import torch
import torchtext
import numpy as np
import torch.nn.functional as F
from torchtext.vocab import GloVe
import torch.nn as nn
import time
start=time.time()
#定义样本处理操作
#小写 截取评论长度为200(少了填充、多了截取) 第一维度是batch
TEXT = torchtext.data.Field(lower=True,fix_length=200,batch_first=True)
#sequential表示是否顺序
LABEL = torchtext.data.Field(sequential=False)
#下载torchtext内置的IMDB电影评论数据集并切分训练集和测试集
#Field知道如何处理原始数据,用户告诉Field去哪里处理
train,test = torchtext.datasets.IMDB.splits(TEXT,LABEL)
#创建词表
#训练集、出现次数最多的10000个单词创建词表、若单词出现次数少于3则抛弃
TEXT.build_vocab(train,max_size=10000,min_freq=3)
LABEL.build_vocab(train)
#生成器
train_iter, test_iter = torchtext.data.BucketIterator.splits((train,test),batch_size=16)
device = torch.device("cuda:0" if torch.cuda.is_available() else "cpu")
#创建模型
class Net(nn.Module):
def __init__(self):
super(Net, self).__init__()
#nn.Embedding()类似于nn.Conv2d()
#有len(TEXT.vocab.stoi)个单词需要做映射
#用一个长度为100的张量来表示每一个单词
#batch*200 --> batch*200*100
self.em = nn.Embedding(len(TEXT.vocab.stoi),100)
self.fc1 = nn.Linear(200*100,1024)
self.fc2 = nn.Linear(1024,3)
def forward(self,x):
x = self.em(x)
#扁平化
x = x.view(x.size(0),-1)
x = F.relu(self.fc1(x))
x = self.fc2(x)
return x
model = Net()
model = model.to(device)
loss_fn = torch.nn.CrossEntropyLoss()
optim = torch.optim.Adam(model.parameters(),lr=0.0001)
epochs = 10
def fit(epoch,model,trainloader,testloder):
correct = 0
total = 0
running_loss = 0
model.train()
#注意这里与前几次图片分类的不一样
#返回的是一个批次成对数据
for b in trainloader:
x, y = b.text, b.label
x = x.to(device)
y = y.to(device)
y_pred = model(x)
loss = loss_fn(y_pred, y)
optim.zero_grad()
loss.backward()
optim.step()
with torch.no_grad():
y_pred = torch.argmax(y_pred, dim=1)
correct += (y_pred == y).sum().item()
total += y.size(0)
running_loss += loss.item()
epoch_loss = running_loss/len(trainloader.dataset)
epoch_acc = correct/total
test_correct = 0
test_total = 0
test_running_loss = 0
model.eval()
with torch.no_grad():
#这里也是同样变化
for b in testloder:
x,y = b.text,b.label
x = x.to(device)
y = y.to(device)
y_pred = model(x)
loss = loss_fn(y_pred,y)
y_pred = torch.argmax(y_pred, dim=1)
test_correct += (y_pred == y).sum().item()
test_total += y.size(0)
test_running_loss += loss.item()
epoch_test_loss = test_running_loss / len(testloder.dataset)
epoch_test_acc = test_correct / test_total
print('epoch: ',epoch,
'train_loss: ',round(epoch_loss,3),
'train_accuracy: ',round(epoch_acc,3),
'test_loss: ',round(epoch_test_loss,3),
'test_accuracy: ',round(epoch_test_acc,3)
)
return epoch_loss,epoch_acc,epoch_test_loss,epoch_test_acc
train_loss=[]
train_acc=[]
test_loss=[]
test_acc=[]
for epoch in range(epochs):
epoch_loss,epoch_acc,epoch_test_loss,epoch_test_acc = fit(epoch,model,train_iter,test_iter)
train_loss.append(epoch_loss)
train_acc.append(epoch_acc)
test_loss.append(epoch_test_loss)
test_acc.append(epoch_test_acc)
end = time.time()
print(end-start)
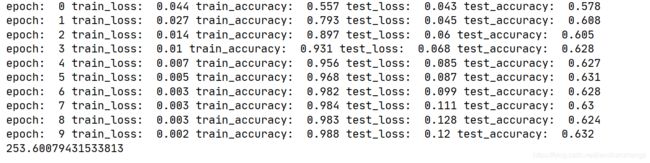
模型出现了过拟合问题