看了几篇关于cnn的文章,感觉那种大模型的cnn真的不适合个人去使用,自己也没有那么强悍的显卡,也没有足够的数据和时间
还是用迁移学习比较好,这里说一下用的模型,inception_v3是谷歌的cnn框架。这个框架有22层深,用tensorboard看的时候是比较大的(相比于letnet和alxnet),这个框架运算量并不大,而且很多卷积层的权值基本上可以不用改变,可以说使用起来非常的方便。
他降低参数有两点 第一是去除了最后的全连接层,采用全局平均池化层(将图片尺寸变为1*1)来取代它。全连接层基本上占据了alxnet和vggnet 90%的参数量,为什么呢?因为卷积核并不多,而三层全连接层(Alxnet)的参数量是非常恐怖的,第一层就以万计。而且参数过多,数据量少的话会过拟合,效果并不好。
第二是Inception V1中精心设计Inception moudle级高了参数的利用率,这个结构的思路借鉴于VGGnet,VGGnet首次实现了多个小卷积核的同时使用,替换了Alxnet的第一层11*11的卷积核,而Inception的卷积核尺寸更小,参数利用率越高
下面我来说一下怎么使用,主要是参考讲座 炼数成金,但是对这个里面的bug进行了修改。
首先,下载数据集合,数据集我用flowers的,事实上后来我才发现,官方提供了直接针对flowes的代码。
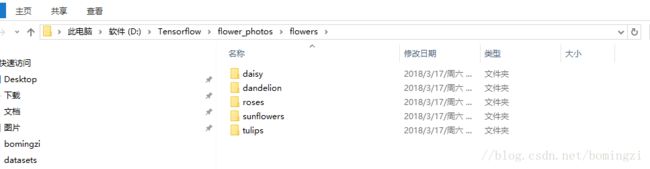
这里面的是花的5个种类
这里有一个txt文件,是output_labels.txt是所有花的名称,放在flower_photo目录下
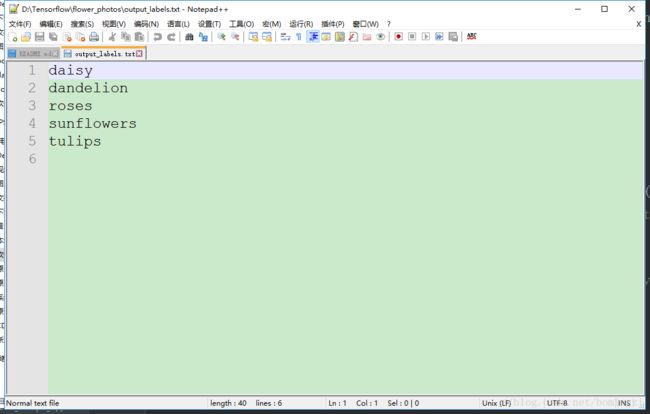
然后生成tfrecord文件
先上代码再解释吧
-
-
- import tensorflow as tf
- import os
- import random
- import math
- import sys
- import types
- from PIL import Image
-
-
- _NUM_TEST = 300
-
- _RANDOM_SEED = 0
-
- _NUM_SHARDS = 5
-
- DATASET_DIR = 'D:/Tensorflow/flower_photos/flowers'
-
- LABELS_FILENAME = 'D:/Tensorflow/flower_photos/output_labels.txt'
-
-
- def _get_dataset_filename(dataset_dir,split_name,shard_id):
- output_filename = 'image_%s_%05d-of-%05d.tfrecord' % (split_name,shard_id,_NUM_SHARDS)
- return os.path.join(dataset_dir,output_filename)
-
-
- def _datase_exists(dataset_dir):
- for split_name in ['train','test']:
- for shard_id in range(_NUM_SHARDS):
-
- output_filename = _get_dataset_filename(dataset_dir,split_name,shard_id)
- if not tf.gfile.Exists(output_filename):
- return False
- return True
-
-
-
- def _get_filenames_and_classes(dataset_dir):
-
- directories = []
-
- class_names = []
- for filename in os.listdir(dataset_dir):
-
- path = os.path.join(dataset_dir,filename)
-
- if os.path.isdir(path):
-
- directories.append(path)
-
- class_names.append(filename)
- photo_filenames = []
-
- for directory in directories:
- for filename in os.listdir(directory):
- path = os.path.join(directory,filename)
-
- photo_filenames.append(path)
- return photo_filenames,class_names
-
- def int64_feature(values):
- if not isinstance(values,(tuple,list)):
- values = [values]
-
- return tf.train.Feature(int64_list=tf.train.Int64List(value=values))
-
- def bytes_feature(values):
- return tf.train.Feature(bytes_list=tf.train.BytesList(value=[values]))
-
-
- def image_to_tfexample(image_data,image_format,class_id):
- return tf.train.Example(features=tf.train.Features(feature={
- 'image/encoded': bytes_feature(image_data),
- 'image/format' : bytes_feature(image_format),
- 'image/class/label' : int64_feature(class_id)
- }))
-
-
- def write_label_file(labels_to_class_names,dataset_dir,filename='label.txt'):
-
- labels_file_name = os.path.join(dataset_dir,filename)
- print(dataset_dir)
-
- with tf.gfile.Open(labels_file_name,'w') as f:
- for label in labels_to_class_names:
- class_name = labels_to_class_names[label]
- f.write('%d;%s\n'%(label,class_name))
-
-
-
- def _convert_dataset(split_name,filenames,class_names_to_ids,dataset_dir):
-
- assert split_name in ['train','test']
-
- num_per_shard = int(len(filenames) / _NUM_SHARDS)
- with tf.Graph().as_default():
- with tf.Session() as sess:
- for shard_id in range(_NUM_SHARDS):
-
- output_filename = _get_dataset_filename(dataset_dir,split_name,shard_id)
- with tf.python_io.TFRecordWriter(output_filename) as tfrecore_writer:
-
- start_ndx = shard_id * num_per_shard
-
- end_ndx = min((shard_id+1) * num_per_shard,len(filenames))
-
- for i in range(start_ndx,end_ndx):
- try:
- sys.stdout.write('\r>> Converting image %d/%d shard %d' % (i+1,len(filenames),shard_id))
- sys.stdout.flush()
-
-
- img = Image.open(filenames[i])
-
- img_raw = img.tobytes()
-
- class_name = os.path.basename(os.path.dirname(filenames[i]))
-
- class_id = class_names_to_ids[class_name]
-
- example = image_to_tfexample(img_raw, b'jpg',class_id)
-
- tfrecore_writer.write(example.SerializeToString())
- except IOError as e:
- print("Could not read: ",filenames[i])
- print("Error: ",e)
- print("Skip it \n")
-
- sys.stdout.write('\n')
- sys.stdout.flush()
-
-
- if __name__=='__main__':
-
- if _datase_exists(DATASET_DIR):
- print('tfrecord 文件已经存在')
- else :
-
- photo_filenames,class_names = _get_filenames_and_classes(DATASET_DIR)
-
-
- class_names_to_ids = dict(zip(class_names,range(len(class_names))))
- print(class_names_to_ids)
-
- random.seed(_RANDOM_SEED)
- random.shuffle(photo_filenames)
- training_filenames = photo_filenames[_NUM_TEST:]
- testing_filenames = photo_filenames[:_NUM_TEST]
-
-
- _convert_dataset('train',training_filenames,class_names_to_ids,DATASET_DIR)
- _convert_dataset('test',testing_filenames,class_names_to_ids,DATASET_DIR)
-
-
- labels_to_class_names = dict(zip(range(len(class_names)),class_names))
- write_label_file(labels_to_class_names,DATASET_DIR)
思路很简单,就是读取图片然后分割,最后转换成tfrecord格式的文件,说一下需要修改的地方(我说了就不用自己找了。。。。)

这两个都是刚才说到的,一个是图片存放的位置,一个是标签文件,为了生成一个类似于字典的txt,其他的不用改,如果
你想改
这里的名字的话,那么你后面读取的时候要改官方给你的py文件,还是省省吧。
默认会在你的图片的目录下生成tfrecord文件和labels标签,
为了好看,我把他们移出来,单独放一个文件夹。
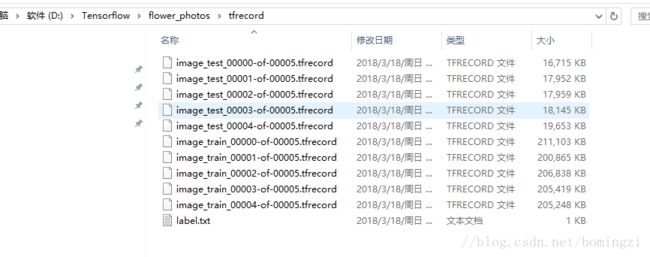
然后我们要特别看一下官方给你的几个py文件,如果你只用官方给的例子像测试下的话可以跳过。
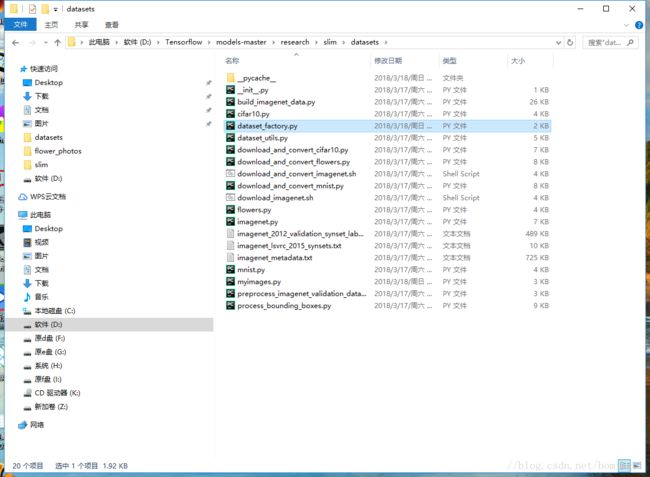
首先是这个dataset_factory 这个要改,

原来是没有这个的,你要加上这个,datasets是你所在的这个目录,myimages自然就是你要自己写的py文件了
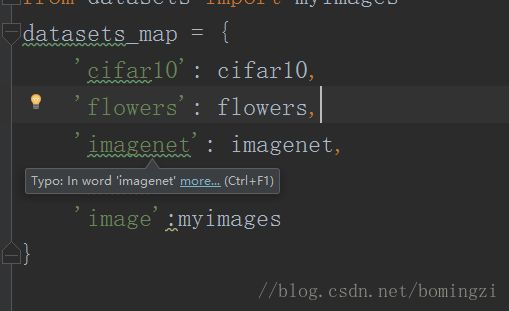
这里新加上最后一个字典,'image'只是个名字或者叫标识,myimages是你的py文件
然后我们来看看我们自己写的myimages
由于我用的是flowes的图片,你会发现官方给了你一个flowers.py所以你可以参考这个写一下。
下面上一下我的myimages文件,
- from __future__ import absolute_import
- from __future__ import division
- from __future__ import print_function
-
- import os
- import tensorflow as tf
-
- from datasets import dataset_utils
-
- slim = tf.contrib.slim
-
- _FILE_PATTERN = 'image_%s_*.tfrecord'
-
- SPLITS_TO_SIZES = {'train': 3320, 'validation': 350}
-
- _NUM_CLASSES = 5
-
- _ITEMS_TO_DESCRIPTIONS = {
- 'image': 'A color image of varying size.',
- 'label': 'A single integer between 0 and 4',
- }
-
-
- def get_split(split_name, dataset_dir, file_pattern=None, reader=None):
-
- if split_name not in SPLITS_TO_SIZES:
- raise ValueError('split name %s was not recognized.' % split_name)
-
- if not file_pattern:
- file_pattern = _FILE_PATTERN
- file_pattern = os.path.join(dataset_dir, file_pattern % split_name)
-
- if reader is None:
- reader = tf.TFRecordReader
-
- keys_to_features = {
- 'image/encoded': tf.FixedLenFeature((), tf.string, default_value=''),
- 'image/format': tf.FixedLenFeature((), tf.string, default_value='png'),
- 'image/class/label': tf.FixedLenFeature(
- [], tf.int64, default_value=tf.zeros([], dtype=tf.int64)),
- }
-
- items_to_handlers = {
- 'image': slim.tfexample_decoder.Image(),
- 'label': slim.tfexample_decoder.Tensor('image/class/label'),
- }
-
- decoder = slim.tfexample_decoder.TFExampleDecoder(
- keys_to_features, items_to_handlers)
-
- labels_to_names = None
- if dataset_utils.has_labels(dataset_dir):
- labels_to_names = dataset_utils.read_label_file(dataset_dir)
-
- return slim.dataset.Dataset(
- data_sources=file_pattern,
- reader=reader,
- decoder=decoder,
- num_samples=SPLITS_TO_SIZES[split_name],
- items_to_descriptions=_ITEMS_TO_DESCRIPTIONS,
- num_classes=_NUM_CLASSES,
- labels_to_names=labels_to_names)
- 你会发现这里,这个可前面生成tfrecord的名字是有对应关系的。
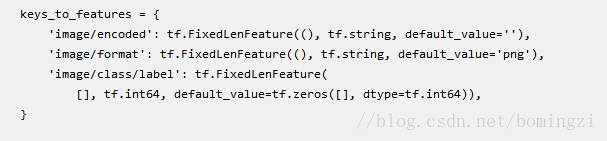
这个文件大致意思就是读取下tfrecord文件,然后分割下,有的用来train,有的用来test
接下来可以进行train了
train.bat写在slim这个文件夹下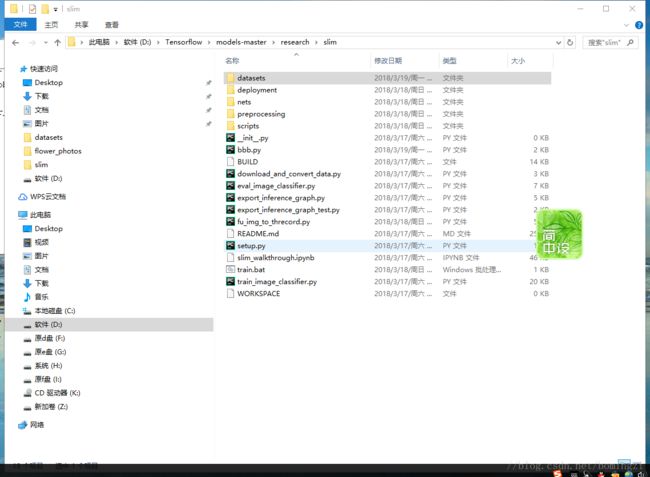
这里我附上我的train然后讲解下参数
- python train_image_classifier.py ^
- --train_dir=D:/Tensorflow/flower_photos/train ^
- --dataset_name=image ^
- --dataset_split_name=train ^
- --dataset_dir=D:/Tensorflow/flower_photos/flowers/tfrecord ^
- --batch_size=5 ^
- --max_number_of_steps=10000 ^
- --model_name=inception_v3 ^
- --clone_on_cpu=true ^
- pause
第一个是你的train_iamge_classifier的位置,这里用的是相对位置
第二个是新建的空文件夹,训练完的数据会放到这个文件夹下
第三个特点的,你在生成tfrecord 的时候切分数据的train和test中的train
第四个是你的tfrecord文件的位置,里面必须要有labels.txt
第五个是分批训练的,主要用于显存不够,不能够一次性存放足够多的数据
第六个是训练的次数,不设置的情况下会一直执行
第七个是训练的模型 这里使用inception_v3模型
第八个很重要,我之前一直报错,问了好多人,上了各种网站都没查出来,这个应该是有些cpu版本的tensorflow才能处理的数据,在GPU上无法计算,所以要开启能够使用cpu的这个选项,如果是cpu版本的tensorflow应该没有问题。
第九个 pause 好像没什么用,改退出还是会退出,所以还是从命令窗口开始执行吧。
训练完之后在你的train文件夹下会生成数据
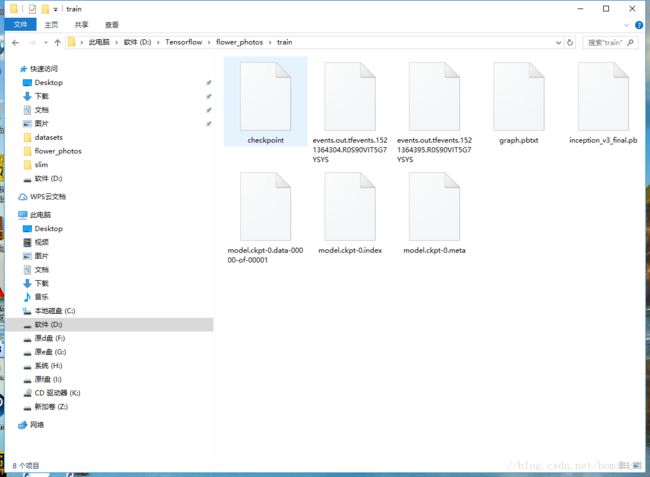
然后在slim目录下新建一个bbb.py
- import os
- import tensorflow as tf
- import tensorflow.contrib.slim as slim
-
- from nets import inception
- from nets import inception_v1
- from nets import inception_v3
- from nets import nets_factory
-
- from tensorflow.python.framework import graph_util
- from tensorflow.python.platform import gfile
- from google.protobuf import text_format
-
- checkpoint_path = tf.train.latest_checkpoint('D:/Tensorflow/flower_photos/train')
- with tf.Graph().as_default() as graph:
- input_tensor = tf.placeholder(tf.float32, shape=(None, 299, 299, 3), name='input_image')
- with tf.Session() as sess:
-
- with slim.arg_scope(inception.inception_v3_arg_scope()):
- logits, end_points = inception.inception_v3(input_tensor, num_classes=5, is_training=False)
-
- saver = tf.train.Saver()
- saver.restore(sess, checkpoint_path)
-
- output_node_names = 'InceptionV3/Predictions/Reshape_1'
-
- input_graph_def = graph.as_graph_def()
- output_graph_def = graph_util.convert_variables_to_constants(sess, input_graph_def, output_node_names.split(","))
- with open('D:/Tensorflow/flower_photos/output_graph_nodes.txt', 'w') as f:
- f.write(text_format.MessageToString(output_graph_def))
-
- output_graph = 'D:/Tensorflow/flower_photos/train/inception_v3_final.pb'
- with gfile.FastGFile(output_graph, 'wb') as f:
- f.write(output_graph_def.SerializeToString())
执行后会在train目录下生成pb文件,这个是tensorflow保存和读取的模型文件。
然后我们来使用他来识别。
用到的命令整理:
rm -rf /home/leo/Downloads/tmp/train_dir/*
python train_image_classifier.py \
--train_dir=/home/leo/Downloads/tmp/train_dir \
--dataset_name=dish \
--dataset_split_name=train \
--dataset_dir=/home/leo/Downloads/train_datas/smallDataSetTest5_9/output_tfrecord \
--model_name=inception_resnet_v2 \
--max_number_of_steps=100000 \
--batch_size=6 \
--learning_rate=0.0001 \
--learning_rate_decay_type=fixed \
--save_interval_secs=60 \
--save_summaries_secs=60 \
--log_every_n_steps=10 \
--optimizer=rmsprop \
--weight_decay=0.00004
不fine-tune把--checkpoint_path, --checkpoint_exclude_scopes和--trainable_scopes删掉。
fine-tune所有层把--checkpoint_exclude_scopes和--trainable_scopes删掉。
如果只使用CPU则加上--clone_on_cpu=True。
验证checkpoint:
python eval_image_classifier.py \
--checkpoint_path=/home/leo/Downloads/tmp/train_dir \
--eval_dir=/home/leo/Downloads/tmp/eval_logs \
--dataset_name=dish \
--dataset_split_name=validation \
--dataset_dir=/home/leo/Downloads/train_datas/smallDataSetTest5_9/output_tfrecord \
--model_name=inception_resnet_v2
其他常用训练命令:
############################################################
使用train image classifier 训练 inception_resnet_v2 using fine-tune
############################################################
python train_image_classifier.py \
--train_dir=/home/leo/Downloads/tmp/train_dir_220_dish_inception_resnet_v2 \
--dataset_dir=/home/leo/Downloads/train_datas/18_5_14_220_tfrecord/output_tfrecord \
--dataset_name=dish \
--dataset_split_name=train \
--model_name=inception_resnet_v2 \
--checkpoint_path=/home/leo/Downloads/pretrained_models/inception_resnet_v2_2016_08_30/inception_resnet_v2_2016_08_30.ckpt \
--checkpoint_exclude_scopes=InceptionResnetV2/Logits,InceptionResnetV2/AuxLogits \
--trainable_scopes=InceptionResnetV2/Logits,InceptionResnetV2/AuxLogits
############################################################
使用train image classifier 训练 mobile net v1 using fine-tune
############################################################
python train_image_classifier.py \
--train_dir=/home/leo/Downloads/tmp/train_dir_220_dish_mobilenet_v1 \
--dataset_dir=/home/leo/Downloads/train_datas/18_5_14_220_tfrecord/output_tfrecord \
--dataset_name=dish \
--dataset_split_name=train \
--model_name=mobilenet_v1 \
--checkpoint_path=/home/leo/Downloads/pretrained_models/mobilenet_v1_1.0_224/mobilenet_v1_1.0_224.ckpt \
--checkpoint_exclude_scopes=MobilenetV1/Logits \
--trainable_scopes=MobilenetV1/Logits
############################################################
使用train image classifier 训练 mobile net v2 from scratch
############################################################
python train_image_classifier.py \
--train_dir=/home/leo/Downloads/tmp/train_dir_220_dish_mobilenet_v2 \
--dataset_dir=/home/leo/Downloads/train_datas/18_5_14_220_tfrecord/output_tfrecord \
--dataset_name=dish \
--dataset_split_name=train \
--model_name=mobilenet_v2 \
--train_image_size=224 \
--learning_rate=0.0001 \
--learning_rate_decay_type=fixed \