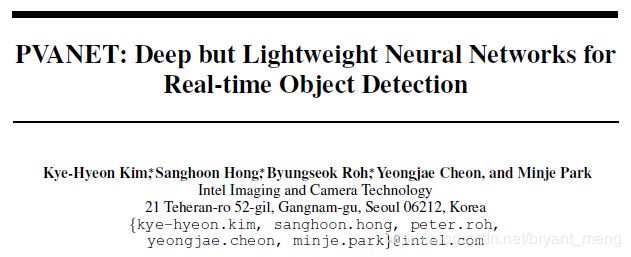
arXiv preprint arXiv:1608.08021, 2016.
caffe code :https://github.com/sanghoon/pva-faster-rcnn/blob/master/models/pvanet/example_train/train.prototxt
caffe code 可视化工具:http://ethereon.github.io/netscope/#/editor
文章目录
- 1 Background and Motivation
- 2 Advantages / Contributions
- 3 Innovations
- 4 Method
- 4.1 C.ReLU: Earlier building blocks in feature generation
- 4.2 Inception: Remaining building blocks in feature generation
- 4.3 HyperNet: Concatenation of multi-scale intermediate outputs
- 4.4 Deep network training
- 5 Experiments
- 5.1 Datasets and Training
- 5.2 VOC 2007
- 5.3 VOC 2012
- 6 Conclusion(Own)
1 Background and Motivation
目前目标检测精度还不错,automotive and surveillance 领域有广泛的商业市场,但是速度堪忧,作者从提升速度这个点出发,重新设计了 backbone,遵循 less channels with more layers 的设计准则,在 VOC 07 和 12 上取得了相当不错的结果,且大幅度的降低了 computational cost,做到 Real-time.
2 Advantages / Contributions
- 83.8% mAP on VOC 2007
- 82.5% mAP on VOC 2012(2nd place,计算量只有第一名 resnet 的 12.3%)
- 46 ms/image on Titan X((21.7FPS))
lightweight feature extraction network
3 Innovations
- 自己设计了整个目标检测网络,light weight 且 精度在线
- 大大提升速度,做到 real time
4 Method
4.1 C.ReLU: Earlier building blocks in feature generation
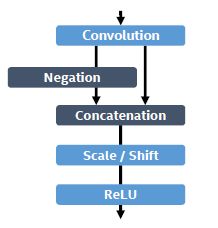
C 为 concatenation 的意思,不同于 original C.ReLU(来源于 《Understanding and Improving Convolutional Neural Networks via Concatenated Rectified Linear Units》),作者增加了 Scale / Shift 操作,同 Batch normalization 的复原操作,对每个通道进行!这种设计的 motivation 是 In the early stage, output nodes tend to be “paired” such that one node’s activation is the opposite side of another’s. 所以可以把 channels 减半,正负 concatenate 即可,精度相仿!2x speed-up
4.2 Inception: Remaining building blocks in feature generation
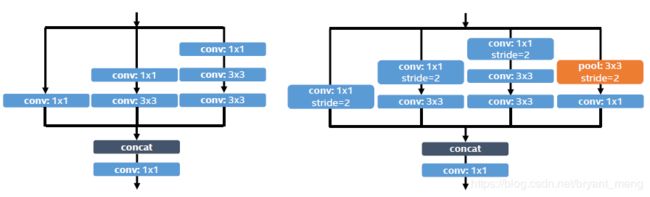
作者的 inception 堆叠形式相对于原版的 GoogleNet,少了pooling 的分支,5x5 替换成了 double 3x3,这中形式能很好的捕捉不同尺寸的目标,作者用如下的图进行了解释
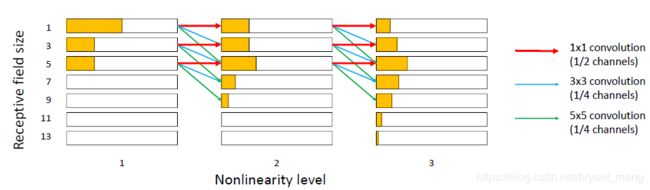
哈哈哈,这个第一眼看会有点懵,但是没关系,经历过大风大浪,看这16年的前人工作,首先心理上不能惧怕!仔细分析,原来如此!
上图描述的就是三个 inception block 堆叠的情况,第一层 1,3,5 的感受野 channels 分别为原来的 ( 1 2 , 1 4 , 1 4 ) (\frac{1}{2},\frac{1}{4},\frac{1}{4}) (21,41,41),两层堆叠后,也即 ( 1 2 , 1 4 , 1 4 ) ∗ ( 1 2 , 1 4 , 1 4 ) (\frac{1}{2},\frac{1}{4},\frac{1}{4})*(\frac{1}{2},\frac{1}{4},\frac{1}{4}) (21,41,41)∗(21,41,41),注意感受野的乘法准则即可, 1 ∗ x = x , 3 ∗ 3 = 5 , 3 ∗ 5 = 7 1*x=x,3*3=5, 3*5=7 1∗x=x,3∗3=5,3∗5=7 以此类推,相邻的奇数相乘等于他们下一个奇数!
我们来算下 ( 1 2 , 1 4 , 1 4 ) ∗ ( 1 2 , 1 4 , 1 4 ) (\frac{1}{2},\frac{1}{4},\frac{1}{4})*(\frac{1}{2},\frac{1}{4},\frac{1}{4}) (21,41,41)∗(21,41,41) 的结果,也即第二层的结果,也即感受野 ( 1 , 3 , 5 ) ∗ ( 1 , 3 , 5 ) (1,3,5)*(1,3,5) (1,3,5)∗(1,3,5) 的结果
- 感受野 1:仅 1 ∗ 1 1*1 1∗1,也即 1 2 ∗ 1 2 = 1 4 \frac{1}{2}*\frac{1}{2} = \frac{1}{4} 21∗21=41
- 感受野 3:有 1 ∗ 3 1*3 1∗3 和 3 ∗ 1 3*1 3∗1,也即 1 2 ∗ 1 4 + 1 4 ∗ 1 2 = 1 4 \frac{1}{2}*\frac{1}{4} + \frac{1}{4}*\frac{1}{2} = \frac{1}{4} 21∗41+41∗21=41
- 感受野 5:有 1 ∗ 5 1*5 1∗5 、 5 ∗ 1 5*1 5∗1 和 3 ∗ 3 3*3 3∗3,也即 1 2 ∗ 1 4 + 1 4 ∗ 1 2 + 1 4 ∗ 1 4 = 5 16 \frac{1}{2}*\frac{1}{4} + \frac{1}{4}*\frac{1}{2} + \frac{1}{4}*\frac{1}{4} = \frac{5}{16} 21∗41+41∗21+41∗41=165
- 感受野 7:有 3 ∗ 5 3*5 3∗5 和 5 ∗ 3 5*3 5∗3,也即 1 4 ∗ 1 4 + 1 4 ∗ 1 4 = 1 8 \frac{1}{4}*\frac{1}{4} + \frac{1}{4}*\frac{1}{4} = \frac{1}{8} 41∗41+41∗41=81
- 感受野 9:仅 5 ∗ 5 5*5 5∗5,也即 1 4 ∗ 1 4 = 1 16 \frac{1}{4}*\frac{1}{4} = \frac{1}{16} 41∗41=161
第三层的计算就是 ( 1 4 , 1 4 , 5 16 , 1 8 , 1 16 ) ∗ ( 1 2 , 1 4 , 1 4 ) (\frac{1}{4},\frac{1}{4},\frac{5}{16}, \frac{1}{8}, \frac{1}{16})*(\frac{1}{2},\frac{1}{4},\frac{1}{4}) (41,41,165,81,161)∗(21,41,41),也即 ( 1 , 3 , 5 , 7 , 9 ) ∗ ( 1 , 3 , 5 ) (1,3,5,7,9)*(1,3,5) (1,3,5,7,9)∗(1,3,5),用感受野的 “乘法公式”,对应通道的比重相乘即可!
it slows down the growth of receptive fields for some output features so that small-sized objects can be captured precisely.
4.3 HyperNet: Concatenation of multi-scale intermediate outputs
x × x x×x x×x C.ReLU 表示 1 × 1 → x × x → 1 × 1 1×1→x×x→1×1 1×1→x×x→1×1 模式,其中 x × x x×x x×x 的形式如下图所示
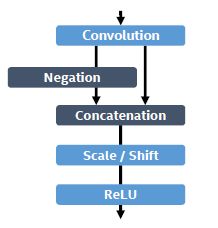
- inception 中的 # out 表示 concatenation 之后的 1 ∗ 1 1*1 1∗1
- resnet 结构中, 1 ∗ 1 1*1 1∗1 的 short cut 用在 stride = 2 和 channels 改变的时候!
- Multi-scale features 的做法如下:conv3_4 downscale(128)、conv4_4(256)、conv5_4(384) upscale concatenation
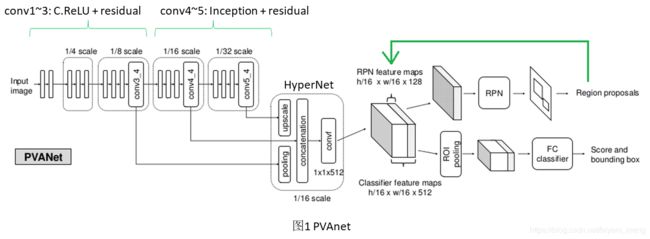
图片来自于 [目标检测]PVAnet原理,简单明了
- RPN 取 convf 的前 128 channels,配合 3 × 3 3×3 3×3 conv (384 channels) 和 1 × 1 1×1 1×1 conv (25x(2+4) = 150 channel),5 scale 和 5 ratio (3, 6, 9, 16, 25),(0.5, 0.667, 1.0, 1.5,2.0). 2 是 2 分类,4 是 bbox delta
- head,after roi pooling 6 ∗ 6 ∗ 512 6*6*512 6∗6∗512, 4096 4096 4096(fc), 4096 4096 4096(fc), 21 21 21(20+1类), 84 84 84(21*4 bbox delta)
4.4 Deep network training
- Batch Normalization
- moving average of loss(keras 有实现,哈哈,这里不再赘述)
- inception + residual connection(注意,作者在 inception block concatenation 之后,接了 1 ∗ 1 1*1 1∗1,residual connection 或者 x,或者 c o n v 1 ∗ 1 conv 1*1 conv1∗1,把 inception 1*1 后的结果和 residual connection 相加)
5 Experiments
5.1 Datasets and Training
ILSVRC2012、MS COCO、PASCAL VOC 2007、2012
- 预训练:ILSVRC2012
- 然后:MS COCO、PASCAL VOC 2007、2012 trainval 训练
- fine-tuning:PASCAL VOC 2007、2012 trainval
5.2 VOC 2007
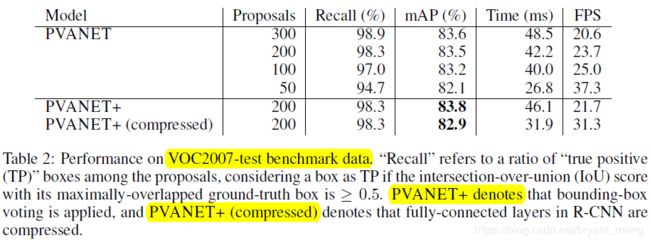
5.3 VOC 2012
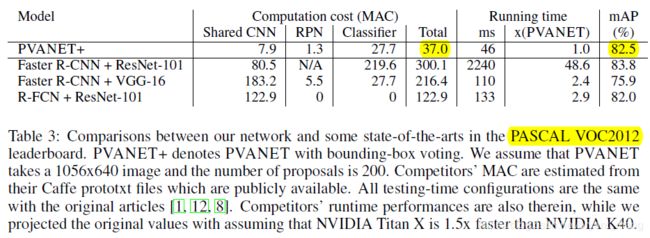
MAC(number of adds and multiplications) 很夸张,mAP 和 state-of-art 相仿,2nd place,还顺带说了下 1st 用了一些 trick,比如多尺度测试!!!
6 Conclusion(Own)
C.ReLU 还是给人很大的启发,up sampling 竟然用的 4 ∗ 4 4*4 4∗4 conv,不过话说好像和 kernel size 无关,这个以后有空得琢磨下!设计网络的思路给人启发!!!