个概念:
Collection集合:一个独立的序列,这些序列都服从一条或多条规则;List必须按顺序保存元素 ,set不能重复元素;Queue按照排队规则来确定对象产生的顺序(通常与他们被插入的
- 杀LINUX的JOB进程
bijian1013
linuxunix
今天发现数据库一个JOB一直在执行,都执行了好几个小时还在执行,所以想办法给删除掉
系统环境:
ORACLE 10G
Linux操作系统
操作步骤如下:
第一步.查询出来那个job在运行,找个对应的SID字段
select * from dba_jobs_running--找到job对应的sid
&n
- Spring AOP详解
bijian1013
javaspringAOP
最近项目中遇到了以下几点需求,仔细思考之后,觉得采用AOP来解决。一方面是为了以更加灵活的方式来解决问题,另一方面是借此机会深入学习Spring AOP相关的内容。例如,以下需求不用AOP肯定也能解决,至于是否牵强附会,仁者见仁智者见智。
1.对部分函数的调用进行日志记录,用于观察特定问题在运行过程中的函数调用
- [Gson六]Gson类型适配器(TypeAdapter)
bit1129
Adapter
TypeAdapter的使用动机
Gson在序列化和反序列化时,默认情况下,是按照POJO类的字段属性名和JSON串键进行一一映射匹配,然后把JSON串的键对应的值转换成POJO相同字段对应的值,反之亦然,在这个过程中有一个JSON串Key对应的Value和对象之间如何转换(序列化/反序列化)的问题。
以Date为例,在序列化和反序列化时,Gson默认使用java.
- 【spark八十七】给定Driver Program, 如何判断哪些代码在Driver运行,哪些代码在Worker上执行
bit1129
driver
Driver Program是用户编写的提交给Spark集群执行的application,它包含两部分
作为驱动: Driver与Master、Worker协作完成application进程的启动、DAG划分、计算任务封装、计算任务分发到各个计算节点(Worker)、计算资源的分配等。
计算逻辑本身,当计算任务在Worker执行时,执行计算逻辑完成application的计算任务
- nginx 经验总结
ronin47
nginx 总结
深感nginx的强大,只学了皮毛,把学下的记录。
获取Header 信息,一般是以$http_XX(XX是小写)
获取body,通过接口,再展开,根据K取V
获取uri,以$arg_XX
&n
- 轩辕互动-1.求三个整数中第二大的数2.整型数组的平衡点
bylijinnan
数组
import java.util.ArrayList;
import java.util.Arrays;
import java.util.List;
public class ExoWeb {
public static void main(String[] args) {
ExoWeb ew=new ExoWeb();
System.out.pri
- Netty源码学习-Java-NIO-Reactor
bylijinnan
java多线程netty
Netty里面采用了NIO-based Reactor Pattern
了解这个模式对学习Netty非常有帮助
参考以下两篇文章:
http://jeewanthad.blogspot.com/2013/02/reactor-pattern-explained-part-1.html
http://gee.cs.oswego.edu/dl/cpjslides/nio.pdf
- AOP通俗理解
cngolon
springAOP
1.我所知道的aop 初看aop,上来就是一大堆术语,而且还有个拉风的名字,面向切面编程,都说是OOP的一种有益补充等等。一下子让你不知所措,心想着:怪不得很多人都和 我说aop多难多难。当我看进去以后,我才发现:它就是一些java基础上的朴实无华的应用,包括ioc,包括许许多多这样的名词,都是万变不离其宗而 已。 2.为什么用aop&nb
- cursor variable 实例
ctrain
variable
create or replace procedure proc_test01
as
type emp_row is record(
empno emp.empno%type,
ename emp.ename%type,
job emp.job%type,
mgr emp.mgr%type,
hiberdate emp.hiredate%type,
sal emp.sal%t
- shell报bash: service: command not found解决方法
daizj
linuxshellservicejps
今天在执行一个脚本时,本来是想在脚本中启动hdfs和hive等程序,可以在执行到service hive-server start等启动服务的命令时会报错,最终解决方法记录一下:
脚本报错如下:
./olap_quick_intall.sh: line 57: service: command not found
./olap_quick_intall.sh: line 59
- 40个迹象表明你还是PHP菜鸟
dcj3sjt126com
设计模式PHP正则表达式oop
你是PHP菜鸟,如果你:1. 不会利用如phpDoc 这样的工具来恰当地注释你的代码2. 对优秀的集成开发环境如Zend Studio 或Eclipse PDT 视而不见3. 从未用过任何形式的版本控制系统,如Subclipse4. 不采用某种编码与命名标准 ,以及通用约定,不能在项目开发周期里贯彻落实5. 不使用统一开发方式6. 不转换(或)也不验证某些输入或SQL查询串(译注:参考PHP相关函
- Android逐帧动画的实现
dcj3sjt126com
android
一、代码实现:
private ImageView iv;
private AnimationDrawable ad;
@Override
protected void onCreate(Bundle savedInstanceState)
{
super.onCreate(savedInstanceState);
setContentView(R.layout
- java远程调用linux的命令或者脚本
eksliang
linuxganymed-ssh2
转载请出自出处:
http://eksliang.iteye.com/blog/2105862
Java通过SSH2协议执行远程Shell脚本(ganymed-ssh2-build210.jar)
使用步骤如下:
1.导包
官网下载:
http://www.ganymed.ethz.ch/ssh2/
ma
- adb端口被占用问题
gqdy365
adb
最近重新安装的电脑,配置了新环境,老是出现:
adb server is out of date. killing...
ADB server didn't ACK
* failed to start daemon *
百度了一下,说是端口被占用,我开个eclipse,然后打开cmd,就提示这个,很烦人。
一个比较彻底的解决办法就是修改
- ASP.NET使用FileUpload上传文件
hvt
.netC#hovertreeasp.netwebform
前台代码:
<asp:FileUpload ID="fuKeleyi" runat="server" />
<asp:Button ID="BtnUp" runat="server" onclick="BtnUp_Click" Text="上 传" />
- 代码之谜(四)- 浮点数(从惊讶到思考)
justjavac
浮点数精度代码之谜IEEE
在『代码之谜』系列的前几篇文章中,很多次出现了浮点数。 浮点数在很多编程语言中被称为简单数据类型,其实,浮点数比起那些复杂数据类型(比如字符串)来说, 一点都不简单。
单单是说明 IEEE浮点数 就可以写一本书了,我将用几篇博文来简单的说说我所理解的浮点数,算是抛砖引玉吧。 一次面试
记得多年前我招聘 Java 程序员时的一次关于浮点数、二分法、编码的面试, 多年以后,他已经称为了一名很出色的
- 数据结构随记_1
lx.asymmetric
数据结构笔记
第一章
1.数据结构包括数据的
逻辑结构、数据的物理/存储结构和数据的逻辑关系这三个方面的内容。 2.数据的存储结构可用四种基本的存储方法表示,它们分别是
顺序存储、链式存储 、索引存储 和 散列存储。 3.数据运算最常用的有五种,分别是
查找/检索、排序、插入、删除、修改。 4.算法主要有以下五个特性:
输入、输出、可行性、确定性和有穷性。 5.算法分析的
- linux的会话和进程组
网络接口
linux
会话: 一个或多个进程组。起于用户登录,终止于用户退出。此期间所有进程都属于这个会话期。会话首进程:调用setsid创建会话的进程1.规定组长进程不能调用setsid,因为调用setsid后,调用进程会成为新的进程组的组长进程.如何保证? 先调用fork,然后终止父进程,此时由于子进程的进程组ID为父进程的进程组ID,而子进程的ID是重新分配的,所以保证子进程不会是进程组长,从而子进程可以调用se
- 二维数组 元素的连续求解
1140566087
二维数组ACM
import java.util.HashMap;
public class Title {
public static void main(String[] args){
f();
}
// 二位数组的应用
//12、二维数组中,哪一行或哪一列的连续存放的0的个数最多,是几个0。注意,是“连续”。
public static void f(){
- 也谈什么时候Java比C++快
windshome
javaC++
刚打开iteye就看到这个标题“Java什么时候比C++快”,觉得很好笑。
你要比,就比同等水平的基础上的相比,笨蛋写得C代码和C++代码,去和高手写的Java代码比效率,有什么意义呢?
我是写密码算法的,深刻知道算法C和C++实现和Java实现之间的效率差,甚至也比对过C代码和汇编代码的效率差,计算机是个死的东西,再怎么优化,Java也就是和C